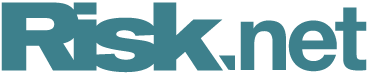
Danske quants discover speedier way to crunch XVAs
Differential machine learning produces results “thousands of times faster and with similar accuracy”
Quants at Danske Bank have found a way to greatly speed up calculations for complex derivatives portfolios, such as American options and autocallables, without the need for clunky Monte Carlo simulations.
The approach proposed by Copenhagen-based quantitative analysts Brian Huge and Antoine Savine combines two of the most powerful weapons in the modern quant finance arsenal. The first is adjoint algorithmic differentiation (AAD), a mathematical technique that is widely used to calculate sensitivities at high speed. The second is neural networks, a type of machine learning that can find patterns in large datasets.
AAD was introduced to finance by Michael Giles and Paul Glasserman in 2006. Danske Bank has successfully applied the technique to significantly reduce the time it takes to calculate credit valuation adjustments, or CVA. AAD generates so-called pathwise differentials for various risk factors. These are currently used to feed the bank’s nested Monte Carlo simulations for derivatives portfolios.
Huge and Savine realised they could do more with those outputs. “The pathwise differentials in themselves contain plenty of information and we suspected we could use it to train pricing approximations more effectively,” Huge says.
They experimented with different pricing approximations, including simple regressions, before shifting their focus to neural networks. A scarcity of data makes it difficult for neural networks to learn the structure of complex derivatives. Augmenting the training data with pathwise differentials solves this problem, and helps prevent overfitting. “We have a tremendous amount of pathwise derivatives. And all these pathwise derivatives are computed accurately and virtually for free with AAD,” says Savine
The initial results exceeded their expectations. The approach, which they call differential machine learning, produced stable prices at a very low computational costs.
The main benefit of differential machine learning is that it helps in “avoiding nested simulations, which are very costly”, says Huge.
“Everything we can do with the differential machine learning can also be done already with nested Monte Carlo simulation,” Savine explains. “With differential machine learning you just do it thousands of times faster and with similar accuracy.”
Using AAD pathwise derivatives to regularise the exposure estimation process is a very clever idea to reduce noise
Giuseppe Benedetti, FIS
For Savine, speed is not the only benefit. “Every time you increase speed, the quality of your risk management massively improves, because when calculations are slow, people have to cut corners,” he says.
The obvious use case is the calculation of derivatives valuation adjustments (XVAs), and margin valuation adjustments in particular. But the potential applications are much wider. “It can also be used to generate dynamic risk reports, because we can quickly compute risks in a vast number of future scenarios,” says Huge.
Bruno Dupire, head of quantitative research at Bloomberg, says the approach “can be applied to any type of derivative portfolio to compute prices, sensitivities or, mostly importantly, highly time-consuming tasks like XVA”.
Dupire is best known for his work on local volatility models. But he was also one of the first to apply neural networks to time series analysis in the 1980s. He is glad to see machine learning finally making tangible contributions to the pricing of derivatives.
“The trick of generating scenarios between today and a contract’s maturity without doing the nesting and using neural network to regress, while training also on the differentials, means the machine learns the shape or the function in a more accurate way,” says Dupire.
Giuseppe Benedetti, senior quantitative analyst at financial software vendor FIS, says differential machine learning will be useful to anyone running calculations that require estimates of future exposures. “We’re looking into this technique as a candidate to be implemented in our products,” he says. “Using AAD pathwise derivatives to regularise the exposure estimation process is a very clever idea to reduce noise and make the methodology more reliable and applicable in production environments.”
Danske Bank is deploying differential machine learning in its front-office platform. The model is currently undergoing testing by the front office and market risk department. The next step is implementing it in production. Huge has no doubt this will happen. “We expect it to compute the official numbers for the bank in 2021,” he says.
Only users who have a paid subscription or are part of a corporate subscription are able to print or copy content.
To access these options, along with all other subscription benefits, please contact info@risk.net or view our subscription options here: http://subscriptions.risk.net/subscribe
You are currently unable to print this content. Please contact info@risk.net to find out more.
You are currently unable to copy this content. Please contact info@risk.net to find out more.
Copyright Infopro Digital Limited. All rights reserved.
As outlined in our terms and conditions, https://www.infopro-digital.com/terms-and-conditions/subscriptions/ (point 2.4), printing is limited to a single copy.
If you would like to purchase additional rights please email info@risk.net
Copyright Infopro Digital Limited. All rights reserved.
You may share this content using our article tools. As outlined in our terms and conditions, https://www.infopro-digital.com/terms-and-conditions/subscriptions/ (clause 2.4), an Authorised User may only make one copy of the materials for their own personal use. You must also comply with the restrictions in clause 2.5.
If you would like to purchase additional rights please email info@risk.net
More on Our take
Getting a handle on model parameters
Mean reversion in rate parameters opens the door to dimensionality reduction
The case for believing in a Bessent put
Money market funds could prove critical in efforts to control 10-year yields
FRTB may bite harder for Europe’s CVA modellers
Farther reach of advanced approach and lighter load on total requirements mean limited takeaways from Canada and Japan’s implementation
Japan, Basel III and the pitfalls of being on time
Capital floor phase-in delay may be least-worst option for JFSA as US and Europe waver
FX traders revel in March Madness
Chaotic Trump policies finally bring diversity to flows – to the delight of market-makers
Market knee-jerks keep VAR models on their toes
With a return to volatility, increased backtesting exceptions show banks’ algos are stretched
A market-making model for an options portfolio
Vladimir Lucic and Alex Tse fill a glaring gap in European-style derivatives modelling
How AI agents could become investing’s crash test dummies
Firms mull the use of chatbot simulations to test organisational set-ups