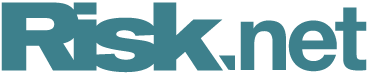
Podcast: Piterbarg and Nowaczyk on running better backtests
Quants discuss new way to extract independent samples from correlated datasets

Quants routinely run backtests to gauge the quality of their models. To ensure accuracy, backtesting must be executed on independent data. This condition is often not met.
Most datasets exhibit correlation between variables, or autocorrelation in a single variable. One example is year-on-year inflation data – each monthly print shows the percentage change over the previous 12 months, with 11 of those inputs also used to calculate the previous month’s figure, resulting in a time series that is highly autocorrelated.
“If you just ignore that in your backtesting framework, the results will be materially incorrect,” says Nikolai Nowaczyk, a risk management and artificial intelligence consultant who has been helping NatWest solve this problem.
The easiest remedy is to simply take fewer samples – for instance, one inflation print per year, instead of 12. This would generate independent samples, but fewer of them, diminishing the power of the statistic.
Another approach would be to simulate a large number of paths using the model being tested, build an empirical distribution of the test statistic, and then divide the results into the quintiles that are most relevant to the exercise at hand. This can be an effective solution, but it can also be computationally intensive, given the large number of simulations involved.
In this episode of Quantcast, Nowaczyk and Vladimir Piterbarg, head of quantitative analytics at Natwest Markets, discuss an alternative methodology for dealing with this problem, based on the idea of decorrelating samples. This does not mean removing the correlation effects from the test statistic, but rather decorrelating the original data and reducing the process to a standard statistical test.
To do this, they first derive the correlation matrix, which in many cases can be calculated analytically or, in the worst case, simulated. The information in the correlation matrix can then be removed from the samples, resulting in a dataset that is suitable for backtesting.
This technique can be useful for backtesting counterparty credit risk and initial margin models, where datasets tend to be small, and observation windows long.
“We use it for counterparty credit risk,” says Piterbarg. “This is where the problem is most pronounced. We hired Nikolai to help us on that front, and he came up with this idea.”
Piterbarg admits the methodology is not particularly intuitive and can be difficult to explain. The easiest way to think about it, he says, is that it magnifies the features of the tail of the distributions, which is key to the backtests the bank runs on counterparty credit models.
In addition to backtesting, Nowaczyk and Piterbarg also discuss their other current research projects, which as it turns out are completely decorrelated from each other. Nowaczyk is working on a simulation of an economic ecosystem of central counterparties, which he thinks will reveal new insights into the systemic risks they pose. Piterbarg, who helped organise the launch of the Paul Lévy Prize in Probability Theory, is turning his attention back to interest rate derivatives. He is developing a model to capture the mean reversion effects in factor models that describe that market. We should hopefully hear more about those projects in a future episode of Quantcast.
Index
00:00 Backtesting in the presence of correlation
04:10 Backtesting using Monte Carlo simulations
08:09 Decorrelating correlated variables
10:00 Application to counterparty credit risk
15:51 Why this method works
19:35 Does decorrelation generate independent samples?
21:00 The Paul Lévy Prize in Probability Theory
25:25 Future research projects
To hear the full interview, listen in the player above, or download. Future podcasts in our Quantcast series will be uploaded to Risk.net. You can also visit the main page here to access all tracks, or go to Spotify, Amazon Music or the iTunes store to listen and subscribe.
Only users who have a paid subscription or are part of a corporate subscription are able to print or copy content.
To access these options, along with all other subscription benefits, please contact info@risk.net or view our subscription options here: http://subscriptions.risk.net/subscribe
You are currently unable to print this content. Please contact info@risk.net to find out more.
You are currently unable to copy this content. Please contact info@risk.net to find out more.
Copyright Infopro Digital Limited. All rights reserved.
As outlined in our terms and conditions, https://www.infopro-digital.com/terms-and-conditions/subscriptions/ (point 2.4), printing is limited to a single copy.
If you would like to purchase additional rights please email info@risk.net
Copyright Infopro Digital Limited. All rights reserved.
You may share this content using our article tools. As outlined in our terms and conditions, https://www.infopro-digital.com/terms-and-conditions/subscriptions/ (clause 2.4), an Authorised User may only make one copy of the materials for their own personal use. You must also comply with the restrictions in clause 2.5.
If you would like to purchase additional rights please email info@risk.net
More on Views
Getting a handle on model parameters
Mean reversion in rate parameters opens the door to dimensionality reduction
Podcast: adventures in autoencoding
Trio of senior quants explain how autoencoders can reduce dimensionality in yield curves
Podcast: Lyudmil Zyapkov on the relativity of volatility
BofA quant’s new volatility model combines gamma processes and fractional Brownian motion
A market-making model for an options portfolio
Vladimir Lucic and Alex Tse fill a glaring gap in European-style derivatives modelling
Degree of influence 2024: volatility and credit risk keep quants alert
Quantum-based models and machine learning also contributed to Cutting Edge’s output
Podcast: Alexandre Antonov turns down the noise in Markowitz
Adia quant explains how to apply hierarchical risk parity to a minimum-variance portfolio
UBS’s Iabichino holds a mirror to bank funding risks
Framing funding management as an optimal control problem affords an alternative to proxy hedging
Podcast: Alexei Kondratyev on quantum computing
Imperial College London professor updates expectations for future tech