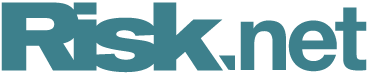
You don’t need to sacrifice accuracy for flexibility
BAML quant proposes option pricing model that softens conflict between the two properties
For many years, quants have been using the stochastic alpha, beta, rho (SABR) model to price swaptions. Despite the fact the model produces arbitrageable prices at the high and low strikes, SABR has remained very popular because of a useful expansion that allows one to calibrate the model exactly to market-implied volatility surfaces very quickly.
One of the biggest challenges for quants who have attempted in the past to fix the problems with SABR was this fundamental dilemma between flexibility and accuracy.
Sometimes the focus on flexibility may lead to models that deviate from the original dynamics, or are arbitrageable. Trying to align with the dynamics more accurately, however, may create models that are computationally cumbersome.
“People have spent of lot of effort and energy in either trying to fix the original formula with sophisticated techniques or pragmatically building models that deviate significantly from the dynamic’s intuition, sometimes losing sight of what the requirements are from a trading perspective,” says Dominique Bang, head of interest rate vanilla analytics at Bank of America Merrill Lynch. “While a lot of papers came out with noticeable improvements, I was always under the impression the solutions weren’t fully satisfactory.”
As a solution, in this month’s first technical, Local stochastic volatility: shaken, not stirred, Bang proposes a local stochastic volatility (LSV) model that can stay sufficiently true to the original stochastic volatility model dynamics and is arbitrage-free. The model is flexible enough to support any local or stochastic volatility model – the latter is not just restricted to SABR.
Local volatility models are one of the most popular option pricing models in the industry today. This isn’t surprising, since they combine two very desirable features – they are good at calibrating exactly to the volatility smile, which means they can match current market prices well. On the other hand, stochastic volatility models reflect the evolution of the market dynamics better. So mixing the two seems like having the best of both worlds.
But attempts to do so have been riddled with problems. For instance, it is not easy, and many quants use approximations to do it, which can lead to arbitrage.
“It is often the case that the approximation makes some assumptions like small volatility of volatility, time to maturity and moneyness. However, the model is used in practice with large maturity and large moneyness, and sometimes large volatility of volatility. So the main domain of applicability of the approximation is somehow violated, and this is how the arbitrage becomes significant,” says Bang.
People have spent of lot of effort and energy in either trying to fix the original formula with sophisticated techniques or pragmatically building models that deviate significantly from the dynamic’s intuition
Dominique Bang, Bank of America Merrill Lynch
In Bang’s paper, the quant applies something called a Lamperti transform to fix this. The transform works by mapping the terminal distribution of a pure stochastic volatility model to the terminal distribution of the underlying. The option in the resulting model can be written as a one-dimensional integral of options in the pure stochastic volatility model.
The mapping fully preserves the distribution of the stochastic model and properly accounts for the dynamics’ dependency to the level of volatility – a common difficulty with existing models, which makes them inflexible.
One caveat is that the overall speed of pricing will depend on the choice of the stochastic volatility model. If it’s quick to price options on the pure stochastic volatility component, the overall LSV model will be quick too. Bang’s paper illustrates this for a normal SABR model. The resulting model can price options to the order of 10,000 options per second.
Since there is flexibility in what type of local or stochastic volatility model one can use in Bang’s approach, quants can pick and choose models based on relevance to a given problem. For instance, if they need to factor in negative interest rates, they can input a local volatility model that can handle it. This is better than just shifting the distribution to avoid negative rates – a common approach in the field.
“If you want your model to comply with negative rates, you have to be able to support this feature in a way that makes sense. You have to be able to calibrate your model to a decent number of liquid swaptions and also you need to have a model that is consistent with convex products. And the pricing of convex products depends strongly on the behaviour of the model in the high strikes wing. So all these constraints we can enforce by using a proper parameterisation of the local volatility model,” says Bang. “Anyone can design it the way they want.”
It’s not hard to sympathise with quants these days, having to tackle regime changes such as negative interest rates, disruptive technology, pricing of valuation adjustments and just an overall rise in the standard of modelling because of regulatory scrutiny.
So a flexible model such as Bang’s, which can be designed to suit a specific trading problem, may come in handy for many quants in the industry.
Only users who have a paid subscription or are part of a corporate subscription are able to print or copy content.
To access these options, along with all other subscription benefits, please contact info@risk.net or view our subscription options here: http://subscriptions.risk.net/subscribe
You are currently unable to print this content. Please contact info@risk.net to find out more.
You are currently unable to copy this content. Please contact info@risk.net to find out more.
Copyright Infopro Digital Limited. All rights reserved.
As outlined in our terms and conditions, https://www.infopro-digital.com/terms-and-conditions/subscriptions/ (point 2.4), printing is limited to a single copy.
If you would like to purchase additional rights please email info@risk.net
Copyright Infopro Digital Limited. All rights reserved.
You may share this content using our article tools. As outlined in our terms and conditions, https://www.infopro-digital.com/terms-and-conditions/subscriptions/ (clause 2.4), an Authorised User may only make one copy of the materials for their own personal use. You must also comply with the restrictions in clause 2.5.
If you would like to purchase additional rights please email info@risk.net
More on Our take
Quants mine gold for new market-making model
Novel approach to modelling cointegrated assets could be applied to FX and potentially even corporate bond pricing
Thin-skinned: are CCPs skimping on capital cover?
Growth of default funds calls into question clearers’ skin in the game
Quants dive into FX fixing windows debate
Longer fixing windows may benefit clients, but predicting how dealers will respond is tough
Talking Heads 2024: All eyes on US equities
How the tech-driven S&P 500 surge has impacted thinking at five market participants
Beware the macro elephant that could stomp on stocks
Macro risks have the potential to shake equities more than investors might be anticipating
Podcast: Piterbarg and Nowaczyk on running better backtests
Quants discuss new way to extract independent samples from correlated datasets
Should trend followers lower their horizons?
August’s volatility blip benefited hedge funds that use short-term trend signals
Low FX vol regime fuels exotics expansion
Interest is growing in the products as a way to squeeze juice out of a flat market