Journal of Credit Risk
ISSN:
1744-6619 (print)
1755-9723 (online)
Editor-in-chief: Linda Allen and Jens Hilscher
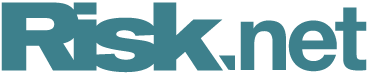
Customer churn prediction for commercial banks using customer-value-weighted machine learning models
Need to know
- We propose a cost sensitive framework for churn prediction.
- Customer values are taken as misclassification costs.
- The value index is calculated by recency, frequency, monetary and asset behaviors of credit card customers.
- Five machine learning models are used for prediction and comparison.
Abstract
Customer churn prediction has become an increasingly important issue in global business, especially in the banking industry, where customer acquisition has become ever more costly in this notoriously competitive business environment. Although many methods have been proposed to solve this issue as a classification problem, there are few studies that consider customer values in the light of attrition analysis. In this paper, we propose a framework to address this, and we quantify customer values with the use of an improved customer value model, examining them from the perspective of their recency, frequency, monetary value and asset level. We take customer values as the basis of misclassification costs that, in turn, direct machine learning predictive models. The returns for banks in this scenario can be maximized, given various cutoffs and some assumptions. This proposed framework may provide commercial banks with useful insights to better formulate marketing strategies for different groups of customers, as well as to analyze attrition in an economic way, rather than as a simple classification problem.
Copyright Infopro Digital Limited. All rights reserved.
As outlined in our terms and conditions, https://www.infopro-digital.com/terms-and-conditions/subscriptions/ (point 2.4), printing is limited to a single copy.
If you would like to purchase additional rights please email info@risk.net
Copyright Infopro Digital Limited. All rights reserved.
You may share this content using our article tools. As outlined in our terms and conditions, https://www.infopro-digital.com/terms-and-conditions/subscriptions/ (clause 2.4), an Authorised User may only make one copy of the materials for their own personal use. You must also comply with the restrictions in clause 2.5.
If you would like to purchase additional rights please email info@risk.net