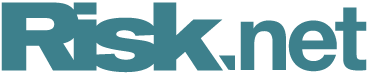
This article was paid for by a contributing third party.More Information.

Vulnerabilities arise in financial services as AI and machine learning use balloons

The scale at which financial services firms are adopting artificial intelligence and machine learning continues to grow, bringing with it new dimensions of risk and vulnerability. In a recent Risk.net webinar sponsored by TCS, experts discussed approaches to bias and discrimination, governance and explainability of models, and the most critical data issues facing firms. Here we explore three themes that emerged from the discussion
Bad bias and discrimination
Bias leading to unfair outcomes or discrimination can occur first in the historical data used to train predictive models, and second in the new data used by that model to make future decisions. The webinar’s panellists noted that bad bias within datasets may impact the ability of AI models to accurately quantify the probability of a default or risk loss event. This could result in unfair decisions and higher misclassification rates. As well as exposing a firm to higher credit risk, this could also lead to customers being wrongly denied access to credit, leading to reputational risk for firms. On a positive note, news sentiment analytics, which uses natural language processing and machine learning, is being used successfully in credit risk management.
UK General Data Protection Regulation specifies the risk of discrimination that comes with automated decision-making, one webinar panellist explained. The Information Commissioner’s Office (ICO) has produced guidance for organisations using AI to process personal data on how to mitigate the risk of bias and discrimination. As part of a new framework the ICO is developing to audit these firms, assurance and investigations teams will scrutinise the technical and organisational measures they are taking to mitigate those risks.
The panellists agreed on the potential of using data and AI to reduce bad bias, and said firms are already using AI tools to try to mitigate these risks. By assessing biases in the underlying datasets they aim to ensure the quality of the data used in model development and in model execution is both consistent and complete. One panellist, a regulator, said that firms should be considering two new concepts in the use of machine learning and AI: representation and completeness of their data.
Explainability
Being able to explain model outputs is vital for the financial services industry; webinar participants said that explainability provides an understanding of rate rationalisation as well as outcomes, such as a denial of credit or a transaction being marked as fraudulent.
A multifaceted, multilayered machine learning model changes with the inputs and with the outcomes. With the governance layer no longer static, firms will need dynamic governance processes to continue to provide explainability. The regulator noted that it runs some of its own machine learning models as challenger models to their current ways of working.
As a first line of defence, firms are educating their modellers to build explainability into their designs. Where the models are not explainable, firms are using a layered approach and assessing outcomes to ensure these are not biased. If there is bias, the ongoing challenge is to try to understand whether the bias is a feature of the model, the dataset on which it was developed or the dataset it is running on.
Data issues
The most successful adoption of AI and machine learning occurs when firms are able to leverage new datasets previously unavailable because of limitations to econometric techniques. However, as one panellist remarked, the speed, scale and complexity of AI models, along with a number of data-related issues, raise new regulatory challenges, both in terms of the safety and soundness of individual firms, and potential systemic risks to financial stability.
Increasingly, firms are operating several AI models within a network to reach particular outcomes quickly. The outputs, such as actions, algorithms and unstructured or quantitative data become even more complicated. The regulator urged responsible innovation and awareness of the implications of large banks and incumbent players using these technologies.
The most critical issues industry participants faced in debiasing and enhancing accountability are around data quality. The market participants on the panel agreed that data quality is the linchpin of good modelling, which is inextricably linked to traceability and lineage. They explained that transformations occur through the lineage flow that impacts data quality. Understanding and correctly applying the controls they have on the lineage flow is an ongoing challenge. To this end, firms are using AI tools to understand logic and data flows.
In summary
AI and machine learning come with significant risks arising from bad bias. There are ongoing challenges in mitigating those risks and explaining model outputs. Understanding their data quality controls is vital to firms’ ability to tackle these issues. There is a comforting prospect in the potential panellists foresaw in the use of AI and machine learning to manage its own ills – for example, to reduce bad bias, understand data lineage and traceability, and run dynamic governance processes to maintain explainability.
Register to watch the webinar, Propelling business innovation and efficiency in financial services with AI
The panellists were speaking in a personal capacity. The views expressed by the panel do not necessarily reflect or represent the views of their respective institutions.
Sponsored content
Copyright Infopro Digital Limited. All rights reserved.
As outlined in our terms and conditions, https://www.infopro-digital.com/terms-and-conditions/subscriptions/ (point 2.4), printing is limited to a single copy.
If you would like to purchase additional rights please email info@risk.net
Copyright Infopro Digital Limited. All rights reserved.
You may share this content using our article tools. As outlined in our terms and conditions, https://www.infopro-digital.com/terms-and-conditions/subscriptions/ (clause 2.4), an Authorised User may only make one copy of the materials for their own personal use. You must also comply with the restrictions in clause 2.5.
If you would like to purchase additional rights please email info@risk.net