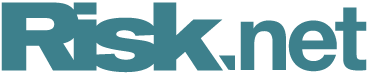
How to hone NLP’s detection skills: a cue from a super-sleuth
With a sharper focus, AI readers could help detect hidden exposures for investors
Navigating the so-called mind palace – a skill that uses context to make mental connections – is an Ancient Greek technique deployed by the smartest of fictional detectives in one of his modern-day incarnations.
But could something like Sherlock’s method help investors realise the utility of natural language processing – the field of artificial intelligence that trains machines to read text – by using similar situational cues to examine a company’s exposure to new threats?
Some asset managers are already using NLP to light up the trail of clues that discretionary investors might use to connect these dots. They are searching with NLP for specific signs of trouble in a firm’s own document trail.
And while such firms are openly broadcasting their use of NLP, critics warn the discipline is still in its infancy. NLP engines grasp facts in a dream-like cluster – a patchwork of information lacking sense or context – the informational equivalent of a word cloud.
These limitations have in the past circumscribed NLP’s utility. NLP-derived alpha signals have proven elusive. Most NLP technology failed to see the Ukraine war coming until very late.
And although there’s no denying NLP is a ground-breaking technology, in some respects it’s a dumb one. Asset managers have struggled to find ways to get the most out of it.
As they do so, one idea might be to use the technique in bottom-up, stock-by-stock fundamental analysis.
Instead of weighing the importance of events such as the Ukraine invasion in the news – and then gauging stock betas to that sentiment signal – the alternative approach is to investigate a company’s exposure to a specific emerging risk or opportunity.
A more focused NLP analysis can show how often a topic has been discussed in a firm’s earnings calls, say, but also in company documents as well as public information such as the firm’s news.
Case study
For Voya Asset Management, such an approach was helpful during Russia sanctions, when the effects on individual companies were hard to gauge.
According to Chrissy Bargeron, client portfolio manager covering equity at the asset manager, traditional country-at-risk models capture a lot of the more obvious direct risk when it comes to performance. What is less clear-cut, she says, is the indirect risk.
The firm is trying to find out if there are “different themes at play” behind the headline, geopolitical risks, says Bargeron.
The main goal of the firm’s AI machine learning team is to apply ML to fundamental analysis.
It’s a markedly bottom-up rather than top-down approach.
A machine can pick out more easily than a human, for example, whether revenues are declining in a geographical pattern – a signal that might trigger before news headlines alert investors to a problem.
In the Russia-Ukraine crisis, the first aim of risk management was to minimise direct ownership of Russian securities. As it turned out, Voya owned very little.
But the firm also wanted to know which companies it held were carrying some indirect exposure, such as those that sell a lot to Russia or Ukraine.
The AI team applied NLP to news and earnings-call transcripts to look for mentions of either country.
Their engine picked out 59 stocks, for which Voya was then able to gauge sensitivity to the Russian equity market using more conventional quant regression techniques.
“We found this to be very useful. And it was reactive. We did it right when the crisis happened,” says Bargeron. “We were able to quickly scan all of this and see, within our developed equity investments, where we had that exposure.”
Bottom-up, fundamental analysis. Elementary?
Editing by Louise Marshall
Only users who have a paid subscription or are part of a corporate subscription are able to print or copy content.
To access these options, along with all other subscription benefits, please contact info@risk.net or view our subscription options here: http://subscriptions.risk.net/subscribe
You are currently unable to print this content. Please contact info@risk.net to find out more.
You are currently unable to copy this content. Please contact info@risk.net to find out more.
Copyright Infopro Digital Limited. All rights reserved.
As outlined in our terms and conditions, https://www.infopro-digital.com/terms-and-conditions/subscriptions/ (point 2.4), printing is limited to a single copy.
If you would like to purchase additional rights please email info@risk.net
Copyright Infopro Digital Limited. All rights reserved.
You may share this content using our article tools. As outlined in our terms and conditions, https://www.infopro-digital.com/terms-and-conditions/subscriptions/ (clause 2.4), an Authorised User may only make one copy of the materials for their own personal use. You must also comply with the restrictions in clause 2.5.
If you would like to purchase additional rights please email info@risk.net
More on Our take
Beware the macro elephant that could stomp on stocks
Macro risks have the potential to shake equities more than investors might be anticipating
Podcast: Piterbarg and Nowaczyk on running better backtests
Quants discuss new way to extract independent samples from correlated datasets
Should trend followers lower their horizons?
August’s volatility blip benefited hedge funds that use short-term trend signals
Low FX vol regime fuels exotics expansion
Interest is growing in the products as a way to squeeze juice out of a flat market
Can pod shops channel ‘organisational alpha’?
The tension between a firm and its managers can drag on returns. So far, there’s no perfect fix
CDS market revamp aims to fix the (de)faults
Proposed makeover for determinations committees tackles concerns over conflicts of interest
BofA quants propose new model for when to hold, when to sell
Closed-form formula helps market-makers optimise exit strategies
Are regulators wrong to think of AT1s as debt?
Bank capital bonds face criticism. One answer might be to treat them as ‘fixed-income equity’