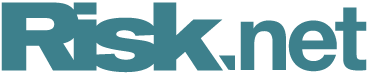
What quant finance can learn from a 240-year-old problem
Optimal transport theory offers a data-driven way to calibrate derivatives pricing models
At first glance, the delivery of building materials to construction sites might not seem to have much in common with the calibration of derivatives pricing models. However, both problems can be solved with the same methodology.
Optimal transport theory was introduced in the 18th century by Gaspard Monge, a French geometer and mathematician, who was looking for an efficient way to move soil between building sites. The theory has been developed by several other mathematicians and was solved only towards the end of the 20th century. Today, it is used in several fields, ranging from topology to image processing.
In simple terms, optimal transport theory is used to measure the distance between two distributions. This can be useful for finance in a number of contexts – one of them being model calibration.
The traditional approach to calibration involves selecting a suitable model to price a given instrument and then finding its optimal parametrisation. “Optimal transport allows us to address the same calibration problem, but in a fully nonparametric way,” says Jan Obloj, professor of mathematics at the University of Oxford.
When viewed through an optimal transport lens, the problem boils down to minimising the distance between a given model, which is constrained to calibrate perfectly, and a reference model. This can be thought of as essentially morphing one distribution into another.
Obloj offers an illustration: “We have our favourite model, A, but we need to have a calibrated one, so we want to change A into some model B, which will match the market data, but we want to make this efficiently without making unnecessary changes.”
Obloj has worked with Ivan Guo, Gregoire Loeper and Shiyi Wang at Monash University in Melbourne to develop calibration solutions for common pricing models and derivatives products. In a series of papers, the latest of which was published in Risk.net, they apply optimal transport theory to local and local-stochastic volatility models and the pricing of path-dependent options.
Their optimal transport methodology calibrates the market data by taking a reference model – in this case, the Heston model – and deviating from it until it finds the perfect calibration. The resulting model is likely to be close to the reference model but also different enough that it will not be possible to formulate it with the same equations.
The latest paper in the series deals with the joint calibration of S&P 500 and Vix options – a problem that has only recently been solved with classic, parametric approaches. With optimal transport, the calibration can be performed with very few model assumptions.
Their optimal transport methodology calibrates the market data by taking a reference model – in this case, the Heston model – and deviating from it until it finds the perfect calibration
One of the advantages of optimal transport is that, unlike traditional approaches that start with a parametric family of models and then calibrate to them, it can consider all models, or a broad category – such as all Markovian models – when performing calibrations.
This means it is extremely versatile and can even be used to price instruments that do not have a satisfactory dedicated pricing model. “One can calibrate to all sorts of instruments that otherwise are hard to accommodate within a parametric model,” says Obloj. It is also possible to update the calibration relatively easily as new data materialises, ensuring the model evolves with the relevant market data.
Some features of optimal transport may be reminiscent of deep learning, but there are fundamental differences. While both approaches are data-driven, deep learning is essentially a nonlinear regression and requires a large training set to learn, whereas the optimal transport calibration methodology starts with a learned guess, the reference model, and evolves it so that it matches the market constraints perfectly.
The downside is that optimal transport methodologies are computationally intensive. Optimising in infinite dimensional spaces is a non-trivial exercise, and while the authors have shown this can be overcome, it will take more computing time than a classical calibration over, say, five parameters.
That might rule it out for front-office applications, where speed is paramount. But for less urgent tasks, optimal transport might prove to be a valuable tool in the quant’s arsenal.
“It is certainly a method that could become a common use for overnight calculations to validate a model or for robustness checks,” says Obloj. “I think it’s a very powerful tool. It also naturally delivers insights into where the real risks are.”
He also sees other potential applications in risk management. “In particular, [martingale] optimal transport allows us to compute the range of no-arbitrage prices for OTC products which are compatible with market prices of exchange-traded products. The optimal transport methodology also leads to a non-parametric version of the classical Greeks, capturing risk in a more comprehensive fashion.”
Only users who have a paid subscription or are part of a corporate subscription are able to print or copy content.
To access these options, along with all other subscription benefits, please contact info@risk.net or view our subscription options here: http://subscriptions.risk.net/subscribe
You are currently unable to print this content. Please contact info@risk.net to find out more.
You are currently unable to copy this content. Please contact info@risk.net to find out more.
Copyright Infopro Digital Limited. All rights reserved.
As outlined in our terms and conditions, https://www.infopro-digital.com/terms-and-conditions/subscriptions/ (point 2.4), printing is limited to a single copy.
If you would like to purchase additional rights please email info@risk.net
Copyright Infopro Digital Limited. All rights reserved.
You may share this content using our article tools. As outlined in our terms and conditions, https://www.infopro-digital.com/terms-and-conditions/subscriptions/ (clause 2.4), an Authorised User may only make one copy of the materials for their own personal use. You must also comply with the restrictions in clause 2.5.
If you would like to purchase additional rights please email info@risk.net
More on Views
Quants mine gold for new market-making model
Novel approach to modelling cointegrated assets could be applied to FX and potentially even corporate bond pricing
Quants dive into FX fixing windows debate
Longer fixing windows may benefit clients, but predicting how dealers will respond is tough
Podcast: Piterbarg and Nowaczyk on running better backtests
Quants discuss new way to extract independent samples from correlated datasets
BofA quants propose new model for when to hold, when to sell
Closed-form formula helps market-makers optimise exit strategies
Podcast: Alvaro Cartea on collusion within trading algos
Oxford-Man Institute director worries ML-based trading could have anti-competitive effects
Podcast: Lorenzo Ravagli on why the skew is for the many
JP Morgan quant proposes a unified framework for trading the volatility skew premium
Counterparty risk model links defaults to portfolio values
Fed’s Michael Pykhtin proposes using copula models to capture effects of margin calls on default risk
Podcast: Olivier Daviaud on P&L attribution for options
JP Morgan quant discusses his alternative to Greeks decomposition