Journal of Operational Risk
ISSN:
1744-6740 (print)
1755-2710 (online)
Editor-in-chief: Marcelo Cruz
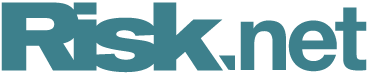
A weighted likelihood estimator for operational risk data: improving the accuracy of capital estimates by robustifying maximum likelihood estimates
Andrea Colombo, Alessandro Lazzarini and Silvia Mongelluzzo
Need to know
- The non - robust MLE can produce unstable and unrealistic OpRisk capital estimates
- The proposed estimator represents a simple and robust generalization of MLE
- It allows to reduce the upward bias in the capital and to get more stable results
Abstract
ABSTRACT
Severity parameters play a crucial role in the operational risk (OpRisk) capital estimates for Advanced Measurement Approach banks. When relying on maximum likelihood estimates (MLEs), nonrobustness to small deviations from the standard regularity conditions and to the inclusion of single data points challenges the stability of the capital estimates severely. We propose the use of a robust generalization of MLEs for the modeling of operational loss data. The weighted likelihood estimator we propose is a special case of the estimator developed by Choi, Hall and Presnel in 2000, and it is robust yet still consistent and efficient.We describe its basic features, discussing its main theoretical properties and how to calibrate the estimation procedure. Finally, using both simulation studies and real data, we show how use of the weighted likelihood estimator can improve the stability of OpRisk capital estimates. The proposed approach generally allows one to reduce the upward capital bias and to obtain capital estimates that are more stable under contamination or with isolated data.
Copyright Infopro Digital Limited. All rights reserved.
As outlined in our terms and conditions, https://www.infopro-digital.com/terms-and-conditions/subscriptions/ (point 2.4), printing is limited to a single copy.
If you would like to purchase additional rights please email info@risk.net
Copyright Infopro Digital Limited. All rights reserved.
You may share this content using our article tools. As outlined in our terms and conditions, https://www.infopro-digital.com/terms-and-conditions/subscriptions/ (clause 2.4), an Authorised User may only make one copy of the materials for their own personal use. You must also comply with the restrictions in clause 2.5.
If you would like to purchase additional rights please email info@risk.net