Podcast: Alexei Kondratyev on quantum computing
Imperial College London professor updates expectations for future tech
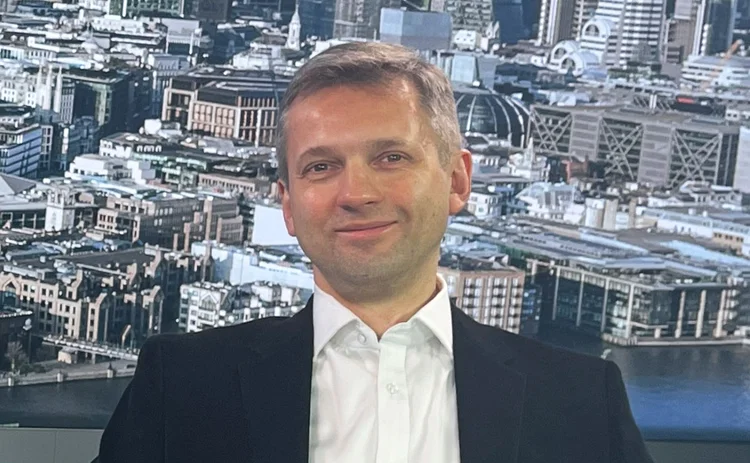
The first time Alexei Kondratyev appeared on Quantcast, he predicted that quantum computers would be commercially available and commonly used by banks within five years.
That was in 2019, when he was leading quantum computing research at Standard Chartered – a position in which he had a unique vantage point to assess the technologies being developed by vendors and their potential applications.
While the first part of Kondratyev’s prediction was mostly correct – banks now have access to quantum computing services from several vendors – the second part has proven overly optimistic.
In this episode of Quantcast, Kondratyev – now a visiting professor at Imperial College London and a researcher at the Abu Dhabi Investment Authority (ADIA) Lab – makes a new prediction.
“I think in five years, we should probably see business processes in the production environment being executed partially on quantum computers,” he says. “And my prediction would be that it will happen through classical supercomputers, working in parallel with quantum processors, outsourcing some function calls to QPUs,” he says.
Kondratyev, together with David Garvin and Marco Paini of Rigetti Computing, and his former ADIA colleague Alex Lipton, recently published a paper showing how a quantum algorithm can classify probability distributions better than classical computers.
Classifying probability distributions is a common problem in finance. It comes up, for example, when testing the performance of investment strategies in-sample versus out-of-sample. The goal is to answer the question: do the two samples come to the same multivariate probability distribution or are they distinct? The process is more difficult than it sounds.
“This is very complex multivariate analysis,” explains Kondratyev. The difficulty lies in choosing from a wide range of possible models – each with its own pros and cons – to represent the features of the distribution. “Therefore, it was very interesting and tempting to see if we could address this problem and formulate a quantum [algorithm] to sample test that can help us to classify multivariate distributions,” he says.
With a classical algorithm, the dimensionality of the problem scales quadratically with the size of the dataset, making it intractable for large datasets. With a quantum algorithm, the dimensionality scales linearly. And while large datasets are not the norm in finance, the quantum algorithm may prove useful to those working with high-frequency data.
Kondratyev explains how their quantum algorithm can be applied to this problem by encoding datasets into corresponding density matrixes – the mathematical descriptions of quantum systems. The algorithm can then efficiently calculate the distances between the two probability distributions.
The results detailed in the paper show that, in addition to being able to handle larger datasets, the quantum algorithm reaches conclusions with a higher degree of confidence.
Kondratyev’s work stands in stark contrast to the bulk of papers on quantum computing, which seem to apply super-fast algorithms to ad hoc problems that are of no practical value – a bugbear of Kondratyev’s. He describes newly unveiled Google’s Willow processor as “totally useless”.
“It’s a specially constructed problem,” he says of the benchmarks used to test Willow, describing them as “potentially interesting if you want to characterise your quantum processor, but with absolutely no application in any field of human activity”.
Looking ahead, Kondratyev believes the future of computing is hybrid. “Quantum processors will be working together in parallel with classical supercomputers, with hybrid computational protocols that would outsource some complex function calls to quantum processors, but most of the protocol will be executed classically,” he says.
Index:
00:00 Introduction
02:25 Classification of probability distributions
08:00 Classifying distributions with a quantum algorithm
11:30 Crash course in quantum computing
15:20 Comparison between the quantum algorithm over a classical one
21:10 Results of the experiment
23:00 The collaboration among the authors
28:00 Practical uses of quantum computing
35:31 The state of art of the technology
39:40 Upcoming projects
42:15 Where will it be in five years
To hear the full interview, listen in the player above, or download. Future podcasts in our Quantcast series will be uploaded to Risk.net. You can also visit the main page here to access all tracks, or go to Spotify, Amazon Music or the iTunes store to listen and subscribe.
Only users who have a paid subscription or are part of a corporate subscription are able to print or copy content.
To access these options, along with all other subscription benefits, please contact info@risk.net or view our subscription options here: http://subscriptions.risk.net/subscribe
You are currently unable to print this content. Please contact info@risk.net to find out more.
You are currently unable to copy this content. Please contact info@risk.net to find out more.
Copyright Infopro Digital Limited. All rights reserved.
As outlined in our terms and conditions, https://www.infopro-digital.com/terms-and-conditions/subscriptions/ (point 2.4), printing is limited to a single copy.
If you would like to purchase additional rights please email info@risk.net
Copyright Infopro Digital Limited. All rights reserved.
You may share this content using our article tools. As outlined in our terms and conditions, https://www.infopro-digital.com/terms-and-conditions/subscriptions/ (clause 2.4), an Authorised User may only make one copy of the materials for their own personal use. You must also comply with the restrictions in clause 2.5.
If you would like to purchase additional rights please email info@risk.net
More on Views
UBS’s Iabichino holds a mirror to bank funding risks
Framing funding management as an optimal control problem affords an alternative to proxy hedging
Quants mine gold for new market-making model
Novel approach to modelling cointegrated assets could be applied to FX and potentially even corporate bond pricing
Quants dive into FX fixing windows debate
Longer fixing windows may benefit clients, but predicting how dealers will respond is tough
Podcast: Piterbarg and Nowaczyk on running better backtests
Quants discuss new way to extract independent samples from correlated datasets
BofA quants propose new model for when to hold, when to sell
Closed-form formula helps market-makers optimise exit strategies
Podcast: Alvaro Cartea on collusion within trading algos
Oxford-Man Institute director worries ML-based trading could have anti-competitive effects
Podcast: Lorenzo Ravagli on why the skew is for the many
JP Morgan quant proposes a unified framework for trading the volatility skew premium
Counterparty risk model links defaults to portfolio values
Fed’s Michael Pykhtin proposes using copula models to capture effects of margin calls on default risk