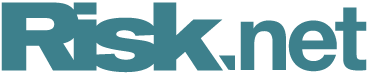
Counterparty risk model links defaults to portfolio values
Fed’s Michael Pykhtin proposes using copula models to capture effects of margin calls on default risk
A rigorous approach to determining the credit risk posed by highly leveraged counterparties is not something that is easy to develop and implement.
One reason for this is the scarcity of information. Dealers do not see what positions a counterparty has with other dealers, and cannot reliably assess the levels of concentration, leverage and wrong-way risk associated with that counterparty.
Another reason is dealers’ fear of losing business. A conservative approach that assumes worse-case scenarios and that requires a commensurately high level of collateral would quickly become too expensive and push clients to take their business elsewhere.
The decision to close the tap on a counterparty may not be based on a bespoke quantitative model. It might instead rely on inadequate models or come from the intuition that a big hole has opened underneath a derivatives portfolio. Such approaches are dangerous, as they might lead to a severe miscalculation of the frequency and size of credit events.
Since the collapse of Archegos in March 2021, various solutions have been put forward to address the issue. These include proposals by the Bank of England’s Fabrizio Anfuso, in which the counterparty’s balance sheet is taken into account, and JP Morgan’s Matthias Arnsdorf, whose approach focuses on modelling the expected exposure (EE).
Most credit models struggle to capture the dependency between default risk and portfolio value. However, a recent paper by Michael Pykhtin, manager in the policy research and analytics function at the Federal Reserve Board in Washington, DC, aims to fill this gap. His model can produce higher credit exposure in the cases where that dependency is higher, thereby reflecting the greater riskiness of leveraged counterparties.
Pykhtin’s model formalises a link between a counterparty’s default event and the incremental change in its portfolio’s value over the margin period of risk. The point is to capture a dealer’s ballooning exposure to a client as the values of derivatives contracts fall, and to correlate this with the chances of the counterparty defaulting.
The link is established with a Gaussian copula – a statistical tool to model the co-dependency between two random variables. Its correlation parameter effectively regulates the level of leveraged wrong-way risk.
Michael Pykhtin’s model can produce higher credit exposure in the cases where dependency is higher, thereby reflecting the greater riskiness of leveraged counterparties
This risk is a by-product of the variation margin that the counterparty posts as collateral. When the value of the counterparty’s portfolio increases, the dealer requires additional margin. The counterparty then has to quickly find the necessary liquidity, which can place it under financial stress. If it receives multiple margin calls from different dealers, it will be much more likely to default because it will be unable to meet them. Archegos has become a textbook example of such a chain of events.
Despite the scarcity of information, Pykhtin’s model may be used to account for the possibility that a counterparty holds similarly leveraged positions with multiple dealers, as was the case with Archegos. A higher value of the correlation parameter could signify an exposure spread across various dealers, with the leverage level pulling the counterparty perilously towards default when markets move adversely.
Theory and practice
Ben Burnett, head of Barclays’ XVA quant team, praises the “practicality” of Pykhtin’s approach: “He makes the point that this can be fitted relatively naturally into a standard exposure simulation system, like those used in XVA and counterparty risk.”
For Pykhtin, the advantage of his method is that it can be applied on top of existing exposure models without the need to modify the simulation frameworks. The method replaces the simple average used to calculate expected exposure from simulated scenarios with a weighted average – greater weights are applied to scenarios in which the impact on the incremental change in portfolio value is greater and where a default is more likely.
However, Burnett is cautious about the model’s applicability. “A key part of it is the correlation,” he says. “Pykhtin uses a Gaussian copula, and the difficult question is how you choose that correlation. Calibration of that is a very difficult thing.
“How many Archegoses have we had? And how many times have you seen the default for the counterparty that you’re currently running?”
How easily Pykhtin’s model can be plugged into an existing system will depend on that system’s architecture. “I think it will very much vary, depending on how a given institution has its exposure model set up,” says Burnett.
One of the practical difficulties involves the simulation of paths to calculate the potential exposure. The number of necessary paths could be so great as to make the calculation challenging, although Burnett says approaches can be taken to make use of Pykhtin’s model even in those sorts of extreme scenarios.
Pykhtin’s paper also extends the scaling technique for expected exposure – used for calculating EE in the presence of initial margin (IM) without calculating future IM requirements – to the case of leveraged wrong-way risk, thereby building on his previous work with Leif Andersen and Alexander Sokol.
Pykhtin has been an influential quant researcher on credit risk models for more than two decades, and was named Risk.net’s Quant of the year not once but twice. It is common for ideas in this field to be clever but hard for banks to implement. However, Pykhtin has always shown a keen eye for solutions that can be implemented in production.
Burnett says the pragmatism of Pykhtin’s approach means it has the potential to reach the production stage. That would provide dealers with a quantitative tool to add to their business intuition, and better enable them to mitigate the consequences of adverse events.
Editing by Daniel Blackburn
Only users who have a paid subscription or are part of a corporate subscription are able to print or copy content.
To access these options, along with all other subscription benefits, please contact info@risk.net or view our subscription options here: http://subscriptions.risk.net/subscribe
You are currently unable to print this content. Please contact info@risk.net to find out more.
You are currently unable to copy this content. Please contact info@risk.net to find out more.
Copyright Infopro Digital Limited. All rights reserved.
As outlined in our terms and conditions, https://www.infopro-digital.com/terms-and-conditions/subscriptions/ (point 2.4), printing is limited to a single copy.
If you would like to purchase additional rights please email info@risk.net
Copyright Infopro Digital Limited. All rights reserved.
You may share this content using our article tools. As outlined in our terms and conditions, https://www.infopro-digital.com/terms-and-conditions/subscriptions/ (clause 2.4), an Authorised User may only make one copy of the materials for their own personal use. You must also comply with the restrictions in clause 2.5.
If you would like to purchase additional rights please email info@risk.net
More on Views
UBS’s Iabichino holds a mirror to bank funding risks
Framing funding management as an optimal control problem affords an alternative to proxy hedging
Podcast: Alexei Kondratyev on quantum computing
Imperial College London professor updates expectations for future tech
Quants mine gold for new market-making model
Novel approach to modelling cointegrated assets could be applied to FX and potentially even corporate bond pricing
Quants dive into FX fixing windows debate
Longer fixing windows may benefit clients, but predicting how dealers will respond is tough
Podcast: Piterbarg and Nowaczyk on running better backtests
Quants discuss new way to extract independent samples from correlated datasets
BofA quants propose new model for when to hold, when to sell
Closed-form formula helps market-makers optimise exit strategies
Podcast: Alvaro Cartea on collusion within trading algos
Oxford-Man Institute director worries ML-based trading could have anti-competitive effects
Podcast: Lorenzo Ravagli on why the skew is for the many
JP Morgan quant proposes a unified framework for trading the volatility skew premium