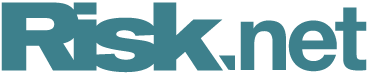
The Catch-22 of US banks’ liquidity buffers
US banks are using held-to-maturity bonds to underpin liquidity adequacy, grating against accounting guidance. What happens if they’re forced to sell?
The job of a financial regulator can often feel like fixing a leaky boat: just when you’ve plugged one hole, the water starts gushing in from somewhere else.
In recent years, regulators had focused their efforts on making sure banks weren’t underestimating the risk in their loan books. But the liquidity panic that engulfed Silicon Valley Bank, Signature Bank and Credit Suisse spouted, in a way, from the opposite end of the vessel, as customers redeemed deposits faster than the banks could liquidate assets.
It’s a weakness global standard-setters thought they’d pre-empted with the liquidity coverage ratio, one of the planks of the Basel III bank-soundness reforms. The measure requires deposit institutions to hold enough high-quality liquid assets (HQLAs) to cover 30 days of stressed cash outflows, as simulated by weighting and stressing liabilities.
While a big chunk of HQLAs consists of cash parked at a central bank, securities that lenders can liquidate at short notice and with minimal friction – like US Treasuries and a handful of such safe-haven bonds – also qualify. Such securities must of course be marked-to-market, to make sure their book valuation aligns with their cash-raising potential.
It would be logical for banks to tag such instruments as available-for-sale (AFS) – non-trading assets that the bank may sell before principal is repaid, and which it thus retains at fair value. Yet disclosures by US banks subject to the liquidity coverage ratio suggest at least part of their HQLAs are booked as held-to-maturity (HTM) – a holding pen, as the name implies, intended for bond investments that will stay with the bank until they mature. These are kept on the balance sheet at – broadly – acquisition cost, rather than their current market worth.
No explicit rule forbids banks from booking HQLAs as HTM, and HTM securities do get measured at fair value when included within HQLAs. Guidance from the Financial Accounting Standards Board and the Office of the Comptroller of the Currency, however, comes pretty close to a prohibition.
Selling an asset from the HTM book can “taint” the remainder of the bank’s investments, the standards say, forcing all securities into AFS and disqualifying the bank from HTM use until regulators are satisfied it won’t happen again.
There are remote circumstances – “safe harbours”, as FASB calls them – in which an HTM-to-AFS move may be warranted. These include balance sheet adjustments after a merger or disposal, fiscal or regulatory overhauls that fundamentally shift the HTM book’s risk profile, a downgrade of the issuer’s credit rating – but not a liquidity emergency.
Indeed, the OCC explicitly posits the example of a bank selling HTM securities “to gain additional liquidity”, and concludes this would fall outside the exceptional circumstances delineated by FASB, tainting the portfolio.
The FASB standards do provide for a further, narrow exception for HTM sales, in response to an event that is “isolated, non-recurring, unusual [and] that could not have been reasonably anticipated” by the reporting entity. While FASB, somewhat in passing, suggests a bank run as a potential such event, the OCC’s chosen example of a category 4 hurricane hints that nothing less than an act of God would qualify – and certainly not endogenous systemic panic.
Banks’ legal counsels have likely already pored over the OCC and FASB guidance to make sure the way they book HQLAs is scrutiny-proof. But as rulemakers in Washington consider forcing mid-to-small lenders to re-adopt the liquidity coverage ratio, some new complementary guidance around accounting may be in order if Basel III’s liquidity backstop is to work as intended.
Only users who have a paid subscription or are part of a corporate subscription are able to print or copy content.
To access these options, along with all other subscription benefits, please contact info@risk.net or view our subscription options here: http://subscriptions.risk.net/subscribe
You are currently unable to print this content. Please contact info@risk.net to find out more.
You are currently unable to copy this content. Please contact info@risk.net to find out more.
Copyright Infopro Digital Limited. All rights reserved.
As outlined in our terms and conditions, https://www.infopro-digital.com/terms-and-conditions/subscriptions/ (point 2.4), printing is limited to a single copy.
If you would like to purchase additional rights please email info@risk.net
Copyright Infopro Digital Limited. All rights reserved.
You may share this content using our article tools. As outlined in our terms and conditions, https://www.infopro-digital.com/terms-and-conditions/subscriptions/ (clause 2.4), an Authorised User may only make one copy of the materials for their own personal use. You must also comply with the restrictions in clause 2.5.
If you would like to purchase additional rights please email info@risk.net
More on Our take
Lots to fear, including fear itself
Binary scenarios for key investment risks in this year’s Top 10 are worrying buy-siders
Podcast: Alexei Kondratyev on quantum computing
Imperial College London professor updates expectations for future tech
Quants mine gold for new market-making model
Novel approach to modelling cointegrated assets could be applied to FX and potentially even corporate bond pricing
Thin-skinned: are CCPs skimping on capital cover?
Growth of default funds calls into question clearers’ skin in the game
Quants dive into FX fixing windows debate
Longer fixing windows may benefit clients, but predicting how dealers will respond is tough
Talking Heads 2024: All eyes on US equities
How the tech-driven S&P 500 surge has impacted thinking at five market participants
Beware the macro elephant that could stomp on stocks
Macro risks have the potential to shake equities more than investors might be anticipating
Podcast: Piterbarg and Nowaczyk on running better backtests
Quants discuss new way to extract independent samples from correlated datasets