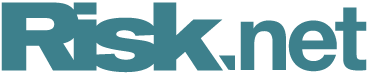
Using transaction data to measure op risk
Since 1999, Peter Hughes has led a team of op risk specialists that has studied transaction processing environments in global banking organisations. The focus was on examining and understanding the causes of operational failure. The information and benchmark data gathered was used to develop a method that provides consistent and comparable measures of exposure and probability of failure in transaction processing environments. Hughes outlines the method that resulted from these studies
After
more than five years of research and consultation, the Basel Committee on Banking Supervision has formally issued the new capital Accord, Basel II. Its aim is twofold. First, to have the financial services industry determine its capital requirement through the application of more sophisticated internal credit risk models. Second, to achieve a similar degree of sophistication with operational risk.The challenge on the operational risk side has turned out to be vastly more complex and
Only users who have a paid subscription or are part of a corporate subscription are able to print or copy content.
To access these options, along with all other subscription benefits, please contact info@risk.net or view our subscription options here: http://subscriptions.risk.net/subscribe
You are currently unable to print this content. Please contact info@risk.net to find out more.
You are currently unable to copy this content. Please contact info@risk.net to find out more.
Copyright Infopro Digital Limited. All rights reserved.
As outlined in our terms and conditions, https://www.infopro-digital.com/terms-and-conditions/subscriptions/ (point 2.4), printing is limited to a single copy.
If you would like to purchase additional rights please email info@risk.net
Copyright Infopro Digital Limited. All rights reserved.
You may share this content using our article tools. As outlined in our terms and conditions, https://www.infopro-digital.com/terms-and-conditions/subscriptions/ (clause 2.4), an Authorised User may only make one copy of the materials for their own personal use. You must also comply with the restrictions in clause 2.5.
If you would like to purchase additional rights please email info@risk.net
More on Operational risk
Integrated GRC solutions 2024: market update and vendor landscape
In the face of persistent digitisation challenges and the attendant transformation in business practices, many firms have been struggling to maintain governance and business continuity
Vendor spotlight: Dixtior AML transaction monitoring solutions
The Chartis Research report, AML transaction monitoring solutions, considers how, by working together, financial institutions, vendors and regulators can create more effective anti-money laundering (AML) systems.
Financial crime and compliance50 2024
The detailed analysis for the Financial crime and compliance50 considers firms’ technological advances and strategic direction to provide a complete view of how market leaders are driving transformation in this sector
Automating regulatory compliance and reporting
Flaws in the regulation of the banking sector have been addressed initially by Basel III, implemented last year. Financial institutions can comply with capital and liquidity requirements in a natively integrated yet modular environment by utilising…
Investment banks: the future of risk control
This Risk.net survey report explores the current state of risk controls in investment banks, the challenges of effective engagement across the three lines of defence, and the opportunity to develop a more dynamic approach to first-line risk control
Op risk outlook 2022: the legal perspective
Christoph Kurth, partner of the global financial institutions leadership team at Baker McKenzie, discusses the key themes emerging from Risk.net’s Top 10 op risks 2022 survey and how financial firms can better manage and mitigate the impact of…
Emerging trends in op risk
Karen Man, partner and member of the global financial institutions leadership team at Baker McKenzie, discusses emerging op risks in the wake of the Covid‑19 pandemic, a rise in cyber attacks, concerns around conduct and culture, and the complexities of…
Moving targets: the new rules of conduct risk
How are capital markets firms adapting their approaches to monitoring and managing conduct risk following the Covid‑19 pandemic? In a Risk.net webinar in association with NICE Actimize, the panel discusses changing regulatory requirements, the essentials…