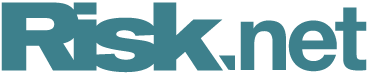
A look at asset liquidation from a different angle
Quants propose a novel approach to assess liquidation cost and stress-testing for hard-to-sell assets
The old way isn’t necessarily the best way. Investment firms traditionally stress-test their assets by calculating how much of a portfolio can be liquidated within a given period of time – two days, or a week, say.
But a new approach flips the orthodoxy on its head. It sets the desired amount of the portfolio to sell, and then aims to find the optimal liquidation time.
Bastien Baldacci, an adviser on the equity derivatives team at HSBC Paris and co-author of the research, argues this is more informative and useful in practice. “This paper aims at providing a quite general framework to answer the basic question ‘how long does it take to me to liquidate X percent of my portfolio, given a schedule, and what are the costs associated to this liquidation?’,” he says.
A schedule is a predefined set of orders that extends over a fixed period of time and can include instructions to process, for example, an equal amount of trades each day or a decreasing amount of trades over time.
The research applies to relatively illiquid assets like corporate bonds, and was initially designed for optimal execution at a UK-based hedge fund. Baldacci conducted the research with co-authors Mike Weber, chief risk officer of UK quantitative asset manager BlueCove, and Iuliia Manziuk, quantitative researcher at Engineers Gate, a US investment firm.
To build their framework, the authors assume that some or all assets in the portfolio follow the so-called locally linear order book (LLOB). The concept of LLOB arises from the assumed pattern in the shape of the trading volume around small deviations of the mid-price. The pattern introduces the notion of latent volume, which is not directly observable. “The latent volumes around the best ticks are linear in price deviation from the best price,” the paper notes.
The authors calculate the density of the book’s trades in a closed-form formula. The idea is to invert that formula and derive the volume that is traded on the market as a function of the price change. “Based on this, one can solve a standard convex optimisation problem and derive the optimal liquidation time,” explains Baldacci.
Baldacci, who, together with Manziuk, was awarded Risk.net rising star in quant finance in 2021 for research on optimal execution across different venues, argues that the assumption on the latent volume is necessary for the model as it would be unrealistic to assume liquidity is fully observable for over-the-counter markets.
In corporate bond markets, liquidity is fragmented. Some bonds can go several days without being traded, while there are single trades that can represent 10 or 20 times the daily average volume.
And for corporate bonds it’s not just the lack of liquidity, but also the lack of fair price. In equity markets, price and bid/ask spread are observable, whereas the corporate bond market operates under a request-for-quote principle, and answers might differ from one to another.
“On OTC markets with fragmented liquidity, this is a good use-case,” says Baldacci.
The long and the short of it
The authors illustrate their method with two stylised examples, one with a long/short portfolio of two bonds of different liquidity and opposite directionality, the other with a long/short portfolio of 20 randomly chosen bonds.
In the first case, the authors note that, in the case of zero correlation between the two bonds, the liquidation times solely depend on their individual liquidity. But as their correlation increases, the liquidation time for both bonds increases and tends to converge.
The portfolio of 20 bonds has a similar outcome, where the liquidation time tends to be higher for higher levels of correlations.
Conversely, in both cases the cost of liquidation decreases with the increase of correlation among securities, as an effect of the longer liquidation time.
Once the methodology is established, using it for stress-testing is relatively straightforward. The values of all the relevant parameters – average daily volume of all assets, volatility and bid/ask spread – are multiplied by given stress coefficients. Recalculating the cost to liquidate the portfolio under those conditions provides the liquidation time in a stressed scenario.
The scenario loss, the impact of the stressed condition on liquidation, is the difference between the stressed scenario liquidation cost and the unstressed scenario liquidation cost.
The approach doesn’t apply to derivatives because it doesn’t take cross-asset impact into account, says Baldacci, but he and other co-authors have been working on a different method to solve optimal trading problems while allowing for general cross-impact matrices, and the results are forthcoming in Risk.net soon.
Only users who have a paid subscription or are part of a corporate subscription are able to print or copy content.
To access these options, along with all other subscription benefits, please contact info@risk.net or view our subscription options here: http://subscriptions.risk.net/subscribe
You are currently unable to print this content. Please contact info@risk.net to find out more.
You are currently unable to copy this content. Please contact info@risk.net to find out more.
Copyright Infopro Digital Limited. All rights reserved.
As outlined in our terms and conditions, https://www.infopro-digital.com/terms-and-conditions/subscriptions/ (point 2.4), printing is limited to a single copy.
If you would like to purchase additional rights please email info@risk.net
Copyright Infopro Digital Limited. All rights reserved.
You may share this content using our article tools. As outlined in our terms and conditions, https://www.infopro-digital.com/terms-and-conditions/subscriptions/ (clause 2.4), an Authorised User may only make one copy of the materials for their own personal use. You must also comply with the restrictions in clause 2.5.
If you would like to purchase additional rights please email info@risk.net
More on Views
UBS’s Iabichino holds a mirror to bank funding risks
Framing funding management as an optimal control problem affords an alternative to proxy hedging
Podcast: Alexei Kondratyev on quantum computing
Imperial College London professor updates expectations for future tech
Quants mine gold for new market-making model
Novel approach to modelling cointegrated assets could be applied to FX and potentially even corporate bond pricing
Quants dive into FX fixing windows debate
Longer fixing windows may benefit clients, but predicting how dealers will respond is tough
Podcast: Piterbarg and Nowaczyk on running better backtests
Quants discuss new way to extract independent samples from correlated datasets
BofA quants propose new model for when to hold, when to sell
Closed-form formula helps market-makers optimise exit strategies
Podcast: Alvaro Cartea on collusion within trading algos
Oxford-Man Institute director worries ML-based trading could have anti-competitive effects
Podcast: Lorenzo Ravagli on why the skew is for the many
JP Morgan quant proposes a unified framework for trading the volatility skew premium