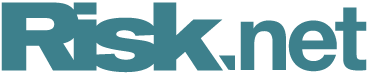
This article was paid for by a contributing third party.More Information.

The future of operational risk management

As the efficiency of operational risk management remains a top priority and pressure to maximise value increases, emerging technology could prove crucial. Nitish Idnani, leader of oprisk management services at Deloitte, explores how the oprisk management space could look in the future if it continues its current evolution, and discusses the potential impact of key technologies
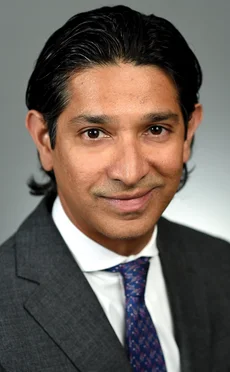
The efficacy and efficiency of operational risk management continue to be a major priority in today’s business climate. The ability to demonstrate the value of oprisk management frameworks – with risk managers being increasingly expected to do more with less – is increasing. This pressure is creating an incentive for risk leaders to explore and embrace new technologies and techniques that can help improve their programmes.
Predictive risk intelligence and the use of advanced analytics for pattern recognition, and correlation and causal analysis give oprisk managers a head start on identifying the build-up of potential risk and the need for remedial action. Banks should seize the opportunities made possible by today’s advanced tools and the ubiquity of vast amounts of data.
Predictive risk analytics, machine learning and artificial intelligence can help efficiently build and mine large and complex datasets that combine traditional Basel Committee on Banking Supervision oprisk loss data with other data sources, including transaction data; non-transaction data such as human resources, compliance and other internal management information; and external data such as sensing data, social media, customer complaints and regulatory actions. These aggregated datasets provide billions of data combinations that can drive improved risk results and insights, and may increase the likelihood of uncovering patterns and correlations that were previously not noticed until too late, if at all.
Over the past 12–18 months, there have been moves toward predictive risk intelligence. Globally, a greater number of organisations are trying to make their oprisk management programmes more forward-looking. Banks have long been interested in finding ways to enhance their traditional oprisk management practices. Although historical data on operational losses is still the baseline for complying with regulatory capital rules, such data has always been a blunt instrument for controlling loss and risk profiles. In the past, the necessary tools and technologies to make more insightful correlations and predictions did not exist. Occasionally, experienced oprisk practitioners – with help from data scientists – have used intuition to identify patterns between risk profiles, losses and events in legacy models. However, this generally did not happen until long after the event occurred, and was often limited to situations where extreme data variations were clearly visible – situations so infrequent they had no real predictive value.
The main driver of careful design for most data models is to build a foundation that positions an organisation to acquire better intelligence around a subject. Patterns and behaviours can help organisations understand, manage or predict the forces that drive them. Given the nature of oprisk, even predictable patterns and behaviours can be challenging to identify consistently. Now might be the time to revisit the foundation of the traditional oprisk data model – including the data collected.
One instructive way might be to learn from techniques derived from outside risk management, such as customer marketing and sales. These disciplines have well-grounded techniques to help understand customer behaviour to generate additional sales and further build customer loyalty. To create these benefits, retail organisations had to monitor data from numerous sources to understand the full profile, preferences and buying patterns of customer behaviour. This ranged from monitoring and understanding customer traffic in retail institutions to developing merchandising and designing websites and applications to increase sales and customer loyalty. In essence, this was a period of trial and error in understanding the customer interaction and engagement environment. Once built, it continues to evolve, adapt and improve. In oprisk management, these successes can be emulated by collecting wide-ranging data through systems, applications and processes – and through human interactions – then derive meaningful patterns and behaviours in line with the unique risk challenges of individual organisations and lines of business.
New data science applications
Some may say the current data environment is too vast and expansive to effectively monitor and evaluate. Nevertheless, with new big data science techniques, institutions can now build these capabilities with increasing ease and less investment. The challenge typically lies in scoping what type and range of data will be relevant to obtain the desired model results. This is where leveraging business, as well as the experience of the oprisk manager, will continue to be important.
Figure 1 is an illustrative data architecture that highlights legacy Basel Committee components and a broader set of data sources required for predictive analysis. Broadly, this architecture includes:
1. Data sources, including systems interfaces, messaging and data flows for bringing together disparate data.
2. Quantification calculators – the models that combine internal and external loss data to produce loss estimates, for example, current capital quantification and/or Comprehensive Capital Analysis and Review of operational stress capital.
3. Core predictive analytics to identify patterns, correlations and causation that are otherwise difficult to spot.
4. Reporting capabilities – the mechanism for communicating current and potential oprisk exposures to senior management and the business line units that manage oprisk on a daily basis, and integration back into traditional oprisk management processes.
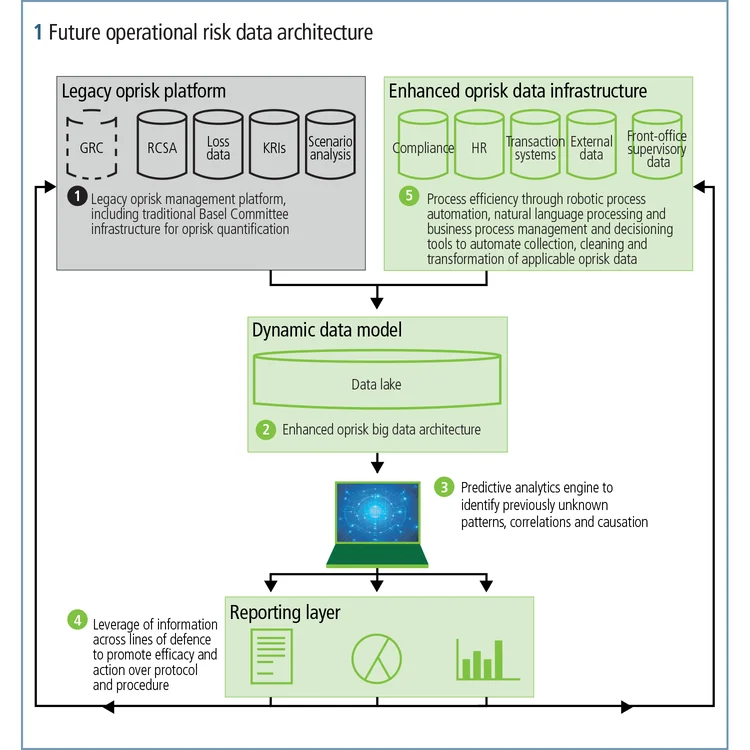
Multiple vendors serve the predictive analytics market – established and nascent emerging companies. While the solutions offered by various companies have points of differentiation, most predictive analytics solutions offer some core features and capabilities, including support for data preparation and selection, insight generation and visualisation.
Many vendors also offer predictive modelling capabilities that use data mining and probability to forecast outcomes. Each model is made up of multiple predictors – variables likely to influence future results. Once data is collected for relevant predictors, a statistical model is formulated. The model may employ simple linear equations or be a more complex neural network that is mapped through sophisticated software. As additional data becomes available, the statistical analysis model is revalidated or revised. Many vendors are also starting to offer machine learning capabilities to help with the process of identifying the most appropriate – in effect, the strongest – predictive model for a given dataset.
Many vendors also offer embedded predictive analytics capabilities that can be used in the context of business processes. Embedded analytics can help organisations gain the visibility required to understand current and historical results, as well as the causal factors influencing them. Embedded predictive analytics also enable organisations to predict system health and trigger alerts or to recommend corrective actions, which can help ensure systems are performing as intended.
Organisations have also begun the journey to evolve their oprisk architectures. The data components and infrastructure that support oprisk are beginning to shift to include a broader definition of the relevant data elements, and predictive analytics and modelling. As oprisk management continues to mature, its future state is likely to look very similar to what has been described in this article.
About Deloitte
Deloitte helps organisations effectively navigate business risks and opportunities – from strategic, reputation and financial risks to operational, cyber and regulatory risks – to gain competitive advantage. We apply our experience in ongoing business operations and corporate lifecycle events to help clients become stronger and more resilient. Our market-leading teams help clients embrace complexity to accelerate performance, disrupt through innovation and lead in their industries.
The author
Nitish Idnani leads Deloitte Risk and Financial Advisory’s operational risk management services team. He can be contacted via email.
As used in this document, “Deloitte” means Deloitte & Touche LLP, a subsidiary of Deloitte LLP. Please see www.deloitte.com/us/about for a detailed description of our legal structure. Certain services may not be available to attest clients under the rules and regulations of public accounting.
This article contains general information only and Deloitte is not, by means of this feature, rendering accounting, business, financial, investment, legal, tax or other professional advice or services. This article is not a substitute for such professional advice or services, nor should it be used as a basis for any decision or action that may affect your business. Before making any decision or taking any action that may affect your business, you should consult a qualified professional adviser. Deloitte shall not be held responsible for any loss sustained by any person who relies on this article.
Sponsored content
Copyright Infopro Digital Limited. All rights reserved.
As outlined in our terms and conditions, https://www.infopro-digital.com/terms-and-conditions/subscriptions/ (point 2.4), printing is limited to a single copy.
If you would like to purchase additional rights please email info@risk.net
Copyright Infopro Digital Limited. All rights reserved.
You may share this content using our article tools. As outlined in our terms and conditions, https://www.infopro-digital.com/terms-and-conditions/subscriptions/ (clause 2.4), an Authorised User may only make one copy of the materials for their own personal use. You must also comply with the restrictions in clause 2.5.
If you would like to purchase additional rights please email info@risk.net