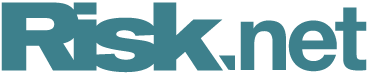
This article was paid for by a contributing third party.More Information.

Harnessing AI to achieve Libor transition

Chris Dias, principal at KPMG, explains how the vast increase in accuracy that artificial intelligence (AI) offers when dealing with large volumes of complex agreements is crucial to exploring the market opportunities and mitigating the risks of the transition away from Libor. Implementing a robust AI capability is an important starting point

Understanding exposure to Libor and the risk associated with it is a critical first step. Firms will need this information to better understand potential outcomes, allowing them to more comprehensively determine action steps for their businesses, operations and clients. Many firms will use existing systems containing structured data to capture a notional value or risk value of exposure. Such an assessment can provide an initial estimate of the challenge ahead, but not a full understanding of the range of possible outcomes.
Refining exposure and risk must go beyond straightforward system value to include consideration of contractual and legal risk, and economic exposure. These elements are best evaluated when combining structured data (from systems) with unstructured data (from contracts and other documents). Information such as consent, termination rights and cessation language are some of the details needed to truly develop an accretive action plan. The immediate challenge for most firms will be to gather, organise, analyse and manage this additional unstructured data.
Organising, analysing and managing data from structured sources, while complex, is a well-understood task that can be accomplished via data engineering tools and approaches. In contrast, organising information from unstructured sources poses new challenges.
The new challenges are manifold and can almost seem insurmountable. Unstructured data can exist anywhere – in risk and accounting systems, spreadsheets and filing cabinets. The quality of the data can vary considerably – from digitally pristine to indecipherably handwritten. Data for financial contracts may not be located in a single system or even one location and, in some cases, deals may have been largely disaggregated into component pieces, making recombination quite aggravating. And any changes to contracts in the form of amendments may not be linked in ways that create a transparent association.
Managing disparate and unstructured data
When working with unstructured data, careful planning is required to determine what information is needed from contracts and documents. In contrast to structured data where variables and fields are already established, documents contain many potential pieces of information but no fixed structure or patterns of language. Best practice is for each institution to focus on desired final business outcomes by thinking carefully about how it intends to treat groups of related contracts under the Libor transition. This pertains to both common contract types as well as bespoke contract types. After establishing preliminary transition plans and organising documents into working repositories, the analysis begins.
The starting point is to link system data (structured data) to the data found in the contracts themselves (unstructured data). Unfortunately there are two major issues associated with this effort:
1. Contracts can be in a digitised, digital scan or physical paper document format and, if on paper, could be located anywhere
2. The effort required to review all Libor-related data is so great that it may not be possible to accomplish by the time Libor ceases to exist in December 2021.
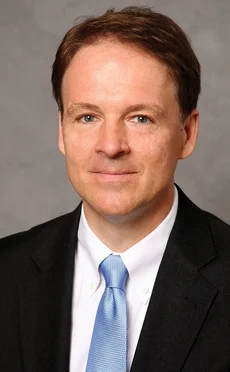
Fortunately, operations research and statistical analysis have recently experienced a resurgence in the form of data analytics. This data science renaissance provides firms with the capability to more easily digitise documents through optical character recognition, making them machine readable. In this form, AI capabilities such as natural language processing and machine learning can be brought to bear, and any document analysis can be undertaken in seconds by a well-trained computer.
AI produces value in several ways. At its most basic, it can perform information retrieval – extracting specific facts and items from documents. This can include names, dates, defined terms and blocks of text describing fallback mechanisms. Of greater value, a well-trained AI capability can apply reason to the information in the document to interpret and summarise. This interpretation can yield information about consent requirements, consistency of language with Alternative Reference Rates Committee guidance, and interdependency of defined terms and related documents; it can group together agreements with similar expected Libor transition handling strategies regardless of variance in language. Finally, AI can perform natural language generation to create draft amendments, summary reports, notices, communications and, potentially, chatbots for internal resources or external clients to interact with.
Conclusion
Given the wide variations in language and subtle details of most agreement types, this analysis can be very expensive when performed manually. AI is a highly scalable and cost-effective tool for facilitating transition. Vertical scalability is the ability to apply trained AI-based reasoning to large numbers of agreements. Horizontal scaling is the ability to extend an AI capability’s training to accommodate new agreement types. A trained AI capability can process and interpret agreements in a matter of seconds and can be deployed to efficiently address large populations at a fraction of human cost. Finally, the accuracy of AI consistently and significantly exceeds human accuracy when dealing with large volumes of complex agreements. Whether you want to explore market opportunities or mitigate risk resulting from the transition, having a robust AI capability is an important first step.
About the authors
Chris Dias is a partner at KPMG in the US Capital Markets group, serving financial services companies as a risk practitioner and strategic adviser. He is an accomplished professional with 25 years of international experience in financial markets.
Timothy Cerino is a managing director in KPMG’s Lighthouse Data and Analytics Center of Excellence, where he leverages new big data architecture and statistical machine learning methods to provide advice and insights that complement traditional approaches and support advisory engagements. Areas of opportunity include commercial banking, risk management, regulatory compliance, portfolio management, capital markets, corporate finance and development of new, data-driven business solutions.
The KPMG name and logo are registered trademarks or trademarks of KPMG International. The information contained herein is of a general nature and is not intended to address the circumstances of any particular individual or entity. Although we endeavour to provide accurate and timely information, there can be no guarantee that such information is accurate as of the date it is received or that it will continue to be accurate in the future. No one should act upon such information without appropriate professional advice after a thorough examination of the particular situation.
Libor transition and implementation – Special report 2019
Read more
Sponsored content
Copyright Infopro Digital Limited. All rights reserved.
As outlined in our terms and conditions, https://www.infopro-digital.com/terms-and-conditions/subscriptions/ (point 2.4), printing is limited to a single copy.
If you would like to purchase additional rights please email info@risk.net
Copyright Infopro Digital Limited. All rights reserved.
You may share this content using our article tools. As outlined in our terms and conditions, https://www.infopro-digital.com/terms-and-conditions/subscriptions/ (clause 2.4), an Authorised User may only make one copy of the materials for their own personal use. You must also comply with the restrictions in clause 2.5.
If you would like to purchase additional rights please email info@risk.net