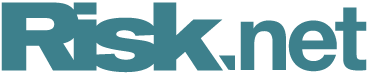
Banks should quantify loan-loss model risk – academic
Models such as those used for IFRS 9, CECL or CCAR are prone to errors, and should be accounted for

Banks should account for – and capitalise against – the risk of the models they use to forecast losses on loans being wrong, says forthcoming research.
In Quantification of model risk in stress testing and scenario analysis, the SAS Institute’s Jimmy Skoglund proposes a method of quantifying model risk using stressed transition probability matrices for credit loss impairment forecasting, in a bid to put a dollar value on the amount banks should set aside against the risk of their models offering inaccurate forecasts.
Two major strands of post-crisis reform – the shift to expected credit loss accounting, and the introduction of stress tests that rely on macroeconomic loss forecasting – have pushed banks to develop more accurate credit loss forecasting models. Most obviously, the new international accounting standard, IFRS 9, and its sister US standard, current expected credit loss, ask banks to provision against the risk of loans turning sour based on forecasted future losses, rather than waiting till losses are incurred.
Regulatory stress-testing regimes, meanwhile – most obviously the US Federal Reserve’s Comprehensive Capital Analysis and Review programme, as well as Europe-based ones run by the European Banking Authority – require banks to measure their capital adequacy against a series of adverse scenarios over a given period of time.
That makes the assumptions that underpin these models crucial from a capital standpoint – but it is rare that banks actively capitalise against the risk of their outputs being wrong, says Skoglund.
“Current analysis of model risk does not translate into actual numbers that allow explicit quantification of a model risk buffer,” argues Skoglund. “Even under best-estimate impairment forecasts, stressed macroeconomic scenarios are frequently being included to mitigate overly positive scenario selection bias in impairments estimation.”
Banks have complained that regulatory guidance on the new expected credit loss modelling under the new accounting regimes has been vague. IFRS 9, for instance, asks banks to base their estimates of a loan’s lifetime expected credit losses on ‘reasonable and supportable’ information – a requirement that banks argue lacks a clear definition. Others have pointed out this leeway could encourage banks to pick and choose the forecasts they feed into their models – for instance, by shortening time horizons to avoid including data where downturns are expected.
Current analysis of model risk does not translate into actual numbers that allow explicit quantification of a model risk buffer
Jimmy Skoglund, SAS Institute
With a focus on loss underestimation, Skoglund’s research seeks to account for the model risk he argues is in inherent credit loss projections, demonstrating how portfolio loss forecasts can change when this is considered. Skoglund posits that, since financial crises are – statistically speaking – rare events, models developed for stressed-loss forecasting are prone to significant model risk.
Proposing a ‘robustness approach’, the paper says that model risk can be broadened out beyond considering parametric uncertainty; by applying relative entropy techniques, loss forecasts are “tilted” to consider model risk.
Skoglund uses proportional hazard models to generate macroeconomic and idiosyncratic loan path-dependent state transition probabilities in a delinquency state transition. Using relative entropy techniques, the “distance” between a set of models and a base model is measured. Model risk is given as the worst case out of all models within a given distance for a given payout. This allows model mis-specification robustness to be numerically quantified using exponential tilting towards an alternative probability law. Without specifying explicit alternative models, robustness is sought against alternative models within a certain distance.
Skoglund says: “The worst-case obtained represents in general an upward scaling of the term-structure consistent with the exponential tilting adjustment. The relative entropy approach to model risk we use has its foundation in economics with robust forecasting analysis, and has recently started to be applied in risk management.”
Skoglund uses a robust estimator of the portfolio loss tilted away from the plain average loss using a loss ratio, controlled by a parameter determining the degree of deviation from the plain average loss.
Because a model generates a number of outcomes, posits Skoglund, it is prudent to consider not only average loss predictions, but also some measure of the worst outcome. Relative entropy provides such a prudent estimate of expected loss using the exponential tilting of the loss estimate.
Because the model loss estimates that are tilted away from the original model are measured in currency amounts, they can be used for quantification of model risk buffers under the relative entropy framework.
Skoglund emphasises that his robustness approach is intended to complement, rather than replace, traditional model risk management practices – the use of sensitivities-based analyses or challenger models, for example – and does not negate the need for qualitative model risk management approaches, such as the use of conservative assumptions.
Skoglund’s paper will be published in the March 2019 issue of the Journal of Risk Model Validation.
Editing by Tom Osborn
Only users who have a paid subscription or are part of a corporate subscription are able to print or copy content.
To access these options, along with all other subscription benefits, please contact info@risk.net or view our subscription options here: http://subscriptions.risk.net/subscribe
You are currently unable to print this content. Please contact info@risk.net to find out more.
You are currently unable to copy this content. Please contact info@risk.net to find out more.
Copyright Infopro Digital Limited. All rights reserved.
As outlined in our terms and conditions, https://www.infopro-digital.com/terms-and-conditions/subscriptions/ (point 2.4), printing is limited to a single copy.
If you would like to purchase additional rights please email info@risk.net
Copyright Infopro Digital Limited. All rights reserved.
You may share this content using our article tools. As outlined in our terms and conditions, https://www.infopro-digital.com/terms-and-conditions/subscriptions/ (clause 2.4), an Authorised User may only make one copy of the materials for their own personal use. You must also comply with the restrictions in clause 2.5.
If you would like to purchase additional rights please email info@risk.net
More on Risk management
More cleared repo sponsors join Eurex ahead of cross-margining
End of TLTROs for banks and pension fund search for liquidity management tools drives uptake
Reimagining model risk management: new tools and approaches for a new era
A collaborative report by Chartis and Evalueserve on how the use of automation can combat the growing complexity of managing model risk due to regulation and market volatility
What Goldman’s appeal victory means for Fed stress tests
Decision could embolden more banks to appeal, analysts say. But others believe result is one-off
Clearing members rattled as CME approved to launch its own FCM
National Futures Association registration sharpens concerns about conflict of interest with CCP
CME files application for US Treasury and repo clearing
New entrant believes direct user access model will avoid accounting problem that hampers rival FICC
UST repo clearing: considerations for ‘done-away’ implementation
Citi’s Mariam Rafi sets out the drivers for sponsored and agent clearing of Treasury repo and reverse repo
Gensler to stick to Treasury clearing timetable
SEC chief promises to keep up the pressure for done-away trades
Clearing houses fear being classified as Dora third parties
As 2025 deadline looms, CCP and exchange members seek risk information that’s usually deemed confidential