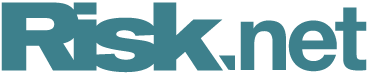
Quant Guide 2020: Carnegie Mellon University
Pittsburgh and New York City, US
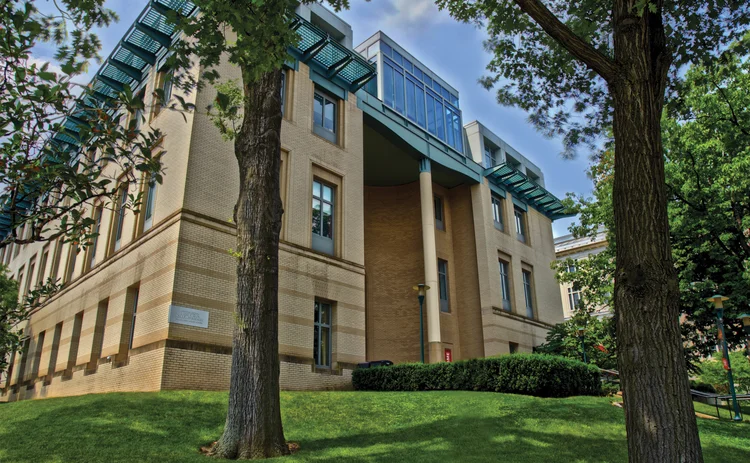
Successful applicants to Carnegie Mellon University’s Master of Science in Computational Finance are presented with a unique choice: the programme is taught on two campuses, and candidates pick one of them. The campus in Pittsburgh, Pennsylvania is the larger of the two and the university’s main academic hub. The New York campus is a lot smaller, but has understandable appeal for fans of quantitative finance. Classes take place in both locations; if a particular lecture is happening at the Pittsburgh campus for a given week, it is livestreamed to New York and vice versa.
Adjunct professor of business Richard Bryant is the executive director of the programme, which sits at eighth place once again in this year’s Risk.net quant guide. Part of the reason for its high ranking is the healthy average salary of degree-holders. The figure has risen by several thousand dollars annually since 2016, to a high last year of $105,056, six months after graduation. The faculty is also broad: teaching staff are drawn from the department of statistics and data science, the Heinz college of information systems and public policy, the department of mathematical sciences and the Tepper School of Business.
The programme’s three terms – autumn, spring and autumn – are divided into six mini-semesters of seven weeks each. The mini-semesters, each designed with a specific quantitative finance career path in mind, function in a similar way to what some institutions call concentrations or tracks. The six topics are trading, financial modelling, quantitative portfolio management, risk management and data science.
“As petabytes of financial data have become readily available, data science and statistics are playing a more important role in quant finance,” says Bryant. The degree has an embedded data science programme and a new addition to the curriculum is a corporate-sponsored project course in machine learning.
Only users who have a paid subscription or are part of a corporate subscription are able to print or copy content.
To access these options, along with all other subscription benefits, please contact info@risk.net or view our subscription options here: http://subscriptions.risk.net/subscribe
You are currently unable to print this content. Please contact info@risk.net to find out more.
You are currently unable to copy this content. Please contact info@risk.net to find out more.
Copyright Infopro Digital Limited. All rights reserved.
As outlined in our terms and conditions, https://www.infopro-digital.com/terms-and-conditions/subscriptions/ (point 2.4), printing is limited to a single copy.
If you would like to purchase additional rights please email info@risk.net
Copyright Infopro Digital Limited. All rights reserved.
You may share this content using our article tools. As outlined in our terms and conditions, https://www.infopro-digital.com/terms-and-conditions/subscriptions/ (clause 2.4), an Authorised User may only make one copy of the materials for their own personal use. You must also comply with the restrictions in clause 2.5.
If you would like to purchase additional rights please email info@risk.net
More on Quantitative finance
Quant Finance Master’s Guide 2025
Risk.net’s guide to the world’s leading quant master’s programmes, with the top 25 schools ranked
Baruch maintains top spot in 2025 Quant Master’s Guide
Sorbonne reclaims top spot among European schools, even as US salaries decouple
Quant Finance Master’s Guide 2023
Risk.net’s guide to the world’s leading quant master’s programmes, with the top 25 schools ranked
Baruch topples Princeton in Risk.net’s quant master’s rankings
US schools cement top five dominance as graduate salaries soar
Is it worth doing a quant master’s degree?
UBS’s Gordon Lee – veteran quant and grad student supervisor – asks the hard question
Starting salaries jump for top quant grads
Quant Guide 2022: Goldman’s move to pay postgrads more is pushing up incomes, says programme director
Quant Finance Master’s Guide 2022
Risk.net’s guide to the world’s leading quant master’s programmes, with the top 25 schools ranked
Princeton, Baruch and Berkeley top for quant master’s degrees
Eight of 10 leading schools for quantitative finance programmes are based in US, latest rankings show