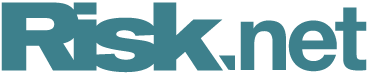
Estimating loan loss provisions may have just got easier
Commerzbank quant proposes shortcut to calculate lifetime loan loss reserves
New accounting standards and stress-testing exercises introduced in recent years have presented banks with a puzzle: how to regularly estimate the necessary reserves for expected lifetime losses on loans without breaking the bank. A computational challenge of this kind needs to be reduced in scale in order to cut its cost.
Michael Winands, quantitative analyst in the department of model validation at Commerzbank, says he has found a way to do exactly that. Instead of estimating each loan’s expected loss for all its potential future ratings, he proposes taking the lifetime expected credit loss for a single rating and a single type of collateralisation and deriving the lifetime expected credit loss for other ratings and collateralisations from those.
In his October paper Approximating lifetime expected credit loss, Winands suggests using stylised “elementary”, or standard, loans as the starting point.
“My main finding is that most loans, in terms of lifetime expected credit loss calculations, are very similar to one another and they can be matched to very elementary loans,” he says. “So, once you calculate the lifetime expected credit loss of very stylised loans for different rating grades, then you can use these expected loan losses results to approximate a broader range of loans.”
Banks’ computational burden increased when the International Financial Reporting Standard 9 and the US Current Expected Credit Loss accounting standard came into force, introducing tougher requirements for the calculation of credit loss provisions.
Under IFRS 9, banks must have enough reserves to cover 12 months of expected credit losses for performing loans and, in a break with previous rules, lifetime losses for all other loans. Under CECL, the entire loan book is treated as if it contains nothing but riskier loans and so, expected credit loss provisions must cover the life of the assets.
Moreover, as part of regular stress tests by regulators, large European Union and US banks must project these losses and reserves under different macroeconomic scenarios.
My presumption is that most of the banks don’t have a very sophisticated approximation method and are likely to look for more robust methods
Michael Winands, Commerzbank
If approached with brute force, the lifetime requirement means simulating large numbers of possible loan realisations, under each possible rating – since a loan’s quality may change during its lifetime – for each loan in a portfolio in order to calculate the expected credit loss, and, based on that, the loss provision.
The approximation technique proposed by Winands drastically reduces the number of times this computationally expensive task needs to be performed.
“My presumption is that most of the banks don’t have a very sophisticated approximation method and are likely to look for more robust methods,” he says.
Under his approach, all standard loans are put into two broad categories: those with linear amortisation – mortgages would be an example – and those with a single repayment, or bullet loans. Each category is represented by a single loan.
The next step is, for each representative loan, to estimate lifetime expected losses for different possible ratings and for an upward or downward evolution in the value of the collateral.
Based on this calculation, Winands has developed a table for each category that indicates the expected loss for each rating and maturity. So instead of recalculating a loan’s expected loss from scratch, it can be simply looked up in the corresponding table, depending on whether the loan is closer in type to the first category of loans or the second. Once all expected losses are estimated, it is easy to aggregate them and work out the necessary loan loss provisions.
The underlying complex calculation is updated only periodically – for instance, monthly.
This method shows out-of-the-box thinking. Innovative approaches like this one are particularly valuable in back- and middle-office functions where things tend to be more conservative
Dariusz Gątarek, Polish Academy of Sciences
Dariusz Gątarek, professor of financial engineering at the Polish Academy of Sciences and an experienced credit quant, sees merit in the approach.
“This method shows out-of-the-box thinking. Innovative approaches like this one are particularly valuable in back- and middle-office functions where things tend to be more conservative and move more slowly,” he says.
He also notes: “Regulatory requirements that affect internal technical procedures often are not intellectually challenging but they are computationally difficult.”
Indeed, previously, estimating a loan provision was relatively straightforward, because in mathematical terms it had a linear relationship with the probability of default (PD).
“We usually had to estimate the expectation of the future stressed PD of a loan and with that we could easily calculate the future loan provision,” Winands says.
But to forecast loan loss provisions in compliance with IFRS 9, all potential future ratings for the loan, along with the probability of each rating, must be considered.
Say, the 21 ratings on Moody’s scale are used. In this case, calculating a future loss provision means taking each of those ratings, weighting them by probability in line with a migration matrix and then calculating the loan loss provision for each potential rating. For very poor ratings, PD term structure, collateral path and amortisation schedule must also be fully considered. As a result, the calculation of expected losses requires a lot of data and a lot of run time, so the less frequently it has to be done, the better.
The methodology devised by Winands – developed when his model validation team was looking for a challenger model – works only for standard loans. But, in his view, that is already a significant step forward.
“This methodology might not work for loans with unusual or complicated amortisation structures, but I think it can deal with most loans, and the two categories exemplified in the paper comprise the vast majority of loans,” he says.
Editing by Olesya Dmitracova
Only users who have a paid subscription or are part of a corporate subscription are able to print or copy content.
To access these options, along with all other subscription benefits, please contact info@risk.net or view our subscription options here: http://subscriptions.risk.net/subscribe
You are currently unable to print this content. Please contact info@risk.net to find out more.
You are currently unable to copy this content. Please contact info@risk.net to find out more.
Copyright Infopro Digital Limited. All rights reserved.
As outlined in our terms and conditions, https://www.infopro-digital.com/terms-and-conditions/subscriptions/ (point 2.4), printing is limited to a single copy.
If you would like to purchase additional rights please email info@risk.net
Copyright Infopro Digital Limited. All rights reserved.
You may share this content using our article tools. As outlined in our terms and conditions, https://www.infopro-digital.com/terms-and-conditions/subscriptions/ (clause 2.4), an Authorised User may only make one copy of the materials for their own personal use. You must also comply with the restrictions in clause 2.5.
If you would like to purchase additional rights please email info@risk.net
More on Views
Getting a handle on model parameters
Mean reversion in rate parameters opens the door to dimensionality reduction
Podcast: adventures in autoencoding
Trio of senior quants explain how autoencoders can reduce dimensionality in yield curves
Podcast: Lyudmil Zyapkov on the relativity of volatility
BofA quant’s new volatility model combines gamma processes and fractional Brownian motion
A market-making model for an options portfolio
Vladimir Lucic and Alex Tse fill a glaring gap in European-style derivatives modelling
Degree of influence 2024: volatility and credit risk keep quants alert
Quantum-based models and machine learning also contributed to Cutting Edge’s output
Podcast: Alexandre Antonov turns down the noise in Markowitz
Adia quant explains how to apply hierarchical risk parity to a minimum-variance portfolio
UBS’s Iabichino holds a mirror to bank funding risks
Framing funding management as an optimal control problem affords an alternative to proxy hedging
Podcast: Alexei Kondratyev on quantum computing
Imperial College London professor updates expectations for future tech