Journal of Risk Model Validation
ISSN:
1753-9579 (print)
1753-9587 (online)
Editor-in-chief: Steve Satchell
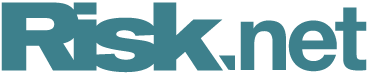
Quantifying credit portfolio sensitivity to asset correlations with interpretable generative neural networks
Need to know
- The paper proposes a new method for quantifying credit portfolio value-at-risk sensitivity to asset correlations using synthetic financial correlation matrices generated by Variational Autoencoders (VAEs).
- Previous work utilized Generative Adversarial Networks (GANs) for generating correlation matrices, but VAEs are chosen for their more interpretable latent space representation.
- By training a VAE on historical correlation matrices, the authors demonstrate the ability to generate synthetic correlation matrices that exhibit expected financial properties, enhancing risk management by providing realistic data augmentation.
- The VAE latent space is found to be useful for capturing crucial factors affecting portfolio diversification and credit portfolio sensitivity to changes in asset correlations, thereby improving the resilience and accuracy of risk management models during backtesting.
Abstract
We propose a novel approach for quantifying the sensitivity of credit portfolio value-at-risk to asset correlations with the use of synthetic financial correlation matrixes generated with deep learning models. In previous work, generative adversarial networks (GANs) were employed to demonstrate the generation of plausible correlation matrixes that capture the essential characteristics observed in empirical correlation matrixes estimated on asset returns. Instead of GANs, we employ variational autoencoders (VAEs) to achieve a more interpretable latent space representation and to obtain a generator of plausible correlation matrixes by sampling the VAE’s latent space. Through our analysis, we reveal that the VAE’s latent space can be a useful tool to capture the crucial factors impacting portfolio diversification, particularly in relation to the sensitivity of credit portfolios to changes in asset correlations. A VAE trained on the historical time series of correlation matrixes is used to generate synthetic correlation matrixes that satisfy a set of expected financial properties. Our analysis provides clear indications that the capacity for realistic data augmentation provided by VAEs, combined with the ability to obtain model interpretability, can prove useful for risk management, enhancing the resilience and accuracy of models when backtesting, as past data may exhibit biases and might not contain the essential high-stress events required for evaluating diverse risk scenarios.
Copyright Infopro Digital Limited. All rights reserved.
As outlined in our terms and conditions, https://www.infopro-digital.com/terms-and-conditions/subscriptions/ (point 2.4), printing is limited to a single copy.
If you would like to purchase additional rights please email info@risk.net
Copyright Infopro Digital Limited. All rights reserved.
You may share this content using our article tools. As outlined in our terms and conditions, https://www.infopro-digital.com/terms-and-conditions/subscriptions/ (clause 2.4), an Authorised User may only make one copy of the materials for their own personal use. You must also comply with the restrictions in clause 2.5.
If you would like to purchase additional rights please email info@risk.net