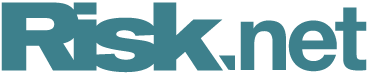
This article was paid for by a contributing third party.More Information.
Revolutionising credit surveillance: part two
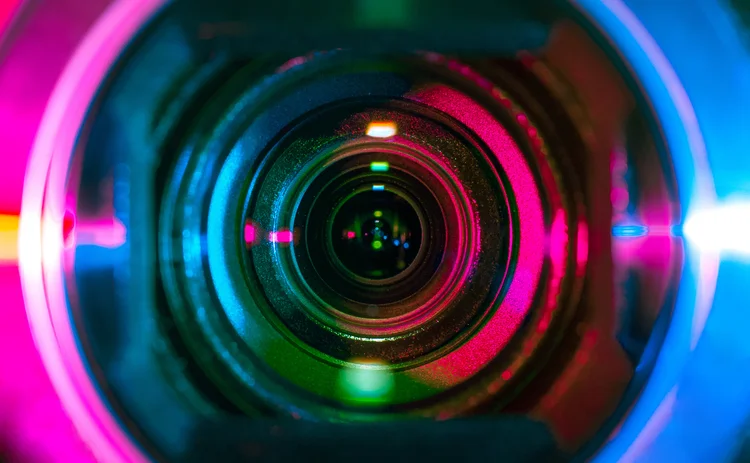
Does generative artificial intelligence (GenAI) live up to the hype? S&P Global Market Intelligence’s Michelle Cheong and Shruthi Nagarajan discuss how prioritising AI and digitisation projects reveals data as the power behind AI initiatives
The authors
- Michelle Cheong, Head, Credit Solutions Thought Leadership, S&P Global Market Intelligence
- Shruthi Nagarajan, Senior research analyst, Credit Solutions, S&P Global Market Intelligence
The authors acknowledge the input of Stephen Hender, head of technology for Credit & Risk Solutions; Katriona Ho, head of market development, Credit Analytics, Asia-Pacific (Apac); and Andy Chu, head of S&P Global Market Intelligence Solutions architect team, Apac.
Where are we in the AI hype cycle?

After several years of buzz around GenAI, S&P Global Market Intelligence has noticed its clients are taking a candid look at their digitisation initiatives in an effort to determine if indeed they are delivering value (see figure 1).
Reprioritising AI and digitisation projects can encompass the following factors:
- Evaluating costs versus benefits: assess the value of AI and digitisation initiatives against their delivery costs.
- Reassessing criticality and value: analyse the competitive advantages of new solutions compared to existing ones, ranking their importance to achieve strategic initiatives, and determining their scalability and future-proof capabilities.
- Quantifying implementation accuracy and impact of risks: investigate the accuracy of automated solutions, identify any critical information that may be overlooked and evaluate potential cyber and data security risks associated with these applications.
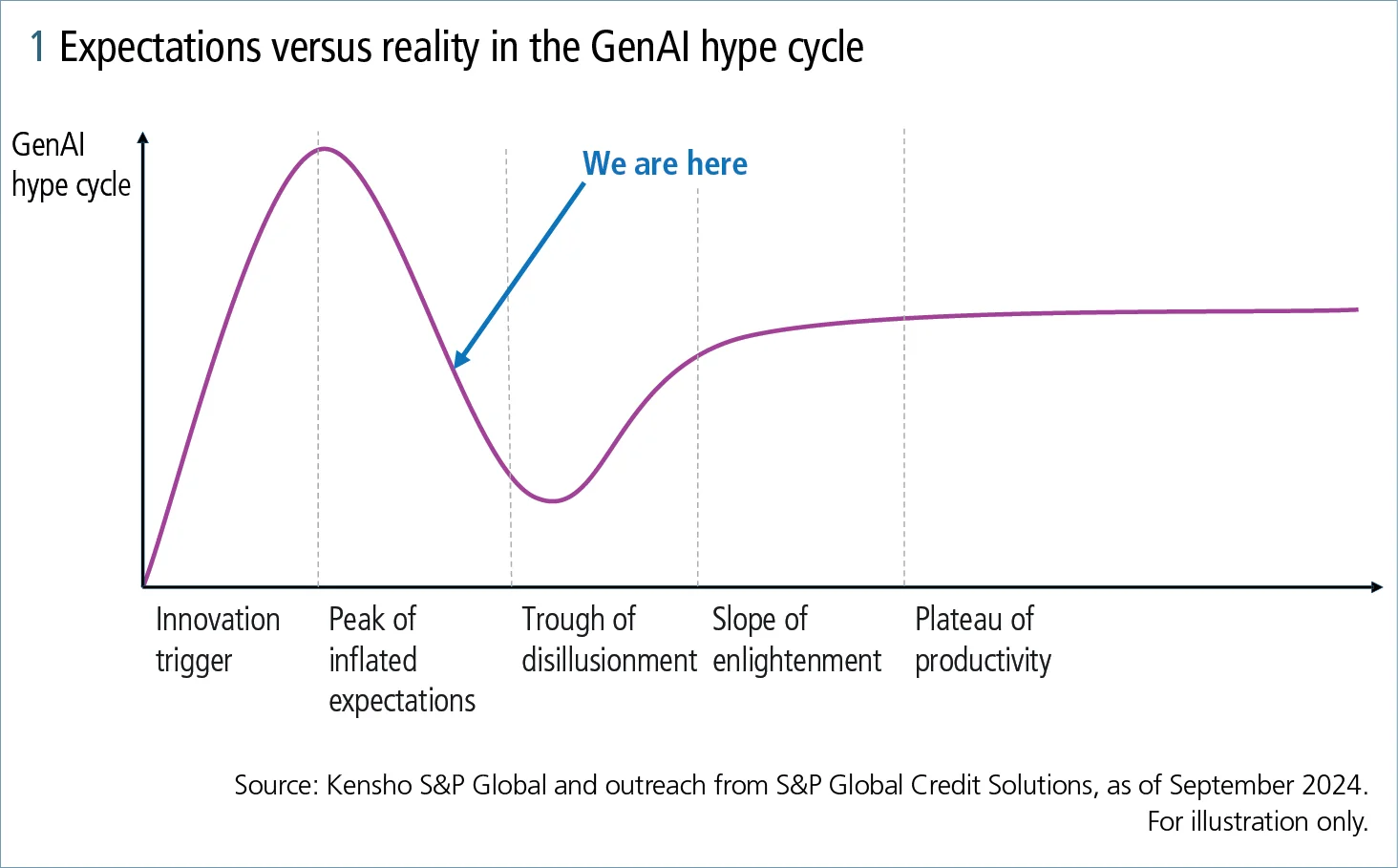
Suitable, fit-for-purpose data as the power behind the AI engine

Through our interactions with clients and the development of our own AI capabilities, we have recognised that data is a pivotal factor in the success of AI applications. Even though comprehensive data testing typically occurs after proof-of-concept (PoC) review, where the potential value of AI initiatives has been communicated to stakeholders, the significance of suitable, fit-for-purpose data cannot be overstated.
Such data, as illustrated in figure 2, can integrate seamlessly into internal workflows, provide substantial value at reduced costs and facilitate the visualisation of information for business units. These attributes help the proposed digitisation or AI initiatives realise their intended benefits. Nevertheless, it is common practice for data sourcing and verification to take place only after the business case has been established at the PoC stage and stakeholder approval has been obtained to move projects forward.
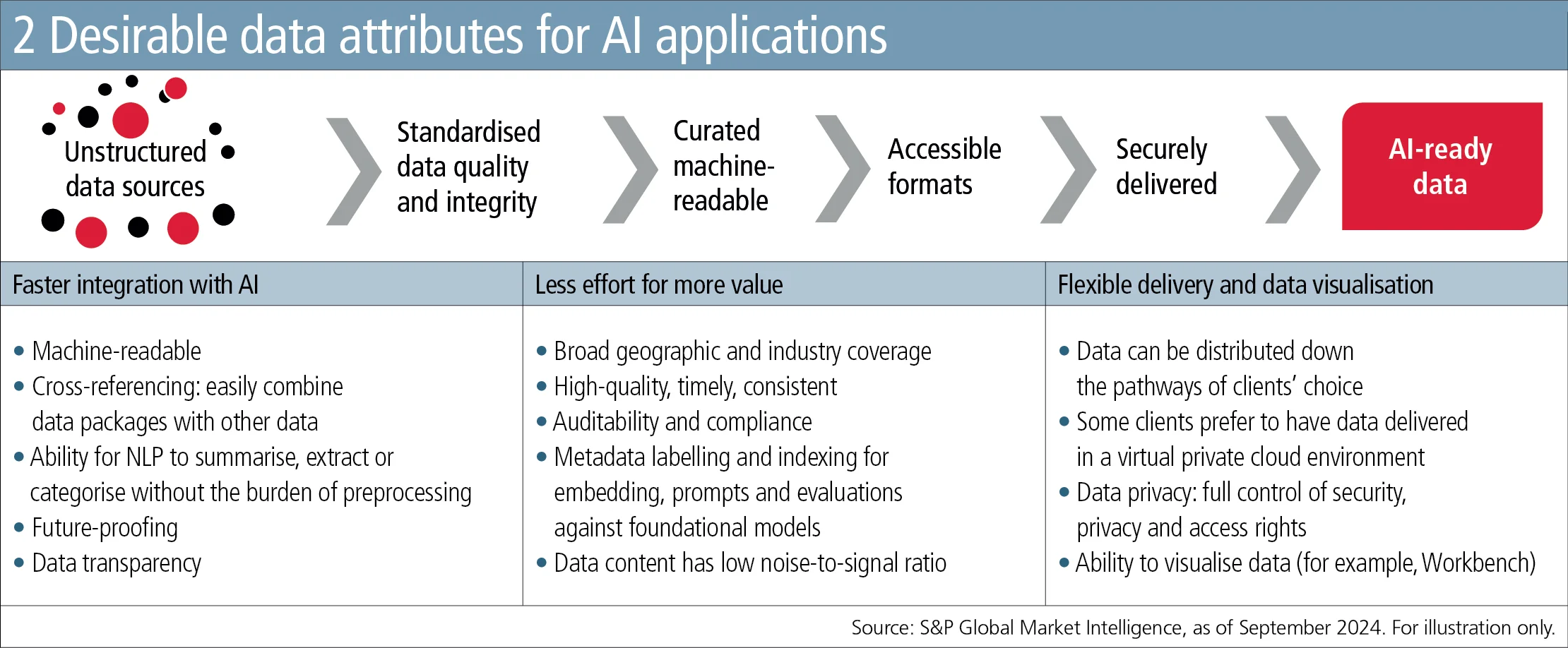
AI applications illustrated: NLP-driven financial thresholds and automated credit memo processing
To help address a potential mismatch between expectations from PoCs and the reality of project deliverables, S&P Global Market Intelligence has been investing in providing these data attributes up front. These desirable data attributes benefit S&P Global’s internal AI-powered applications as well, including S&P Global Market Intelligence’s natural language processing (NLP)-powered financial thresholds on RatingsDirect® (see figure 3), which is one indicator that may be helpful to assess how far an entity is from a potential credit rating change, and the beta version of its credit research chatbot on RatingsDirect® on S&P Capital IQ Pro.
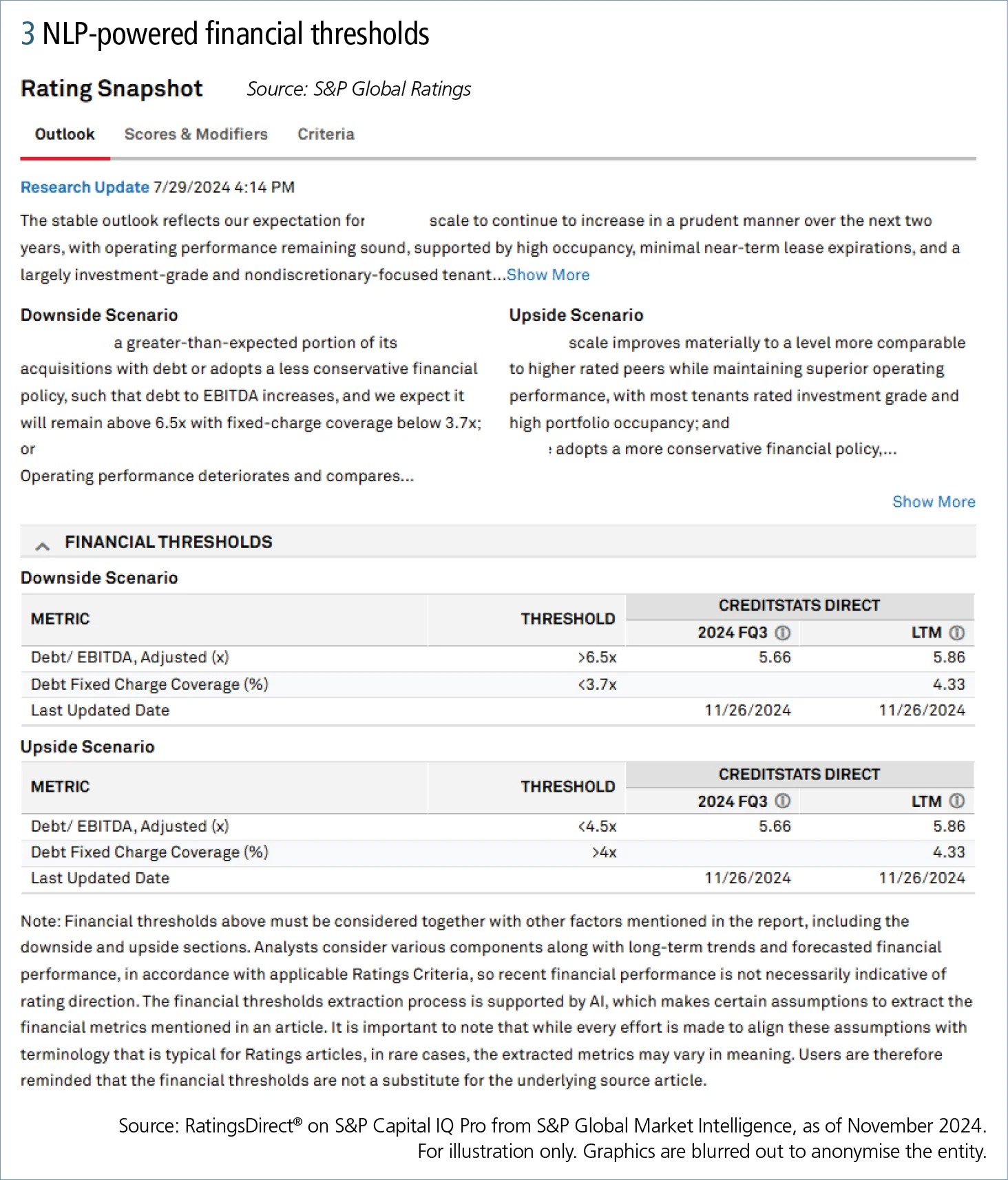
This data also can be fed into clients’ own internal AI or automation solutions. For instance, credit memos can be automated for large-scale generation, a common use case in commercial lending, capital markets and wealth management. Figures 4 and 5 illustrate live data accessible through the Xpressfeed™, XpressAPI and XpressCloud delivery platforms.
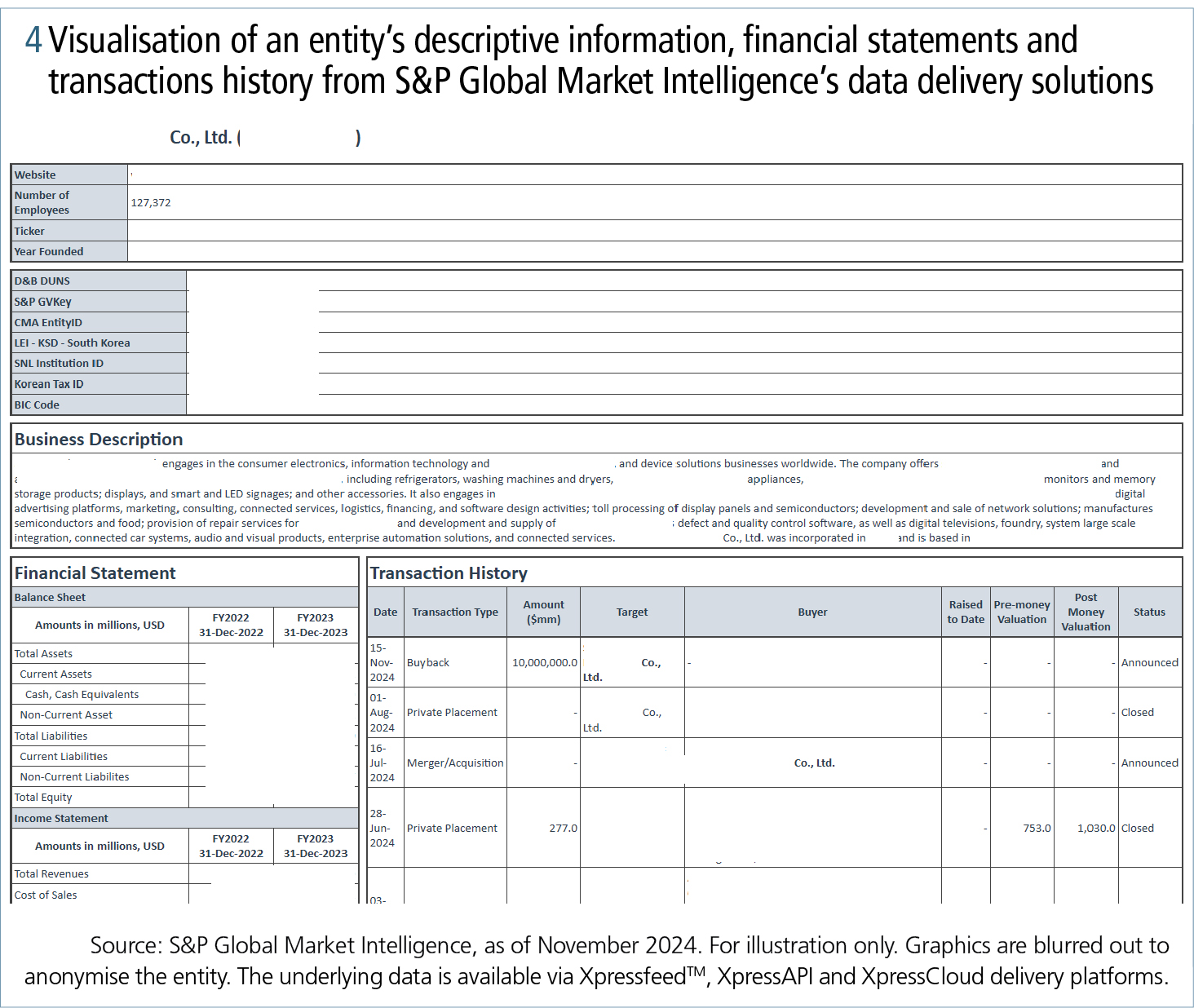
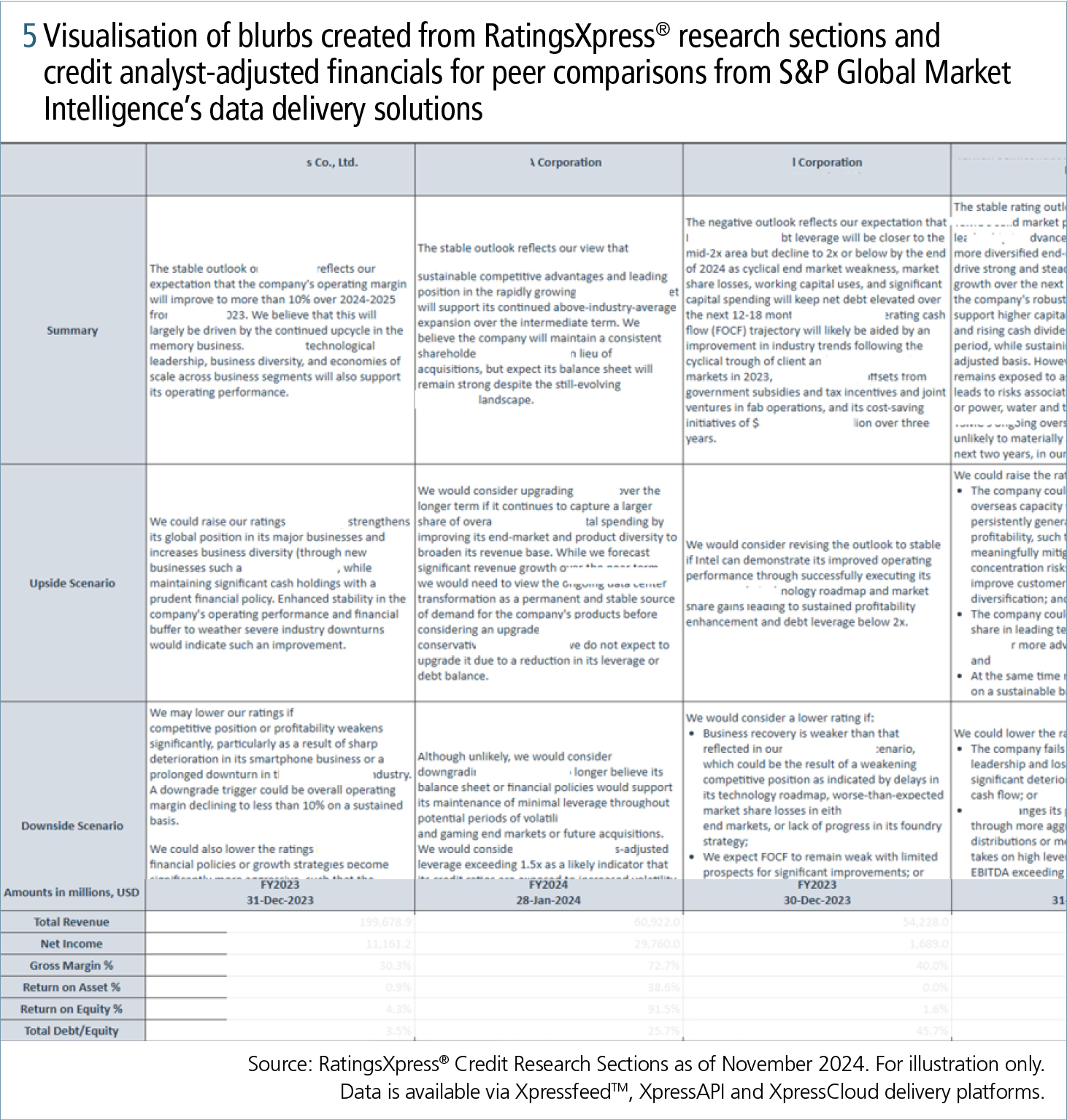
Figures 4 and 5 also provide descriptive information and peer comparisons derived by S&P Global Market Intelligence from S&P Global Ratings’ research text, which can be automatically integrated into credit memos and linked to other datasets by leveraging the cross-reference capabilities of the Business Entity Cross References Service. There is potential for credit memos, in the future, to be populated based on responses to customer-specific chatbot prompts via an application programming interface, which are designed and validated by the customer to ensure consistent and reliable responses tailored to each use case, context, user and product type.
Finally, data coverage is an important consideration when selecting a data solution, as it alleviates the need to manage multiple data pipelines and harmonises data definitions across providers. For entities that are not rated by credit rating agencies, the RiskGauge precalculated credit scores and bond-implied scores from Credit Analytics leverage market- and fundamentals-driven analytical models that deliver more than 880,000 lower-case credit scores for public and private entities, which aims to broadly align with ratings from S&P Global Ratings.
To supplement our extensive coverage, we allow clients to load and score the financials of their counterparties, which they may acquire directly. We are looking to automate these processes in the future to seamlessly expand the universe of credit scores available.
A data-oriented take on whether GenAI lives up to the hype
Ultimately, GenAI relies heavily on data to drive outputs through learning models. While traditional data processes prioritise the timeliness and geographic coverage of data as key competitive advantages – attributes that are still relevant for AI applications – the same GenAI model can yield markedly different results based on factors such as machine readability, consistency of presentation, cross-referencing and indexing, as well as the inherent noise within the data, and so on.
Furthermore, biases present in the data, subsamples of the data or similar content across multiple sources may cause AI/GenAI to develop systematic biases in its outputs at run time. Ultimately, models require data that is used for learning and to be fit-for-purpose at run time – suitable data can help these GenAI projects live up to expectations, hyped or otherwise.
Copyright © 2024 by S&P Global Market Intelligence, a division of S&P Global Inc. All rights reserved.
This material has been created by S&P Global Market Intelligence. S&P Global Market Intelligence’s opinions, quotes, and credit-related and other analyses are statements of opinion by S&P Global Market Intelligence as of the date they are expressed and not statements of fact or recommendations to purchase, hold or sell any securities or to make any investment decisions, and do not address the suitability of any security. This material does not represent the views of S&P Global Ratings, nor did S&P Global Ratings participate in the creation of this material.
These materials have been prepared solely for information purposes based upon information generally available to the public and from sources believed to be reliable. No content (including index data, ratings, credit-related analyses and data, research, model, software or other application or output therefrom) or any part thereof (content) may be modified, reverse-engineered, reproduced or distributed in any form by any means, or stored in a database or retrieval system, without the prior written permission of S&P Global Market Intelligence or its affiliates (collectively, S&P Global). The Content shall not be used for any unlawful or unauthorised purposes. S&P Global and any third-party providers, (collectively S&P Global Parties) do not guarantee the accuracy, completeness, timeliness or availability of the content. S&P Global Parties are not responsible for any errors or omissions, regardless of the cause, for the results obtained from the use of the content. The content is provided on an “as is” basis. S&P Global Parties disclaim any and all express or implied warranties, including, but not limited to, any warranties of merchantability or fitness for a particular purpose or use, freedom from bugs, software errors or defects, that the content’s functioning will be uninterrupted or that the content will operate with any software or hardware configuration. In no event shall S&P Global Parties be liable to any party for any direct, indirect, incidental, exemplary, compensatory, punitive, special or consequential damages, costs, expenses, legal fees or losses (including, without limitation, lost income or lost profits and opportunity costs or losses caused by negligence) in connection with any use of the content even if advised of the possibility of such damages.
S&P Global Market Intelligence’s opinions, quotes and credit-related and other analyses are statements of opinion as of the date they are expressed and not statements of fact or recommendations to purchase, hold or sell any securities or to make any investment decisions, and do not address the suitability of any security. S&P Global Market Intelligence may provide index data. Direct investment in an index is not possible. Exposure to an asset class represented by an index is available through investable instruments based on that index. S&P Global Market Intelligence assumes no obligation to update the content following publication in any form or format. The content should not be relied on and is not a substitute for the skill, judgment and experience of the user, its management, employees, advisors and/or clients when making investment and other business decisions. S&P Global keeps certain activities of its divisions separate from each other in order to preserve the independence and objectivity of their respective activities. As a result, certain divisions of S&P Global may have information that is not available to other S&P Global divisions. S&P Global has established policies and procedures to maintain the confidentiality of certain non-public information received in connection with each analytical process.
Disclosures for this article from S&P Global Market Intelligence
Read more
Revolutionising credit surveillance: part one
Early warning indicators for credit risk changes are key
Sponsored content
Copyright Infopro Digital Limited. All rights reserved.
As outlined in our terms and conditions, https://www.infopro-digital.com/terms-and-conditions/subscriptions/ (point 2.4), printing is limited to a single copy.
If you would like to purchase additional rights please email info@risk.net
Copyright Infopro Digital Limited. All rights reserved.
You may share this content using our article tools. As outlined in our terms and conditions, https://www.infopro-digital.com/terms-and-conditions/subscriptions/ (clause 2.4), an Authorised User may only make one copy of the materials for their own personal use. You must also comply with the restrictions in clause 2.5.
If you would like to purchase additional rights please email info@risk.net