Journal of Computational Finance
ISSN:
1460-1559 (print)
1755-2850 (online)
Editor-in-chief: Christoph Reisinger
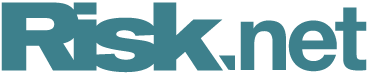
Estimating risks of European option books using neural stochastic differential equation market models
Need to know
- We examine the capacity of an arbitrage-free neural-SDE market model to produce realistic scenarios for the joint dynamics of multiple European options on a single underlying.
- We verify that these neural-SDE market models produce price paths similar to historical data for European equity index markets. We subsequently demonstrate its use as a risk simulation engine for option portfolios.
- Through backtesting analysis, we show that our models are more computationally efficient and accurate for evaluating the Value-at-Risk (VaR) of option portfolios, with better coverage performance and less procyclicality than standard filtered historical simulation approaches.
Abstract
In this paper we examine the capacity of arbitrage-free neural stochastic differential equation market models to produce realistic scenarios for the joint dynamics of multiple European options on a single underlying. We subsequently demonstrate their use as a risk simulation engine for option portfolios. Through backtesting analysis we show that our models are more computationally efficient and accurate for evaluating the value-at-risk of option portfolios than standard filtered historical simulation approaches, with better coverage and less procyclicality.
Copyright Infopro Digital Limited. All rights reserved.
As outlined in our terms and conditions, https://www.infopro-digital.com/terms-and-conditions/subscriptions/ (point 2.4), printing is limited to a single copy.
If you would like to purchase additional rights please email info@risk.net
Copyright Infopro Digital Limited. All rights reserved.
You may share this content using our article tools. As outlined in our terms and conditions, https://www.infopro-digital.com/terms-and-conditions/subscriptions/ (clause 2.4), an Authorised User may only make one copy of the materials for their own personal use. You must also comply with the restrictions in clause 2.5.
If you would like to purchase additional rights please email info@risk.net