Journal of Operational Risk
ISSN:
1744-6740 (print)
1755-2710 (online)
Editor-in-chief: Marcelo Cruz
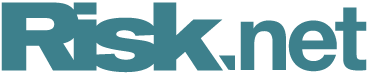
Need to know
- We investigate whether the inclusion of past operational losses improves performance of operational risk models and find that it does even when accounting for a wide range of controls.
- Information provided by past losses likely results from them capturing hard to quantify factors such as the quality of operational risk controls, the risk culture and the risk appetite of the bank.
- Past losses are predictive of future losses for all operational loss event types.
Abstract
Operational risk is a substantial source of risk for US banks. Improving the performance of operational risk models allows banks’ management to make more informed risk decisions by better matching economic capital and risk appetite, and it allows regulators to enhance their understanding of banks’ operational risk. We show that past operational losses are informative of future losses, even after controlling for a wide range of financial characteristics. We propose that the information provided by past losses results from their capturing hard-to-quantify factors such as the quality of operational risk controls, the risk culture and the risk appetite of the bank.
Copyright Infopro Digital Limited. All rights reserved.
As outlined in our terms and conditions, https://www.infopro-digital.com/terms-and-conditions/subscriptions/ (point 2.4), printing is limited to a single copy.
If you would like to purchase additional rights please email info@risk.net
Copyright Infopro Digital Limited. All rights reserved.
You may share this content using our article tools. As outlined in our terms and conditions, https://www.infopro-digital.com/terms-and-conditions/subscriptions/ (clause 2.4), an Authorised User may only make one copy of the materials for their own personal use. You must also comply with the restrictions in clause 2.5.
If you would like to purchase additional rights please email info@risk.net