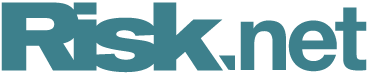
Robo-traders and robo-labour
Banks and buy-siders are starting to harvest the benefits of machine learning beyond the front office

The application of machine learning to generate trading strategies might grab the headlines, but its use elsewhere within financial firms also deserves attention.
Machines are being put to work in areas such as model validation and credit risk, in addition to activities such as trading execution. Teaching a computer how to make money remains a work in progress, but teaching it, say, to spot errors in overnight risk calculations could be both easier and less hazardous.
In trading, Bloomberg is one firm employing tools to analyse fixed-income liquidity that draw on machine-learning techniques. The vendor uses cluster analysis – a machine-learning method – to estimate transaction costs for illiquid bonds where relevant trading data is sparse. Machine learning helps determine the similarity of apparently unrelated bonds.
Natixis, meanwhile, is using machine learning to alert it to anomalies in its stress testing results. Regulatory pressure has loaded model validators with greater workloads, and machine-learning techniques help ease that burden, freeing staff up to focus on more pressing issues.
Machine learning is really just sophisticated statistical analysis for large libraries of data. It uses an algorithm like any other program, but rather than being told explicitly what to do, it is told how to construct and revise hypotheses using statistical methods.
Greater volumes of data, increasingly powerful hardware and the development of sophisticated algorithms have all contributed to its rise.
But one of machine learning’s greatest strengths is also one of its greatest weaknesses. By allowing a computer to learn for itself, without an explicit authoritative code, it becomes harder to explain the computer’s conclusions.
This is the notorious ‘black box’ problem: “Data goes in and data comes out, but what happens in between?” critics ask. Detractors worry that by dint of relying so heavily on historical data, machine learning’s statistical results are invariably overfitted. They fret that such methods will be applied to problems where data is too scarce or where relationships between data are changing.
Quants are right to be wary – but wariness should be applied to any tool. Machine learning can point to demonstrable successes when aptly used. Firms are steadily working out that its application is well-suited to areas where resource-stretched teams are drowning in data – problems that are equally likely to be found in the back office as in the front.
Only users who have a paid subscription or are part of a corporate subscription are able to print or copy content.
To access these options, along with all other subscription benefits, please contact info@risk.net or view our subscription options here: http://subscriptions.risk.net/subscribe
You are currently unable to print this content. Please contact info@risk.net to find out more.
You are currently unable to copy this content. Please contact info@risk.net to find out more.
Copyright Infopro Digital Limited. All rights reserved.
As outlined in our terms and conditions, https://www.infopro-digital.com/terms-and-conditions/subscriptions/ (point 2.4), printing is limited to a single copy.
If you would like to purchase additional rights please email info@risk.net
Copyright Infopro Digital Limited. All rights reserved.
You may share this content using our article tools. As outlined in our terms and conditions, https://www.infopro-digital.com/terms-and-conditions/subscriptions/ (clause 2.4), an Authorised User may only make one copy of the materials for their own personal use. You must also comply with the restrictions in clause 2.5.
If you would like to purchase additional rights please email info@risk.net
More on Our take
Podcast: Lorenzo Ravagli on why the skew is for the many
JP Morgan quant proposes a unified framework for trading the volatility skew premium
Quants see promise in DeBerta’s untangled reading
Improved language models are able to grasp context better
Counterparty risk model links defaults to portfolio values
Fed’s Michael Pykhtin proposes using copula models to capture effects of margin calls on default risk
Does Basel’s internal loss multiplier add up?
As US agencies mull capital reforms, one regulator questions past losses as an indicator of future op risk
Is JSCC-CFTC stalemate about to be broken?
Japan CCP gains allies in battle to clear yen swaps for US clients, but CFTC shakeup could dash hopes
What T+1 risk? Dealers shake off FX concerns
Predictions of increased settlement risk and later-in-the-day trading have yet to materialise
Go your own way: departures pose new challenges for CFTC
Loss of Democratic majority would impede chairman’s ambitions for regulatory agenda
Altice’s dropdown is a warning for European creditors
Carve-out used to shield assets from lenders may occur in a fifth of European deals