Journal of Financial Market Infrastructures
ISSN:
2049-5404 (print)
2049-5412 (online)
Editor-in-chief: Manmohan Singh
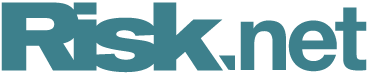
Using payments data to nowcast macroeconomic variables during the onset of Covid-19
Need to know
- Policymakers need understand the current state of the economy to effectively respond to crisis, such as ongoing Covid-19 pandemic.
- Major macroeconomic indicators are typically released with a delay. This makes predicting the economy during a crisis challenging.
- In this paper, we help address this challenge by building a nonlinear nowcasting model by using retail payments system data with flexible machine learning methods.
Abstract
The Covid-19 pandemic and its resulting public health mitigation measures have caused large-scale economic disruption globally. At this time, there is an increased need to predict the macroeconomy’s short-term dynamics to ensure the effective implementation of fiscal and monetary policy. However, economic prediction during a crisis is challenging because of the unprecedented economic impact of such an event, which increases the unreliability of traditionally used linear models that employ lagged data. We help to address these challenges by using timely retail payments system data in linear and nonlinear machine learning models. We find that, compared with a benchmark, our model has a roughly 15–45% reduction in root mean square error when used for macroeconomic nowcasting during the global financial crisis. For nowcasting during the Covid-19 shock, our model predictions are much closer to the official estimates.
Copyright Infopro Digital Limited. All rights reserved.
As outlined in our terms and conditions, https://www.infopro-digital.com/terms-and-conditions/subscriptions/ (point 2.4), printing is limited to a single copy.
If you would like to purchase additional rights please email info@risk.net
Copyright Infopro Digital Limited. All rights reserved.
You may share this content using our article tools. As outlined in our terms and conditions, https://www.infopro-digital.com/terms-and-conditions/subscriptions/ (clause 2.4), an Authorised User may only make one copy of the materials for their own personal use. You must also comply with the restrictions in clause 2.5.
If you would like to purchase additional rights please email info@risk.net