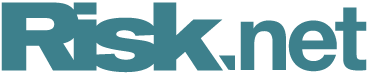
This article was paid for by a contributing third party.More Information.
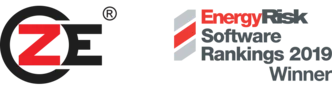
The advance of analytics
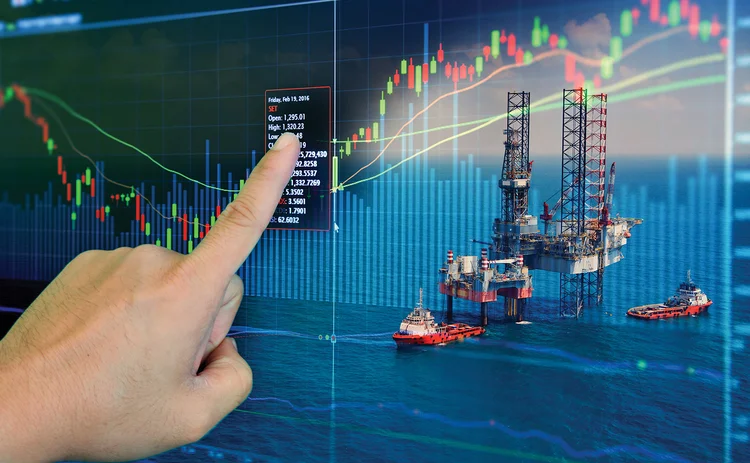
Machine learning is coming to analytics but there are hurdles to overcome first, says Aiman El‑Ramly, chief operations officer at ZE PowerGroup
The sophistication of energy firms’ analytics has increased substantially in recent years, thanks to a ramp-up in available data, increased computational power through cloud computing and a hike in statistical power through the integration of offerings such as the open-source language R. The next stage in the race to gain a competitive advantage will involve applying machine learning to data, says Aiman El‑Ramly, chief operations officer at ZE PowerGroup – a data software and consulting firm that specialises in energy and commodities. The firm, which launched its ZEMA data integration and analytics platform 20 years ago, provides across-the-board commodities data and helps firms achieve their analytics goals.
While machine learning offers huge potential for analytics development, it is fraught with challenges at this stage, says El‑Ramly.

What is the long-term vision for machine learning and data analytics?
Aiman El‑Ramly: Machine learning can supplement the data management process by providing unique insights into patterns that may lay hidden in data. Using data enrichment techniques, trading companies can look longitudinally at the data they have collected over the years to discover patterns and relationships in the past to help predict future outcomes within a band of confidence. While analysts have been undertaking this type of analysis for years to support trading and risk management strategies, machine learning technology offers abilities in speed, volume, complexity and variation that is difficult to replicate without immense effort and cost. As analysts have learned, they can work to enhance the adequacy of the strategy by iterating, adapting and adding new machine algorithms.
What are the greatest challenges when it comes to using machine learning for analytics?
Aiman El‑Ramly: The biggest challenge for machine learning technology has existed since the advent of computing. Data collection, validation, staging and transformation are integral to the success of any desired machine enhancement. Machine learning technology requires a predictable format across a vast array of data to be effective. In the absence of a workable expanse of data, machine results will be erroneous or unavailable. It is a challenge people often dismiss but, if they don’t deal effectively with the data, all downstream machine results will either be wrong or not statistically significant. Without fully allowing for the structure of the underlying data and all of its attributes, it won’t matter what questions are being asked, the results will be erroneous.
Is there a science behind asking the right question?
Aiman El‑Ramly: Getting the question right is very difficult. For example, if you want to find out whether there is a correlation between falling power prices and rain in a particular region, it may not be sufficient to say: ‘Show me all the occasions when it rained and power prices fell to x level.’ You might need to add more factors, such as volume of rain, temperature, hydro levels, and so on. Otherwise, you may find a correlation, but not a causation, or one that’s really secondary to your analysis. You might find prices fall, on average, when it rains, but if you don’t realise the fall only occurs when it rains within a certain condition, you could execute a strategy based on the rainfall alone. But the more important variable might be another condition such as volume of rain or hydro levels or something seemingly unconnected such as how much wind is blowing. You can have too many variables as well as too few. Finding the magic in the middle is not easy. You have to ask a lot of questions before you find the right one. There’s a lot of testing and regression analysis needed to determine whether the question is valid. There’s no firm science on it yet.
What are energy firms typically using machines for at the moment?
Aiman El‑Ramly: Currently the work is on using machine learning capabilities to develop enhanced data. ZEMA could support a firm’s machine learning analytics project by providing enhanced data and relaying what level of confidence we believe you should have in the underlying results.
If you don’t have the data – the enormous amount of records required – or it hasn’t been validated and structured properly, you can’t undertake predictive analytics. ZE has a fantastic advantage in the energy and commodities market in that it has data dating back decades in some cases. That can, however, bring its own challenges. You can’t perform a machine learning process if each point of data isn’t properly attributed with its full meaning. For example, imagine a data point at 28.60: you need to know what commodity it represents – whether it is for a particular grade or product, the units of measure, when it was measured, and so on – but also who published it, for what purpose and how that relates to other sources of data. It could be that the names of markets or hubs have changed over time or a location is no longer valid. If the data has not been attributed correctly from the beginning, you can’t perform a meaningful analysis. ZE has understood from the start that a data point has many factors to consider, so it built its parsers – data collectors – from day one with the idea of including attributes.
How useful do you think social media and other unstructured data is?
Aiman El‑Ramly: I think sentiment analysis – going out into the world of social media and combing through it for market indicators – is fraught with folly. Just look at the wildly inaccurate predictions of the 2016 US presidential election and Brexit. You can collect sentiment data but, unless you understand the psychology of the data – which is almost impossibly complex to model, and is influenced by things such as uncertainty, fear or gaming – you can quickly find yourself in trouble.
What ZE finds more fruitful is monitoring chat mechanisms, such as those provided by the Intercontinental Exchange or various brokers that like-minded people are using to communicate market variables – for example, bids, offers and price quotes. ZE collects this information and produces curves or executable products that enable decisions. ZE quickly integrates with external or internal systems to extract data from these formalised chats to create corporate-specific data streams that create high value for executing trade strategy.
When do you expect a meaningful advance in machine learning and analytics?
Aiman El‑Ramly: It is very early days. There are not many people that know how to perform machine learning and predictive analytics in a real way for a specific commodity. The required corporate resources at most firms are limited. No vendor firms have yet developed a plug-and-play machine analysis package. Viable products may be rolled out in the next five years or so. However, we’re a dozen years away from substantive use of what we’re envisioning for machine learning. University students are currently learning the science; they will then need a few years in the field to specialise.
Will data science students be attracted to the energy industry?
Aiman El‑Ramly: We will see today’s students migrating to a new class of industry that does not yet exist – a sort of machine learning service where experts apply machines to your industry. I think this new class of business could arrive in the next 10 years.
Sponsored content
Copyright Infopro Digital Limited. All rights reserved.
As outlined in our terms and conditions, https://www.infopro-digital.com/terms-and-conditions/subscriptions/ (point 2.4), printing is limited to a single copy.
If you would like to purchase additional rights please email info@risk.net
Copyright Infopro Digital Limited. All rights reserved.
You may share this content using our article tools. As outlined in our terms and conditions, https://www.infopro-digital.com/terms-and-conditions/subscriptions/ (clause 2.4), an Authorised User may only make one copy of the materials for their own personal use. You must also comply with the restrictions in clause 2.5.
If you would like to purchase additional rights please email info@risk.net