Journal of Network Theory in Finance
ISSN:
2055-7795 (print)
2055-7809 (online)
Editor-in-chief: Ron Berndsen
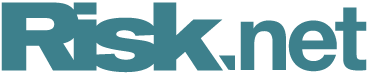
Large vector autoregressive exogenous factor (VARX) model with network regularization
Need to know
- Vector autoregression with exogenous variables (VARX) has been used as a powerful model for portfolio returns. We provide a regularization method, based on network analysis, that reduces the dimensionality problem and makes VARX applicable when the number of time series becomes large.
- We illustrate our method on the series of returns of over 600 hedge funds with over US$100 million and we use their 13F filings to construct a network of overlapping portfolios.
- Carhart's four-factor model is integrated in the model in order to provide exogenous variables for the excess return of the portfolios. Our model achieves better prediction performances, indicating that the topology of portfolios due to overlapping asset holdings can be applied as a structure for the penalty function.
- It works especially well when the fund returns are homogeneous. Homogeneity is defined in terms of belonging to the same cluster, whereby clustering is based on orthogonal wavelet decomposition in combination with hierarchical clustering.
Abstract
The vector autoregressive model has long been used for portfolio analysis, while a recent extension (VARX) incorporates exogenous factors. Despite its increased forecasting precision, the applicability of the VARX model is seriously hampered in the absence of sparsity, as the number of coefficients to be estimated grows quadratically with the number of series. We introduce a novel regularization method for the VARX model in the context of portfolios, where weighted links between portfolios are used to construct a penalty function for the autoregressive parameter matrixes. To test the prediction performance of the new method, we first cluster the time series into several groups using wavelet decomposition and hierarchical clustering, after which we construct data sets of different homogeneity. Our method is advantageous in two ways: the computation time for the model is significantly reduced, and the forecasting precision of the model is enhanced by 50% compared with existing regularization methods.
Copyright Infopro Digital Limited. All rights reserved.
As outlined in our terms and conditions, https://www.infopro-digital.com/terms-and-conditions/subscriptions/ (point 2.4), printing is limited to a single copy.
If you would like to purchase additional rights please email info@risk.net
Copyright Infopro Digital Limited. All rights reserved.
You may share this content using our article tools. As outlined in our terms and conditions, https://www.infopro-digital.com/terms-and-conditions/subscriptions/ (clause 2.4), an Authorised User may only make one copy of the materials for their own personal use. You must also comply with the restrictions in clause 2.5.
If you would like to purchase additional rights please email info@risk.net