Journal of Operational Risk
ISSN:
1744-6740 (print)
1755-2710 (online)
Editor-in-chief: Marcelo Cruz
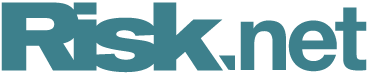
Cyber risk definition and classification for financial risk management
Filippo Curti, Jeffrey Gerlach, Sophia Kazinnik, Michael Lee and Atanas Mihov
Need to know
- Cyber risk is a critical emerging risk to the financial industry that poses a significant threat to financial stability.
- The lack of proper data on cyber risk losses impedes efforts to effectively measure and manage this risk.
- The paper proposes a cyber risk definition and classification scheme for risk management purposes that financial institutions can use as a data collection template.
- The proposed scheme ensures that adopting institutions are utilizing common language, allowing for consistent data collection and sharing, and maps existing cybersecurity events into the scheme.
Abstract
Cyber risk is undeniably one of the most critical emerging risks to the financial industry. However, even though cyber risk is recognized as a significant threat to financial institutions and, more generally, to financial stability, the lack of proper data on cyber risk losses impedes efforts to effectively measure and manage this risk. This paper aims to address this gap by providing a cyber risk definition and classification scheme for risk management purposes, to be used as a data collection template for financial institutions. As such, the proposed scheme would ensure that the adopting institutions utilize common language and would allow consistent data collection and sharing.We provide a deeper dive into the reasoning behind the variables we propose to collect and demonstrate how some of the existing cyber security events map into our proposed scheme.
Copyright Infopro Digital Limited. All rights reserved.
You may share this content using our article tools. Printing this content is for the sole use of the Authorised User (named subscriber), as outlined in our terms and conditions - https://www.infopro-insight.com/terms-conditions/insight-subscriptions/
If you would like to purchase additional rights please email info@risk.net
Copyright Infopro Digital Limited. All rights reserved.
You may share this content using our article tools. Copying this content is for the sole use of the Authorised User (named subscriber), as outlined in our terms and conditions - https://www.infopro-insight.com/terms-conditions/insight-subscriptions/
If you would like to purchase additional rights please email info@risk.net