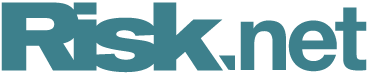
How XVA quants learned to stop worrying and trust the machine
Initial scepticism about using neural networks for derivatives pricing is giving way to enthusiasm
Jesper Andreasen knows a thing or two about speeding up the calculation of derivatives valuation adjustments, or XVAs.
So, when Microsoft claimed in a blog post last year that a neural network running on its Azure cloud platform could do the job up to 20 million times faster than existing models, the head of quant research at Saxo Bank – and self-styled Kwantfather – was sceptical.
Andreasen dismissed the idea on LinkedIn, saying it could only appeal to those with “more computers than brain cells”. Among other things, he argued the neural networks would take too long to train and may not be reliable enough to be used by the front office. Several other leading quants agreed.
Now, most of those questions have been put to rest. Last year, Scotiabank began using a deep neural network developed by Riskfuel, a fintech start-up, to approximate the outputs of the Monte Carlo models it uses for derivatives pricing. The neural network was trained within a day using the vast amounts of computing power available on Microsoft’s cloud platform. The bank credits the new system with improving both the speed and accuracy of its XVA calculations.
Since then, quants Antoine Savine and Brian Huge at Danske Bank have published research describing a novel way to speed up the training process. They trained their neural network using the sampled payoffs and sensitivities of derivatives, rather than examples of prices. This improves the quality of the training data while lowering the computational cost and time required to train the network. The new system has proven to be reliable and is already being used by the bank’s traders and risk managers. “Our research has resolved some key problems in the application of machine learning to risk,” Huge, Danske Bank’s chief quant analyst, tells Risk.net.
Others are now racing to catch up. At least four large dealers, including Barclays, Citi and HSBC, are actively exploring the use of neural networks for derivatives valuations. Ryan Ferguson, founder and chief executive of Riskfuel, reckons around a dozen banks are looking at implementing the technology.
Neural networks contain multiple hidden layers that transform the input data in ways that are often difficult to trace, let alone explain
The hurdles to wider adoption haven’t fallen away entirely, though. The training process can still be unduly burdensome for dealers with large trading books comprising a mix of complex products. Quants at JP Morgan have already decided to look for other ways to speed up XVA calculations.
There’s also the lingering question of explainability. Neural networks contain multiple hidden layers that transform the input data in ways that are often difficult to trace, let alone explain. Increased regulatory scrutiny could slow the adoption of more complex deep learning techniques, such as those used for XVA calculations. A 2020 report from the European Banking Authority concluded that it was “premature to consider machine learning an appropriate tool for determining capital requirements, taking into account current limitations”, such as explainability.
Danske’s neural network is not yet being used to report official numbers to regulators, although Huge has no doubt it will be.
Other regulatory developments though may push banks to adopt neural networks. The standardised approach to credit valuation adjustments (SA-CVA), due to go live in January 2023, will require banks to calculate CVA sensitivities for all risk factors – a computationally intensive task that could push the limits of tried-and-tested technologies.
Among quants, there is a growing sense that deep neural networks will soon become a standard part of the XVA pricing toolkit. At Saxo Bank, Andreasen, who has been actively studying machine learning since 2017, is now considering using neural networks to model both the sensitivities of derivatives to underlying risk factors, as well as the co-movement of volatility surfaces.
Editing by Helen Bartholomew
Only users who have a paid subscription or are part of a corporate subscription are able to print or copy content.
To access these options, along with all other subscription benefits, please contact info@risk.net or view our subscription options here: http://subscriptions.risk.net/subscribe
You are currently unable to print this content. Please contact info@risk.net to find out more.
You are currently unable to copy this content. Please contact info@risk.net to find out more.
Copyright Infopro Digital Limited. All rights reserved.
As outlined in our terms and conditions, https://www.infopro-digital.com/terms-and-conditions/subscriptions/ (point 2.4), printing is limited to a single copy.
If you would like to purchase additional rights please email info@risk.net
Copyright Infopro Digital Limited. All rights reserved.
You may share this content using our article tools. As outlined in our terms and conditions, https://www.infopro-digital.com/terms-and-conditions/subscriptions/ (clause 2.4), an Authorised User may only make one copy of the materials for their own personal use. You must also comply with the restrictions in clause 2.5.
If you would like to purchase additional rights please email info@risk.net
More on Our take
Quants dive into FX fixing windows debate
Longer fixing windows may benefit clients, but predicting how dealers will respond is tough
Talking Heads 2024: All eyes on US equities
How the tech-driven S&P 500 surge has impacted thinking at five market participants
Beware the macro elephant that could stomp on stocks
Macro risks have the potential to shake equities more than investors might be anticipating
Podcast: Piterbarg and Nowaczyk on running better backtests
Quants discuss new way to extract independent samples from correlated datasets
Should trend followers lower their horizons?
August’s volatility blip benefited hedge funds that use short-term trend signals
Low FX vol regime fuels exotics expansion
Interest is growing in the products as a way to squeeze juice out of a flat market
Can pod shops channel ‘organisational alpha’?
The tension between a firm and its managers can drag on returns. So far, there’s no perfect fix
CDS market revamp aims to fix the (de)faults
Proposed makeover for determinations committees tackles concerns over conflicts of interest