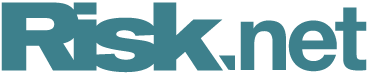
Calling out autocallable pricing
Quants show popular autocallable pricing technique has a flaw that has been ignored until now

Autocallables have been one of the most successful structured equity products in recent years, providing investors with the ability to earn coupons as high as 10% in a low-yield environment.
The products essentially have two types of barriers – upside and downside. If spot remains within these bounds, investors would receive an above-market coupon. The structure knocks out when spot hits the upside barrier, returning principal and an improved coupon to investors. The investors also sell the issuer a knock-in put option as part of the structure, so when the downside barrier triggers the put option, they could lose part of the principal. To boost coupons, autocallables are often written on the lagged component of a basket of underlyings.
Given the description of the product, it is not surprising that its pricing is error-prone. Simple local volatility models – which calibrate very well to vanilla prices – won’t cut it because they are not good at capturing the path-dependent nature of autocallables.
This means the value of the product at any point in time would depend on the path of the movement of the underlying rather than its absolute levels. So the forward probabilities of events such as whether the lower barrier is knocked in, given the upper barrier has not been breached, need to be estimated.
Market standard is to use a stochastic local volatility (SLV) model, which is better at capturing path dependency. The trouble is, when SLV models are used for a basket of underlyings, which is more common than a single underlying, a well-known effect called decorrelation shows up. The addition of a stochastic volatility component reduces the correlations between the underlying spots to below their input levels. Multi-autocallable products are typically long correlation, so lower correlation lowers the price. Overall, autocallables are priced higher in SLV compared with local volatility models.
“When you go from local volatility to SLV you observe an effect that is often referred to as decorrelation. It means that due to the volatility fluctuations in the stochastic volatility model that are higher in the SLV model, the effective realised correlation is smaller in this model than the local volatility model,” says Patrick Kuppinger, head of equities and forex model validation at Vontobel in Zurich, who was in the UBS business solutions team at the time the article was written.
“What you would expect from that is, in isolation, it would have a negative pricing impact on the autocallables.”
Kuppinger adds this is a well-known effect in the industry, and what quants possibly do to correct for it is increase the correlations between the spots before feeding them into the model.
In this month’s technical, The interplay between stochastic volatility and correlations in equity autocallables, Kuppinger and Alvise De Col, head of the loan portfolio advisory group in the global wealth management division – and previously part of the business solutions team – of UBS in Zurich – show there is a second opposing effect that biases autocallables priced using SLV models – called bilocality. This means correcting for decorrelation alone does not ensure the prices are somewhat free of unwanted biases.
Bilocality lowers the realised volatility in the SLV model compared to the local volatility model, especially around the at-the-money levels of the put option. Autocallables are short volatility, so when it is reduced the price goes up – an opposite effect to decorrelation. So if this effect dominates, prices are driven higher rather than lower. Simply fixing the correlation – as quants have been doing – will correct the prices in the “wrong direction” – they will go further up, when they are already biased upwards.
“We were somewhat puzzled when we saw the effects were opposite in sign to what we were expecting,” says De Col.
The fix
Given there is now a better understanding of these effects, the pair say quants can fix the undesirable pricing issues. When the correlation between the variances of the underlyings are large, bilocality is more likely to dominate, they argue.
“In case you have a very large vol-vol correlation you see the bilocality effect is the one that matters the most. Whereas in the case of no correlation between the variances, the decorrelation is the most important effect. It’s the call of the risk manager to decide what they like and what is actually more in line with market behaviour,” says De Col.
Many quants focus on devising new ways to boost computing speed or applying a complicated technique borrowed from physics to price exotic derivatives. While these developments are crucial to progress in this field, it also pays to dedicate time and effort into studying a model’s limitations for a range of scenarios. As is made evident in this paper, sometimes the simple fixes made on the basis of intuition alone may make matters worse.
Only users who have a paid subscription or are part of a corporate subscription are able to print or copy content.
To access these options, along with all other subscription benefits, please contact info@risk.net or view our subscription options here: http://subscriptions.risk.net/subscribe
You are currently unable to print this content. Please contact info@risk.net to find out more.
You are currently unable to copy this content. Please contact info@risk.net to find out more.
Copyright Infopro Digital Limited. All rights reserved.
As outlined in our terms and conditions, https://www.infopro-digital.com/terms-and-conditions/subscriptions/ (point 2.4), printing is limited to a single copy.
If you would like to purchase additional rights please email info@risk.net
Copyright Infopro Digital Limited. All rights reserved.
You may share this content using our article tools. As outlined in our terms and conditions, https://www.infopro-digital.com/terms-and-conditions/subscriptions/ (clause 2.4), an Authorised User may only make one copy of the materials for their own personal use. You must also comply with the restrictions in clause 2.5.
If you would like to purchase additional rights please email info@risk.net
More on Our take
Why did UK keep the pension fund clearing exemption?
Liquidity concerns, desire for higher returns and clearing capacity all possible reasons for going its own way
UBS’s Iabichino holds a mirror to bank funding risks
Framing funding management as an optimal control problem affords an alternative to proxy hedging
Trump 2.0 bank supervision: simpler but no soft touch?
Republican FDIC vice-chair Travis Hill wants more focus on financial risk instead of process
Lots to fear, including fear itself
Binary scenarios for key investment risks in this year’s Top 10 are worrying buy-siders
Podcast: Alexei Kondratyev on quantum computing
Imperial College London professor updates expectations for future tech
Quants mine gold for new market-making model
Novel approach to modelling cointegrated assets could be applied to FX and potentially even corporate bond pricing
Thin-skinned: are CCPs skimping on capital cover?
Growth of default funds calls into question clearers’ skin in the game
Quants dive into FX fixing windows debate
Longer fixing windows may benefit clients, but predicting how dealers will respond is tough