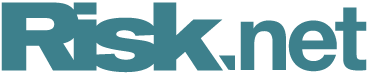
Research uncovers new sources of financial model risk
Past performance of financial models is no guarantee of future success, two forthcoming papers suggest

Investment managers will typically select financial models by looking at their past performance and using backtesting, but two academic studies forthcoming in Risk Journals suggest that approach may be mistaken.
Specifically, the papers draw attention to two key problems with this approach – overfitting and the “buying-out” effect – which underline existing industry concerns about model risk.
One study, by the University of Bremen’s Christian Fieberg and Thorsten Poddig, along with Eduard Baitinger, head of asset allocation at German investment manager Feri, investigated a range of 90 financial forecasting models which used various indicators to predict movements in a few major market indexes.
The authors calibrated each model using a 150-month sample period of historical data, before measuring its performance during the out-of-sample periods, both before and after the training period. The aim was to identify ‘performance persistence’ – in other words, whether models that performed well in the past would continue to do so.
Previous studies of economic forecasting models have found good evidence of persistence at the top and bottom ends of the scale. But this isn’t inevitable, and there are a number of cases in which the best-performing in-sample model would be among the worst-performing out-of-sample models and vice versa.
In their study, due to be published in the Journal of Risk in August, Fieberg and his colleagues found the same result with financial forecasting models, with one interesting exception: the very best performers in-sample showed no significant persistence out-of-sample.
Baitinger says this is due to the buying-out effect – the phenomenon whereby the best-performing models are the most lucrative, and so become applied more widely, eroding their competitive advantage and quickly reducing their performance.
“I strongly believe that the relative performance persistence behaviour of forecasting models depends on whether you can earn serious money with those models,” he says. “Given very intensive model discovery processes, this conclusion is what you would expect.”
In another study, Jonathan Borwein of the University of Newcastle in Australia, Qiji Jim Zhu of Western Michigan University, and David Bailey and Marcos Lopez de Prado of the US Lawrence Berkeley National Laboratory, explored a new way of testing for one possible explanation of the lack of performance persistence: overfitting. Overfitted models are those that are excessively complicated and trained to respond to past noise rather than future signals.
In a paper due to be published in the Journal of Computational Finance in March next year, the authors point out that growth in high-power computing has made it temptingly easy for financial researchers to produce false positives. This would leave firms with overfitted models that fail badly when tested out-of-sample. Even relatively simple models could involve billions of possible combinations of parameters and target securities, many of which will produce excellent risk/return profiles purely by chance.
Rather than designating a single set of data points – for instance, the previous three years – as the out-of-sample testing set, they use an approach they call “combinatorially symmetric cross-validation”. In this approach, the entire data set is divided into paired subsets, and each is used in turn as the training data set and the out-of-sample test data set. They argue this improves the testing process compared with the typical approach of simply splitting the data set into a training sample and a ‘hold-out’ sample for testing use.
The common approach of using either the most recent or oldest data as the hold-out sample means the designers either fail to train the model on the most recent and most relevant data, or test it against the least representative set of conditions. The use of pseudorandom data may introduce even more model risk, as the process used to generate the new data from the historical record may itself be overfitted.
The authors use the full series of comparisons between training and testing data to produce a probability of backtest overfitting (PBO) – where overfitting is defined as the situation in which the strategy that performs best in-sample has a below-median performance out-of-sample. If the strategy selection process yields a high PBO, then it is highly susceptible to overfitting.
“In that situation, the strategy selection process becomes in fact detrimental,” the authors write. But they caution the result will only apply at a group level; even if the measure indicates that a group of strategies has a high probability of overfitting, it may contain some individual strategies that legitimately perform well.
Additionally, they caution that this approach can only answer the question of whether a specific strategy selection process is likely to work. It can’t be used to assess the strategies themselves, as this would effectively bring the entire data set in-sample and produce a new risk of overfitting.
Feri’s Baitinger says that both overfitting and the buying-out effect could explain the tendency of high-performing models to break down out-of-sample. But he believes that further research is needed on this question – something that could involve developing deliberately flawed models and then studying them.
“A final answer with regard to the behaviour of overfitted models in the context of relative performance persistence cannot be given because no research exists on this issue,” he says. “For this purpose, one would have to create overfitted models intentionally and study their relative performance attributes. To the best of my knowledge, nobody has ever done this.”
Only users who have a paid subscription or are part of a corporate subscription are able to print or copy content.
To access these options, along with all other subscription benefits, please contact info@risk.net or view our subscription options here: http://subscriptions.risk.net/subscribe
You are currently unable to print this content. Please contact info@risk.net to find out more.
You are currently unable to copy this content. Please contact info@risk.net to find out more.
Copyright Infopro Digital Limited. All rights reserved.
As outlined in our terms and conditions, https://www.infopro-digital.com/terms-and-conditions/subscriptions/ (point 2.4), printing is limited to a single copy.
If you would like to purchase additional rights please email info@risk.net
Copyright Infopro Digital Limited. All rights reserved.
You may share this content using our article tools. As outlined in our terms and conditions, https://www.infopro-digital.com/terms-and-conditions/subscriptions/ (clause 2.4), an Authorised User may only make one copy of the materials for their own personal use. You must also comply with the restrictions in clause 2.5.
If you would like to purchase additional rights please email info@risk.net
More on Risk management
Emir 3.0 could complicate Eurex launch of repo on Prisma
Clearing house now targets November 2025, but faces hurdle from new model change approval process
Vendor oversight splinters across FMIs
Op Risk Benchmarking: firms grapple with “chaos” of third-party rule changes, amid growing recognition of cyber and resilience threats
Evalueserve tames GenAI to boost client’s cyber underwriting
Firm’s insurance client adopts machine learning to interrogate risk posed by hackers
Wait in the Q: US banks hold back on tariff-related provisions
Lack of data on supply chain vulnerabilities creates challenges for early CECL adjustments
Rising systemic risk demands a new risk management paradigm
Reinsurers need insurance-linked securities to share burden of climate-related catastrophic risk
ECB removes need for governing council to approve CCP facility
New “automatic” facility will require safeguards that are “still being implemented”, bank says
Dodging a steamroller: how the basis trade survived the tariff tantrum
Higher margins, rising yields and stable repo funding helped avert another disruptive blow-up
BoE plans to link system-wide and individual stress tests
Meanwhile, ECB wants to broaden system-wide stress models to include central counterparties