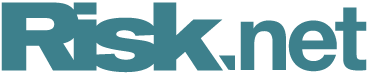
A step closer to the perfect volatility model
Research on ‘rough volatility’ gives fresh insight into financial fluctuations, quant expert explains
Despite centuries of academic study, nobody knows for sure what makes the price of financial assets move. The best we can do is make a model of how they behave.
This was the argument of Louis Bachelier, who proposed the first mathematical model of prices in 1900: the Brownian motion, from which he derived an option pricing formula. Quite remarkably, the empirical data available to him at the time revealed what is now called the volatility smile.
After 120 years of improvements and refinements, research has moved the frontier forward again. Last year, Aditi Dandapani, Paul Jusselin and Mathieu Rosenbaum proposed the Quadratic Rough Heston Model, probably the most realistic model of financial price series to date.
The final part of the puzzle, however, is to understand how prices interact with the behaviour of countless traders, each with his or her own investment style, trading frequency, risk limits etc. Interestingly, recent study strongly suggests that markets self-organise in subtle way, hovering between stability and instability. This could be the missing link that researchers have been looking for.
Standing on the shoulders of giants
For many years, the only modification to Bachelier’s proposal was to consider that Brownian motion describes log-prices, not prices themselves. Apart from preventing prices from becoming negative, this modification doesn’t seriously tackle any of the flaws of the Bachelier model.
Brownian finance reached its zenith with Fisher Black and Myron Scholes’s landmark 1973 paper, The pricing of options and corporate liabilities. The paper shows that perfect delta-hedging is possible – but only because, in the Black-Scholes world, price jumps are absent and crashes impossible.
It’s a hazardous assumption. The fat-tailed distribution of returns had been highlighted as early as 1963 by mathematician Benoit Mandelbrot, who further noted: “Large changes tend to be followed by large changes, of either sign, and small changes tend to be followed by small changes.” This effect is now commonly referred to as “volatility clustering”, and was captured by the extended family of Garch models.
It took the violent crash of October 1987, exacerbated by Black-Scholes delta-hedging, for new models to emerge. A model published in 1993 by Steven Heston is among the most famous post-Black-Scholes models, encapsulating volatility clustering within a structure of continuous-time Brownian motion. Like Garch, the Heston model predicts that volatility fluctuations decay over a single timescale – in other words that periods of high or low volatility have a well-defined duration. This is not compatible with market data: volatility bursts can last anything between a few hours and a few years.

Mandelbrot had been mulling this for a long while, and proposed in 1974 a model to describe a very similar phenomenon in turbulent flows, called “multifractality”. He adapted his theory in 1997 to describe currency exchange rates, before Emmanuel Bacry, Jean-Francois Muzy and Jean Delour formulated in 2000 a more convincing, causal version of the model, which they called the Multifractal Random Walk (MRW).
With a single extra parameter, interpreted as a kind of volatility of volatility, the MRW captures many important empirical observations, such as fat-tailed distribution of returns and long memory of volatility fluctuations.
Fifteen years later, the Rough Volatility model was born. Coined by Jim Gatheral, Thibault Jaisson and Mathieu Rosenbaum, the model can be seen as an extension of the MRW with an extra parameter that enables the roughness of volatility to be tuned; in the MRW model it is set in stone.
And indeed, empirical data suggests that volatility is slightly less rough than that posited by the MRW.
But in the meantime, a major breakthrough came in 2009 when Gilles Zumbach noticed a subtle, yet crucial aspect of empirical financial time series: they are not statistically invariant upon time reversal. Past and future are not equivalent, whereas almost all models to that date, including the MRW, did not distinguish past from future. More precisely, past price trends, whether up or down, lead to higher future volatility, but not the other way round.
Taking stock of this effect, Dandapani, Jusselin and Rosenbaum proposed their Quadratic Rough Heston Model, following some work by Pierre Blanc, Rémy Chicheportiche, Jonathan Donier and myself. The model is a synthesis of all the ideas reviewed above and reproduces almost all known statistical observations about financial time series. In particular, it provides a natural solution to a long-standing puzzle, namely the joint calibration of the volatility smile of the S&P 500 and Vix options, which had eluded quants for many years. A missing ingredient was indeed the Zumbach effect.
The beginning of the end
The latest version of the Rough Volatility model sets a high bar, purely from an engineering point of view. But there is one remaining challenge: to explain how the model interfaces with the underlying flow of buy and sell orders from market-makers, high-frequency traders and many other participants. Parts of the story are already clear; in particular, Jaisson, Jusselin and Rosenbaum argue the Rough Volatility model is connected with an incipient market instability that justifies the rough, multi-timescale nature of volatility.
But what is the self-organising mechanism through which all markets appear to settle close to such a critical point? Stocks, futures, commodities and exchange rates share very similar statistical features, in particular in the tails. Could this scenario allow us to understand why financial time series all look so much alike?
Beyond being the denouement of a 120-year odyssey, reaching this final stage would allow us to see the final model as a robust, trustworthy framework for risk management and derivatives pricing – and not just a figment of our mathematical imagination.
Jean-Phillipe Bouchaud is chairman of Capital Fund Management and member of the Académie des Sciences
Only users who have a paid subscription or are part of a corporate subscription are able to print or copy content.
To access these options, along with all other subscription benefits, please contact info@risk.net or view our subscription options here: http://subscriptions.risk.net/subscribe
You are currently unable to print this content. Please contact info@risk.net to find out more.
You are currently unable to copy this content. Please contact info@risk.net to find out more.
Copyright Infopro Digital Limited. All rights reserved.
As outlined in our terms and conditions, https://www.infopro-digital.com/terms-and-conditions/subscriptions/ (point 2.4), printing is limited to a single copy.
If you would like to purchase additional rights please email info@risk.net
Copyright Infopro Digital Limited. All rights reserved.
You may share this content using our article tools. As outlined in our terms and conditions, https://www.infopro-digital.com/terms-and-conditions/subscriptions/ (clause 2.4), an Authorised User may only make one copy of the materials for their own personal use. You must also comply with the restrictions in clause 2.5.
If you would like to purchase additional rights please email info@risk.net
More on Comment
An AI-first approach to model risk management
Firms must define their AI risk appetite before trying to manage or model it, says Christophe Rougeaux
Op risk data: At Trafigura, a $1 billion miss in Mongolia
Also: Insurance cartels, Santander settlement and TSB’s “woeful” customer treatment. Data by ORX News
UST repo clearing: considerations for ‘done-away’ implementation
Citi’s Mariam Rafi sets out the drivers for sponsored and agent clearing of Treasury repo and reverse repo
Op risk data: Macquarie mauled by securities mismarks
Also: Danske’s costliest branch, tedious times for TD, and WhatsApp won’t stop. Data by ORX News
Climate stress tests are cold comfort for banks
Flaws in regulators’ methodology for gauging financial impact of climate change undermine transition efforts, argues modelling expert
Op risk data: Shady loans robbing Reliance of $1.1bn
Also: H20’s less-than-liquid holdings, Ripple ripped for $125m, and more WhatsApp slaps expected. Data by ORX News
FX algo users change tack to navigate market doldrums
BestX data finds traders ditching TWAP in favour of more opportunistic execution styles
Op risk data: Payday lender Skytrail sees $1.4bn disappear
Also: Cartel claims cost European bond dealers dearly, plus oil price gouging and crypto cover-ups. Data by ORX News