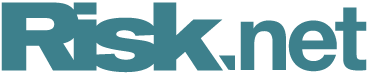
Artificial intelligence and the future of financial regulation
Software has taken over from humans in trading – and will spread further in the years to come. Regulators will need to prepare for a faster, darker industry

At some point in the last few years, the human stock trader became an endangered species. Most trades on the world's equity markets are now conducted by machines – algorithmic trading systems. And research in the areas of machine learning, big data and artificial intelligence promises to change the financial world still more fundamentally in the near future – bringing both new benefits and new challenges for operational risk teams and compliance managers.
Regulatory attention so far in this area
Only users who have a paid subscription or are part of a corporate subscription are able to print or copy content.
To access these options, along with all other subscription benefits, please contact info@risk.net or view our subscription options here: http://subscriptions.risk.net/subscribe
You are currently unable to print this content. Please contact info@risk.net to find out more.
You are currently unable to copy this content. Please contact info@risk.net to find out more.
Copyright Infopro Digital Limited. All rights reserved.
As outlined in our terms and conditions, https://www.infopro-digital.com/terms-and-conditions/subscriptions/ (point 2.4), printing is limited to a single copy.
If you would like to purchase additional rights please email info@risk.net
Copyright Infopro Digital Limited. All rights reserved.
You may share this content using our article tools. As outlined in our terms and conditions, https://www.infopro-digital.com/terms-and-conditions/subscriptions/ (clause 2.4), an Authorised User may only make one copy of the materials for their own personal use. You must also comply with the restrictions in clause 2.5.
If you would like to purchase additional rights please email info@risk.net
More on Regulation
Iosco pre-hedging review: more RFQs than answers
Latest proposals leave observers weighing new clampdown on pre-hedging
FCMs welcome CFTC margin rule ring-fencing clarification
Final rule on separate accounts replicates no-action relief as Republicans strip out gold plate
Stuck in the middle with EU: dealers clash over FRTB timing
Largest banks want Commission to delay implementation, but it’s not the legislator’s only option
Treasury clearing timeline ‘too aggressive’ says BofA rates head
Sifma gears up for extension talks with incoming SEC and Treasury officials
Rostin Behnam’s unfinished business
Next CFTC chair must finish the work Behnam started on crypto regulation and conflicts of interest
European Commission in ‘listening mode’ on potential FRTB changes
Delay or relief measures on the table after UK postpones start of Basel III to 2027
Australian FRTB projects slow down amid scheduling uncertainty
Market risk experts think Apra might soften NMRF regime to spur internal model adoption
EBA to address double-counting caused by new capital floor
Existing EU capital add-ons for model risk would duplicate new Basel floor on internal models