Journal of Risk Model Validation
ISSN:
1753-9579 (print)
1753-9587 (online)
Editor-in-chief: Steve Satchell
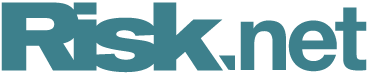
Expected shortfall model based on a neural network
Sanja Doncic, Nemanja Pantic, Marija Lakićević and Nikola Radivojević
Need to know
- The paper presents a model that represents a combination of ES models based on EVT and neural networks.
- The model successfully takes advantage of the application of neural networks and traditional ES models.
- The model meets all the criteria for the validity of the Basel III standard.
Abstract
Considering both the limitations of traditional models of value-at-risk and expected shortfall (ES) for risk estimation in the context of the Basel standards and the possibilities of applying neural network models for risk estimation purposes, our paper presents a new ES model or, more specifically, an ES-extreme-value-theory (ESEVT) model improvement, as it is a combination of the standard multilayer perceptronmodel and the ES model based on EVT. This model exploits the advantages of both approaches in estimating financial risk. The model was tested on 15 example indexes of emerging European capital markets. The model quality assessment against the ES-EVT model used mean squared error, while model validation in the context of the Basel III standards was done using Berkowitz’s ES backtesting, based on bootstrap simulation, and Acerbi and Szekely’s first method. The results obtained imply that our neural network application improves ES-EVT model performance.
Copyright Infopro Digital Limited. All rights reserved.
As outlined in our terms and conditions, https://www.infopro-digital.com/terms-and-conditions/subscriptions/ (point 2.4), printing is limited to a single copy.
If you would like to purchase additional rights please email info@risk.net
Copyright Infopro Digital Limited. All rights reserved.
You may share this content using our article tools. As outlined in our terms and conditions, https://www.infopro-digital.com/terms-and-conditions/subscriptions/ (clause 2.4), an Authorised User may only make one copy of the materials for their own personal use. You must also comply with the restrictions in clause 2.5.
If you would like to purchase additional rights please email info@risk.net