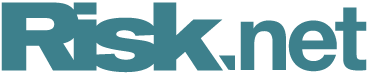
Backtesting expected shortfall: mission accomplished?
A rigorous backtest for ES cannot exist, but a good approximation might do the job
The debate on the backtestability of expected shortfall (ES) is almost as old as the risk measure itself. Despite warnings from prominent quants that the measure was unsuitable because no backtest existed, the Basel Committee chose to adopt it as the standard measure for the calculation of market risk capital requirements – prompting numerous research projects dedicated to validating its performance.
ES has long lived in the shadow of value-at-risk, a measure that in itself provides the risk manager with information of questionable usefulness. Its advantage is that it is very easy to backtest: to verify if VAR has been estimated correctly, it is sufficient to compare the series of realised returns and that of daily VAR estimates. If the number of returns violates the threshold set by the VAR’s confidence level, then the backtest has failed and VAR needs to be recalibrated.
With ES, however, it is not so simple. ES represents the average risk in the tail of the distribution. Intuitively, validating a measure of risk by comparing it to realised returns is like comparing apples to oranges: the first is a function of a probability distribution, while the second is a single realisation from that distribution. On more rigorous grounds, it was proved by Tilmann Gneiting in 2011 that ES is, strictly speaking, not backtestable. This is because it lacks a mathematical property called elicitability, which is necessary in order for any risk measure to submit to a rigorous backtest.
Also, because ES by definition relies on VAR predictions, its own accuracy and the reliability of its backtest are inevitably sensitive to the accuracy of VAR predictions. Incorrect VAR estimates may potentially lead to excessively prudential ES values and consequent suboptimal allocation of capital, or, worse, losses of unexpected frequency or magnitude.
Any validation test on ES therefore needs to have some degree of approximation. Because large biases in VAR entail higher capital charges for the banks, it is crucial to minimise such biases. An idea on how to achieve such minimisation comes from the latest work of Carlo Acerbi, head of valuation and quantitative solutions at Banque Pictet, and Balazs Szekely, an economic adviser at the Central Bank of Hungary.
“You can never write a test that is exclusively testing ES; you are always testing ES and VAR,” explained Acerbi, in a podcast with Risk.net to discuss his research. “A test for ES is perfect only if the VAR prediction is perfect,” he added.
Acerbi and Szekely’s test is a function of both ES and VAR predictions. The backtest is possible thanks to the introduction of the so-called realised ES, which is an unobservable quantity but is clearly defined by the authors in their paper as a function of VAR predictions.
Its attractiveness lies in the fact that it allows the user to compare quantities of the same kind: forecast and realised ES. Interestingly, such a test not only provides an answer to whether the ES model is accepted or rejected, but it also gives the difference between the expected and the realised ES, giving a precise measure of the error. This allows a bank to adjust its risk models accordingly.
The authors derive the explicit formula for that difference, or bias, and find that it is always positive. That means capital charges can be overestimated, but not underestimated – a welcome prudential feature. From that formula, it can also be observed that bias is conveniently small.
As part of the explanation of their method, the authors introduce the concept of sharpness of backtests. A test is ‘sharp’ if its expectation function only depends on forecast values and realised values of the statistic being backtested. Their backtest complies with such a criterion.
The desirable properties of this technique come with a cost, albeit a manageable one. Its implementation is not as straightforward as that of VAR backtests, where it is sufficient to collect two data points per interval and count if the violations are within the boundary of acceptability.
When backtesting ES, it is necessary to collect the daily forecast of the entire distribution of the tail. The distribution can be described by a parametric model, which means one must have associated the tail with a specific family of statistical distribution (for example Gaussian, or Student-t or chi-square) and its statistical moments. A more computationally intensive alternative is to describe the tail with its historical distribution and resample it to generate the forecast.
Acerbi and Szekely’s method is currently being examined by the risk team of the Bank of Italy, where the risk measure is currently being applied to the central bank’s own portfolio. Marco Orlandi is part of a team of three within the financial risk control function of the central bank, working on finding a reliable backtest for expected shortfall.
“Our feedback on their backtest is so far very positive,” says Orlandi. “We hope to be able to show the results internally by the end of the year,” adding that it will likely be part of an approximately annual procedure to assess their risk methodologies.
Acerbi and Szekely are optimistic about the possible applications of their solutions, which, beyond the backtesting of capital models, could include rule-based margins, risk budgeting or stop-risk rules.
Only users who have a paid subscription or are part of a corporate subscription are able to print or copy content.
To access these options, along with all other subscription benefits, please contact info@risk.net or view our subscription options here: http://subscriptions.risk.net/subscribe
You are currently unable to print this content. Please contact info@risk.net to find out more.
You are currently unable to copy this content. Please contact info@risk.net to find out more.
Copyright Infopro Digital Limited. All rights reserved.
As outlined in our terms and conditions, https://www.infopro-digital.com/terms-and-conditions/subscriptions/ (point 2.4), printing is limited to a single copy.
If you would like to purchase additional rights please email info@risk.net
Copyright Infopro Digital Limited. All rights reserved.
You may share this content using our article tools. As outlined in our terms and conditions, https://www.infopro-digital.com/terms-and-conditions/subscriptions/ (clause 2.4), an Authorised User may only make one copy of the materials for their own personal use. You must also comply with the restrictions in clause 2.5.
If you would like to purchase additional rights please email info@risk.net
More on Our take
Getting a handle on model parameters
Mean reversion in rate parameters opens the door to dimensionality reduction
The case for believing in a Bessent put
Money market funds could prove critical in efforts to control 10-year yields
FRTB may bite harder for Europe’s CVA modellers
Farther reach of advanced approach and lighter load on total requirements mean limited takeaways from Canada and Japan’s implementation
Japan, Basel III and the pitfalls of being on time
Capital floor phase-in delay may be least-worst option for JFSA as US and Europe waver
FX traders revel in March Madness
Chaotic Trump policies finally bring diversity to flows – to the delight of market-makers
Market knee-jerks keep VAR models on their toes
With a return to volatility, increased backtesting exceptions show banks’ algos are stretched
A market-making model for an options portfolio
Vladimir Lucic and Alex Tse fill a glaring gap in European-style derivatives modelling
How AI agents could become investing’s crash test dummies
Firms mull the use of chatbot simulations to test organisational set-ups