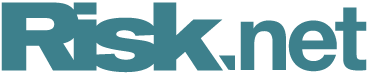
This article was paid for by a contributing third party.More Information.
Accelerating the evolution of credit decisioning and modelling
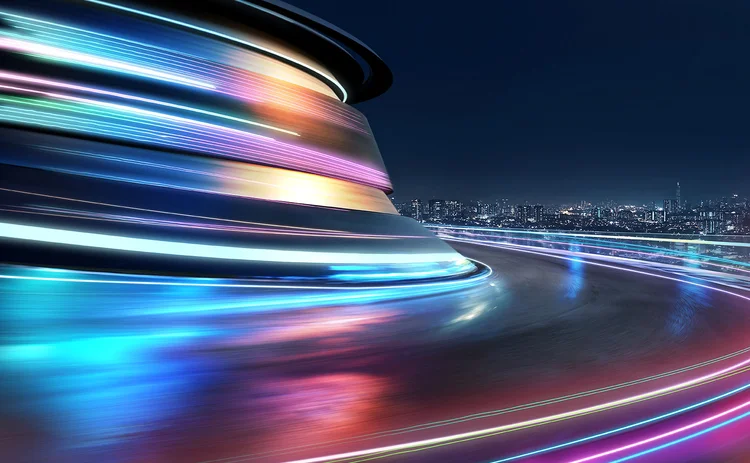
Anthony Mancuso, director, global head of risk modelling and decisioning at SAS, explains the importance of developing a fully capable credit modelling lifecycle to empower non-specialist personnel, and offers insight into its own solutions to this end, which can provide a seamless experience across business lines and improve the speed of credit decisions
Globally, credit modelling has undergone significant transformation, driven by factors such as digitalisation, adoption of artificial intelligence and machine learning, and regulatory demands. Financial services providers – both traditional banks and newer financial technology players – want to provide consumers with a consistent and contextual experience across the credit lifecycle.
In addition, the Covid‑19 pandemic has put a greater emphasis on the ability to assess impacts of macroeconomic changes on portfolios using a range of scenarios, but also highlighted inefficiencies in the risk model lifecycle. Given the speed of some of the changes in economic conditions, organisations are looking for more agility to respond by making changes to models and policy rules. As the demand for more and better models increases, firms are looking for efficiency benefits by automating parts of the risk model lifecycle. A key lesson from the pandemic is that organisations that are digitally enabled are much better able to respond to new business and regulatory demands.

These requirements have placed greater demand on financial services providers to deal with large datasets, develop state-of-the-art risk models and integrate analytics in decisioning processes, all while meeting and maintaining model governance requirements. An example of the challenge that banks face is a credit risk decline. When a bank declines any customer for a credit risk reason, it must explain exactly why it is being declined. And, if the bank uses a ‘black box’ that utilises a complex algorithm around a customer’s spending patterns, for example – but the bank cannot explain why – then that is a problem for the bank. Banks have very specific regulatory and compliance regulations that require them to provide the customer with details as to why they may be declined.
So it is important to build more meaningful credit models that are not black boxes that enable the business to better understand credit risk and help the bank’s customers fully understand decisions related to their requests for credit.
To meet these demands, SAS developed an integrated environment to support the end-to-end credit lifecycle – from loan originations to account management to collections and recoveries. It offers a standardised process for a comprehensive range of risk models – from regulatory capital and provisioning models, such as through-the-cycle or forward-looking probability of default, loss given default and exposure-at-default estimation, applications and behavioural scoring, to models fulfilling auxiliary functions, such as income estimation, prepayment and propensity models.
Today’s credit modelling lifecycle needs a broad set of capabilities in data preparation and analytics, with open-source integration, model governance and lineage, enabling a guided experience for non-specialist personnel to quickly accomplish what once took specialised resources weeks or months.
Deploying models to decisioning engines
Data engineers and scientists access and onboard any data, be it granular transactional data, data from third-party providers, or traditional or alternative data in structured or unstructured formats.
Data scientists develop risk models, be they statistical or modern machine learning, using their preferred open-source language (such as R or Python), or the easy-to-use SAS interfaces. When ready, these models can be deployed to any combination of in-memory, in-database, batch, real-time or streaming systems with the click of a button, eliminating the need for recoding. A comprehensive set of model monitoring metrics for backtesting, benchmarking and compliance is built in.
All of the above are available for a variety of model types, including linear and non-linear modelling, scorecard development, including optimal grouping methods, forecasting, optimisation, simulation, machine learning, deep learning and text analytics.
A critical market differentiator is the ease with which models of all types can be deployed to decisioning engines. SAS risk decisioning provides a modernised decision tool for credit decision automation, integrating analytically powered operational decisions into risk management processes, such as loan originations, limit management and collections. By combining business rules management, advanced analytics and decision governance, a range of operational decisions can be automated.
As an illustration of what can be achieved, one of the largest global banks chose SAS to transform their enterprise decisioning platform and build strong digital capability for the future. They want to offer customers a seamless experience across business lines: credit risk, collections and fraud detection. SAS supports these digital transformation objectives to offer consistent and contextual customer journeys. Furthermore, during the proof-of-value process, SAS has greatly improved the speed of credit decisions (straight-through processing rates have risen from 60% to 75%) while the deployment of models, previously six months on average, can now be performed with SAS in minutes – whether open source or SAS.
Credit risk & modelling – Special report 2021
Read more
Sponsored content
Copyright Infopro Digital Limited. All rights reserved.
As outlined in our terms and conditions, https://www.infopro-digital.com/terms-and-conditions/subscriptions/ (point 2.4), printing is limited to a single copy.
If you would like to purchase additional rights please email info@risk.net
Copyright Infopro Digital Limited. All rights reserved.
You may share this content using our article tools. As outlined in our terms and conditions, https://www.infopro-digital.com/terms-and-conditions/subscriptions/ (clause 2.4), an Authorised User may only make one copy of the materials for their own personal use. You must also comply with the restrictions in clause 2.5.
If you would like to purchase additional rights please email info@risk.net