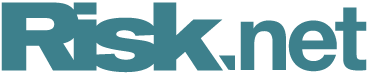
This article was paid for by a contributing third party.More Information.

Machine learning governance

The ability of machine learning models to read great quantities of unstructured data, spot patterns and translate it into actionable information is driving a significant uptake in the technology. David Asermely, SAS MRM global lead, highlights the need for rigorous model governance as businesses expect to adopt artificial intelligence and machine learning models to support key risk business use cases
Today, there is great interest in harnessing machine learning to turn the massive volumes of data – including non-traditional data – into new insights and information. In contrast to traditional statistical models, which are limited in the number of dimensions they can effectively access, machine learning models overcome these limitations and can ingest vast amounts of unstructured data, identify patterns and translate them into actionable information.
It is therefore no surprise that machine learning modelling is being eagerly adopted. A recent survey conducted by SAS and the Global Association of Risk Professionals found that, over the next three to five years, businesses expect to significantly increase adoption of artificial intelligence (AI) and machine learning models to support key risk business use cases (see figure 1). Banks, for example, are using machine learning models in marketing, fraud detection and anti-money laundering.

However, the fact that machine learning models need more governance than other data models is often overlooked. While machine learning models offer the promise of better predictions, they may also introduce ethical biases and increased model risk.
Machine learning models are designed to improve automatically through experience. This ability to ‘learn’ is what enables greater machine learning model accuracy and predictability. At the same time, it can heighten the need to quickly identify when a model begins to fail.
As a result, there’s an increased need to define operating controls on machine learning inputs (the data) and outputs (the model results). The dynamic nature of machine learning models means they require more frequent performance monitoring, constant data review and benchmarking, better contextual model inventory understanding, and well thought out and actionable contingency plans.
Looking ahead, the need for effective governance for machine learning models will only increase. This is a result of:
- Growing complexities of the global, multidimensional marketplace
- An increasing volume and complexity of data
- Rapidly increasing model usage by industries
- Growing complexity of machine learning models.
Implications of ineffective model risk management (MRM) on banks
Governance, risk and transparency concerns have introduced a major speed bump in machine learning model adoption. As noted by the US Federal Reserve: “Model risk increases with greater model complexity, higher uncertainty about inputs and assumptions, broader use, and larger potential impact.”
The promise of analysing non-traditional data using less transparent machine learning models – for example, to make better predictions – has raised major financial, reputational and regulatory concerns. Banks must be able to clearly explain their own models and how outputs were achieved using machine learning techniques – and yet often struggle to do so with machine learning models. Without this, how can regulators measure the systemic risks of such models to the global banking community?
The ‘explainability’ limitations of machine learning have also stopped many banks taking advantage of new, non-traditional data sources such as social media. Many machine learning models therefore don’t use new data sources, often resulting in them not meeting the expected lift in accuracy compared to historically tuned statistical models. Organisations must determine if the lift is worth the shift from well-understood and explainable models to more complex and less explainable machine learning models.
The solution: Robust, automated governance
Given concerns regarding transparency and the potential misuse of machine learning models, it is vital that organisations implement a robust and automated model governance system. The good news is that MRM teams are investing significant time and resources to determine how to best manage these models.
As AI/machine learning models become the norm, rigorous supporting model governance will be needed to classify machine learning models and introduce more frequent performance-monitoring quantitative data, benchmark comparisons, model usage, model interconnectedness, interpretability, variable sensitivity, modelling techniques, data metrics, model technique rational documentation and much more.
Learn more
Complex artificial intelligence/machine learning black-box models are being considered to replace well-understood statistical models. This change offers the promise of better predictions but may introduce unknown ethical biases and increased model risk. Model risk professionals are grappling with how to best reduce this risk with automation, technology and best practices. By utilising best practices – rationale, mapping, data governance, performance monitoring, recalibration, interpretability, benchmarking and contingency planning – financial organisations can better satisfy increased regulatory demands when implementing a robust, reliable and automated machine learning model governance infrastructure.
Model risk management – Special report 2019
Read more
Sponsored content
Copyright Infopro Digital Limited. All rights reserved.
As outlined in our terms and conditions, https://www.infopro-digital.com/terms-and-conditions/subscriptions/ (point 2.4), printing is limited to a single copy.
If you would like to purchase additional rights please email info@risk.net
Copyright Infopro Digital Limited. All rights reserved.
You may share this content using our article tools. As outlined in our terms and conditions, https://www.infopro-digital.com/terms-and-conditions/subscriptions/ (clause 2.4), an Authorised User may only make one copy of the materials for their own personal use. You must also comply with the restrictions in clause 2.5.
If you would like to purchase additional rights please email info@risk.net