Journal of Risk Model Validation
ISSN:
1753-9579 (print)
1753-9587 (online)
Editor-in-chief: Steve Satchell
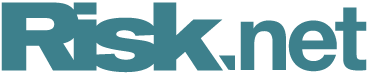
Analyzing credit risk model problems through natural language processing-based clustering and machine learning: insights from validation reports
Szymon Lis, Mariusz Kubkowski, Olimpia Borkowska, Dobromił Serwa and Jarosław Kuparnik
Need to know
- We apply clustering and machine learning techniques to analyze validation reports.
- The XGBoost model outperforms Logistic regression and clustering methods in predicting dimensions of findings from the reports.
- The addition of sentence embeddings, representing the textual context of findings into the predictive factors, significantly improved the overall predictive power.
- The differing precision of the final model in predicting respective dimensions suggests challenges in distinguishing certain deficiencies in findings of analysed reports.
Abstract
This paper employs clustering and machine learning techniques to analyze validation reports. It provides insights into issues related to credit risk model development, implementation and maintenance. Natural language processing is used in the study to classify issues based on findings raised in validation reports. A total of 657 findings, which are raised for selected credit risk models in a large banking institution between 2019 and 2022, are grouped into nine categories representing different validation dimensions. Next, sentence embedding generation from titles and descriptions of findings is used to create predictors in classification models of the validation dimensions. Several clustering methods are compared in order to group similar findings, effectively identifying common issues in each category with an accuracy level of more than 60%. Further, machine learning algorithms, such as logistic regression and extreme gradient boosting (XGBoost), are employed to forecast the finding’s category, with XGBoost achieving 80% accuracy. The top 10 predictive words for each category are also determined.
Copyright Infopro Digital Limited. All rights reserved.
As outlined in our terms and conditions, https://www.infopro-digital.com/terms-and-conditions/subscriptions/ (point 2.4), printing is limited to a single copy.
If you would like to purchase additional rights please email info@risk.net
Copyright Infopro Digital Limited. All rights reserved.
You may share this content using our article tools. As outlined in our terms and conditions, https://www.infopro-digital.com/terms-and-conditions/subscriptions/ (clause 2.4), an Authorised User may only make one copy of the materials for their own personal use. You must also comply with the restrictions in clause 2.5.
If you would like to purchase additional rights please email info@risk.net