Journal of Computational Finance
ISSN:
1460-1559 (print)
1755-2850 (online)
Editor-in-chief: Christoph Reisinger
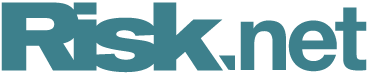
Robust pricing and hedging via neural stochastic differential equations
Patrick Gierjatowicz, Marc Sabate-Vidales, David Šiška, Łukasz Szpruch and Žan Žurič
Need to know
- Modern data science techniques makes data-drive model selection mechanisms easier but the risk of using an inadequate model is hard to quantify.
- The authors aim to learn the drift and diffusion from data using overparameterized neural networks.
- The proposed model, called neural SDE, allows consistent calibration under both risk-neutral and real-world measures and is an instantiation of generative models closely linked with the theory of causal optimal transport.
Abstract
Modern data science techniques are opening the door to data-driven model selection mechanisms. However, most machine learning models are “black boxes”, as individual parameters do not have a meaningful interpretation. In contrast, classical risk models based on stochastic differential equations (SDEs) with fixed parameterization are well understood. Unfortunately, the risk of using an inadequate model is hard to detect and quantify. In this paper, instead of choosing a fixed parameterization for the model SDE, we aim to learn the drift and diffusion from data using overparameterized neural networks. The resulting model, called neural SDE, allows consistent calibration under both risk-neutral and real-world measures and is an instantiation of generative models closely linked with the theory of causal optimal transport. We demonstrate how neural SDEs make it possible to find robust bounds for the prices of derivatives and the corresponding hedging strategies. Further, the model can simulate the market scenarios needed for assessing risk profiles and hedging strategies. We develop and analyze novel algorithms for the efficient use of neural SDEs and we validate our approach with numerical experiments using both market and synthetic data.
Copyright Infopro Digital Limited. All rights reserved.
As outlined in our terms and conditions, https://www.infopro-digital.com/terms-and-conditions/subscriptions/ (point 2.4), printing is limited to a single copy.
If you would like to purchase additional rights please email info@risk.net
Copyright Infopro Digital Limited. All rights reserved.
You may share this content using our article tools. As outlined in our terms and conditions, https://www.infopro-digital.com/terms-and-conditions/subscriptions/ (clause 2.4), an Authorised User may only make one copy of the materials for their own personal use. You must also comply with the restrictions in clause 2.5.
If you would like to purchase additional rights please email info@risk.net