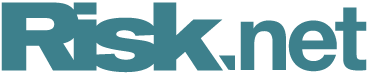
Podcast: Jan Rosenzweig on fat tails and LDI portfolios
An optimised portfolio can look very different when extreme moves are given more weight
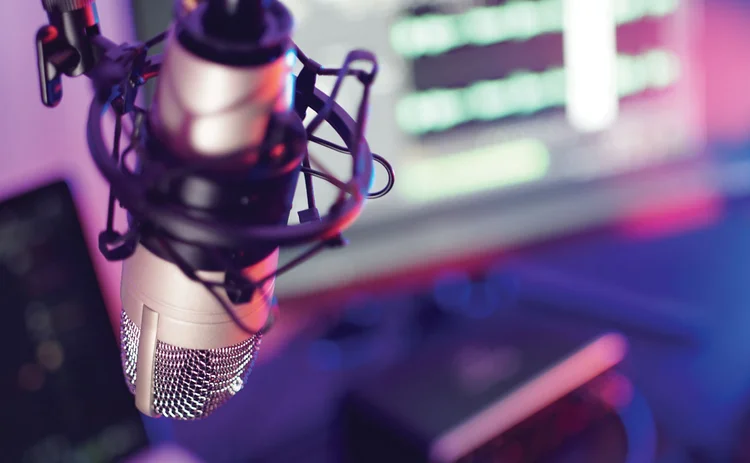
UK pensions learned a thing or two about fat tails and extreme moves during last year’s gilt crisis, when 30-year yields jumped nearly 100 basis points over the course of two trading days. Jan Rosenzweig’s familiarity with market-breaking events dates back further, to the global financial crisis of 2008 and 2009.
“Once you’ve lived through something like that, that’s always at the back of your mind,” he says. “I’ve seen first-hand how some of these things develop and why they develop, and I’ve been thinking in those terms [ever since].”
The experience prompted Rosenzweig, a portfolio manager at Pine Tree Market Neutral and visiting senior research fellow at King’s College London, to explore portfolio optimisation methods that defy standard thinking.
In this podcast, Rosenzweig – who also consults for insurers and pension funds – discusses the optimisation of liability-driven investments (LDI). His latest paper, published on Risk.net, shows that an optimised LDI portfolio looks very different if the assumption that returns are normally distributed is dropped.
Rosenzweig’s method relies on the decomposition of portfolios into uncorrelated components, using a technique he introduced in 2021 called independent component analysis. In that paper, he also introduced a risk-adjustment optimisation based on kurtosis – the fourth moment of the return distribution – rather than volatility. This approach penalises tail returns more than volatility-based optimisation.
In his new paper, Rosenzweig generalises that method to allow the risk adjustment to use any positive exponent. A high exponent identifies securities with extreme returns and minimises the allocation to them. When returns exhibit extreme deviation, the exponent corresponds to infinity. In that setting, the portfolio manager’s aim is to minimise the maximum deviation.
Identifying independent components, and filtering out assets prone to extreme deviation, results in an optimised portfolio that may differ significantly from one optimised under the assumption that returns are normally distributed.
Could this approach have prevented last year’s LDI crisis? Not directly. The problems were primarily caused by a funding gap, rather than poor portfolio optimisation. “The cushion wasn’t big enough,” says Rosenzweig.
But he also sees other lessons in the crisis. “There was too much focus on neutralising small market moves, and that ate up too much capital, probably unnecessarily,” says Rosenzweig.
This is something his portfolio optimisation method could help with. The approach described in the paper “allows any hedging to be very focused”, says Rosenzweig. “You can focus on the part of the distribution, you can focus on the set of returns you want to hedge against, and that means the actual capital you need to allocate for hedging is sometimes quite a bit smaller.”
And if pensions need less capital for hedging, they may have more available for other purposes. “So, in that sense, you have plenty of capital left over for cushioning the impact of any funding gaps, for pursuing returns, for anything else you might need extra capital for.”
Index
00:00 Intro
02:00 Portfolio optimisation and LDI investments
07:50 The optimisation method
09:28 Extreme deviation
13:12 Diversification
16:36 Future research
17:43 The LDI crisis
To hear the full interview, listen in the player above, or download. Future podcasts in our Quantcast series will be uploaded to Risk.net. You can also visit the main page here to access all tracks, or go to the iTunes store, Spotify or Google Podcasts to listen and subscribe.
Only users who have a paid subscription or are part of a corporate subscription are able to print or copy content.
To access these options, along with all other subscription benefits, please contact info@risk.net or view our subscription options here: http://subscriptions.risk.net/subscribe
You are currently unable to print this content. Please contact info@risk.net to find out more.
You are currently unable to copy this content. Please contact info@risk.net to find out more.
Copyright Infopro Digital Limited. All rights reserved.
As outlined in our terms and conditions, https://www.infopro-digital.com/terms-and-conditions/subscriptions/ (point 2.4), printing is limited to a single copy.
If you would like to purchase additional rights please email info@risk.net
Copyright Infopro Digital Limited. All rights reserved.
You may share this content using our article tools. As outlined in our terms and conditions, https://www.infopro-digital.com/terms-and-conditions/subscriptions/ (clause 2.4), an Authorised User may only make one copy of the materials for their own personal use. You must also comply with the restrictions in clause 2.5.
If you would like to purchase additional rights please email info@risk.net
More on Cutting Edge
The WWR in the tail: a Monte Carlo framework for CCR stress testing
A methodology to compute stressed exposures based on a Gaussian copula and mixture distributions is introduced
Estimating mean reversions in interest rate models
The speed of factors’ mean reversion in rate models is estimated
Auto-encoding term-structure models
An arbitrage-free low-dimensionality interest rate model is presented
The relativity of the fractional Gamma Clock
Bank of America quant expands his Gamma Clock model with a fractional Brownian motion
A market-making model for an options portfolio
Vladimir Lucic and Alex Tse fill a glaring gap in European-style derivatives modelling
Option market-making and vol arbitrage
The agent’s view is factored in to a realised-vs-implied vol model
Degree of influence 2024: volatility and credit risk keep quants alert
Quantum-based models and machine learning also contributed to Cutting Edge’s output
Overcoming Markowitz’s instability with hierarchical risk parity
Portfolio optimisation via HRP provides stable and robust weight estimates