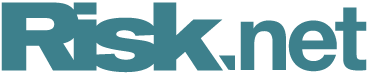
Fake data can help backtesters, up to a point
Synthetic data made with machine learning will struggle to capture the caprice of financial markets
Quant investors often complain they have only a single version of history against which to test their ideas.
One way to get round the problem has been to make history up. Quants have done that for a long time already – using bootstrapping or Monte Carlo simulations to create alternative time series data for the backtests they run.
A new idea, though, is to employ machine learning techniques to invent wholly artificial data. Quants are experimenting with these models and say they can produce data indistinguishable in some cases from the real thing.
The potential of the new ‘fake’ data gives cause for optimism. With it, quants can test strategies against scenarios that might have happened as well as those that did. There’s a caveat, though. Fake data may fix some of the shortcomings of conventional backtesting, but it can’t fix all of them.
The models the quants are using to generate the new data effectively learn the process by which past data was generated.
That’s worked impressively outside investing, where the models have been used to create anything from deep fake videos to so-called Ganimals – synthesised animals, like an elephant crossed with a cat – conjured up using generative adversarial networks (Gans).
Amazon used synthetic data to train its Alexa bot to understand instructions in Hindi. Rather than train the voice recognition software with millions of real commands, the tech firm generated fake samples from data on just a subset of recordings.
But applications in financial markets face a key difference from applications in other such fields. Markets are fast-changing systems, subject at times to sudden, unexpected regime shifts.
Backtesting with multiple versions of history may be better than backtesting with one. But the generative models still are recreating a version of events learnt from the past. And even a richer view of history could be a poor guide to the future.
One quant draws a parallel with forecasting climate change, a process in which what’s gone before – by definition – will be largely redundant. And in the case of equity markets, “even the most intimate knowledge of history isn’t going to tell you where Apple’s stock price is going to be,” he says.
In another way, too, historical data could prove a bad teacher.
The mechanics of the market are hugely complex, including the actions and motivations of thousands of investors, companies and intermediaries and the complex dynamics of market microstructure. That’s before accounting for the influence of the global macro environment, news events, and so on.
It’s never guaranteed the data will lead a model to a full understanding of those mechanics. That’s to say, the training set may provide only a patchy representation of the truth. “You could end up generating fake data that’s just too simplistic for what’s at stake,” says another quant. “It could be counterproductive.”
These are limitations the data-generation models have not faced outside finance. They also are limitations that apply to any form of backtesting, be it conventional or using fake data. But as investors proceed with the new techniques, they will need to keep sight of the problems that fake data cannot solve. A picture of an elephant or a cat looks like a picture of an elephant or a cat for ever. A picture of a market is always changing.
Only users who have a paid subscription or are part of a corporate subscription are able to print or copy content.
To access these options, along with all other subscription benefits, please contact info@risk.net or view our subscription options here: http://subscriptions.risk.net/subscribe
You are currently unable to print this content. Please contact info@risk.net to find out more.
You are currently unable to copy this content. Please contact info@risk.net to find out more.
Copyright Infopro Digital Limited. All rights reserved.
As outlined in our terms and conditions, https://www.infopro-digital.com/terms-and-conditions/subscriptions/ (point 2.4), printing is limited to a single copy.
If you would like to purchase additional rights please email info@risk.net
Copyright Infopro Digital Limited. All rights reserved.
You may share this content using our article tools. As outlined in our terms and conditions, https://www.infopro-digital.com/terms-and-conditions/subscriptions/ (clause 2.4), an Authorised User may only make one copy of the materials for their own personal use. You must also comply with the restrictions in clause 2.5.
If you would like to purchase additional rights please email info@risk.net
More on Our take
Getting a handle on model parameters
Mean reversion in rate parameters opens the door to dimensionality reduction
The case for believing in a Bessent put
Money market funds could prove critical in efforts to control 10-year yields
FRTB may bite harder for Europe’s CVA modellers
Farther reach of advanced approach and lighter load on total requirements mean limited takeaways from Canada and Japan’s implementation
Japan, Basel III and the pitfalls of being on time
Capital floor phase-in delay may be least-worst option for JFSA as US and Europe waver
FX traders revel in March Madness
Chaotic Trump policies finally bring diversity to flows – to the delight of market-makers
Market knee-jerks keep VAR models on their toes
With a return to volatility, increased backtesting exceptions show banks’ algos are stretched
A market-making model for an options portfolio
Vladimir Lucic and Alex Tse fill a glaring gap in European-style derivatives modelling
How AI agents could become investing’s crash test dummies
Firms mull the use of chatbot simulations to test organisational set-ups