Journal of Risk Model Validation
ISSN:
1753-9579 (print)
1753-9587 (online)
Editor-in-chief: Steve Satchell
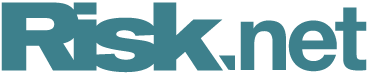
Machine learning prediction of loss given default in government-sponsored enterprise residential mortgages
Need to know
- This research evaluates the performance of various predictive models for residential mortgage Loss Given Default (LGD), highlighting CatBoost and Light Gradient Boost Machine Regressor as the most accurate models in predicting LGD.
- Variables such as the mark-to-market loan-to-value ratio, unpaid balance at default and the role of mortgage servicers are critical in shaping LGD outcomes and for effective risk management.
- The study reveals variations in LGD among fintech firms, banks and nonbanks, illustrating the impact of technological advancements and operational strategies on risk assessments in the mortgage market.
- Our findings establish a benchmark for the validation of LGD models, demonstrating the robustness and accuracy necessary for reliable model validation in the mortgage sector.
Abstract
In the dynamic field of residential mortgage lending, the accurate estimation of loss given default (LGD) is crucial for effective risk management and model validation. This study investigates LGD using machine learning techniques on a data set of conforming loans from government-sponsored enterprises in the United States between January 2012 and March 2018. The research objectives were threefold: to evaluate the predictive capabilities of various machine learning models for LGD that incorporate a comprehensive set of risk factors; to compare LGD across lender types; and to identify the dominant variables influencing LGD outcomes. Ensemble tree-based methods (specifically the CatBoost, the Gradient-Boosting Regressor and Light Gradient-Boosting Machine regressor algorithms) demonstrated superior accuracy, which was further enhanced through hyperparameter tuning. These models highlighted the importance of loan-specific details and broader economic indicators in LGD estimation. The study found that, unlike banks and nonbanks, fintech firms presented distinct LGD patterns, suggesting diverse risk assessment approaches. Key variables impacting LGD predictions included the mark-to-market loan-to-value ratio, the unpaid balance at default, the liquidation timeline and the mortgage servicer as well as regional economic factors. This paper contributes to the methodological advancement in LGD prediction at the origination stage, which is crucial for proactively managing loan portfolios. It contributes to advancing methodological approaches in LGD prediction and risk model validation, offering valuable insights for lenders and policy makers in refining risk frameworks within the evolving mortgage market.
Copyright Infopro Digital Limited. All rights reserved.
As outlined in our terms and conditions, https://www.infopro-digital.com/terms-and-conditions/subscriptions/ (point 2.4), printing is limited to a single copy.
If you would like to purchase additional rights please email info@risk.net
Copyright Infopro Digital Limited. All rights reserved.
You may share this content using our article tools. As outlined in our terms and conditions, https://www.infopro-digital.com/terms-and-conditions/subscriptions/ (clause 2.4), an Authorised User may only make one copy of the materials for their own personal use. You must also comply with the restrictions in clause 2.5.
If you would like to purchase additional rights please email info@risk.net