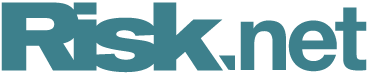
Equity derivatives house of the year: UBS
Asia Risk Awards 2018

UBS’s Asia equities business is leaning on machine learning and artificial intelligence models in Asia to boost pricing accuracy, hedging effectiveness, volumes and risk recycling. They are being deployed by UBS’s equities business to improve the way the bank selects, prices and hedges risk. The machines are already reaping results.
The application of machine learning leverages on enhancements the Swiss bank has made in the automation of its structured products platform. A majority of UBS’s structured exotics flow with bank intermediary clients across Japan, Hong Kong, Singapore and Taiwan is now almost fully automated.
Clients can see a real-time price and trades can be executed, booked, hedged, monitored and processed post-trade with minimal sales staff input, Vikesh Kotecha, managing director, head of equity derivatives at UBS in Hong Kong, says.
“We have enhanced and streamlined pricing, order-taking, documentation and post-trade analytics; it’s a very smooth set-up,” Kotecha says.
The bank’s machine learning and data analytics efforts are led out of Asia, with a team of quants and data scientists based in Hong Kong and Shanghai. In Shanghai, it has a 70-member quants team and some of the solutions developed by the team is being deployed across UBS’s global offices.
These efforts have allowed the bank to move quickly and offer solutions to clients proactively and often ahead of competitors.
Behind the scenes, the bank has “been collecting all of the previously scattered data and are now building analytics on it. The majority of structured exotics flow across Japan, Hong Kong, Singapore and Taiwan is already automated,” Kotecha explains. “Machine learning simply levers on the automation of the structured products platform and allows us to grow market share and be more selective with the risk we take.”
The enhanced automation generates the ‘big data’ necessary to develop predictive analytics and also allows UBS to process in excess of 30,000 trades each month, he says. The electronification of the flows is also providing the bank with a huge pool of real-time data on which predictive analytics are being developed. Those analytic technologies dissect patterns in incoming quotes and compare against the bank’s risk.
This is driving improvements in the accuracy of pricing, hedging effectiveness and understanding of the appropriate market share to target for different trades. It also allows the bank to take pre-emptive positions on risk-recycling trades with clients, creating a virtuous circle in which automation begets better data, which in turn begets more automation.
We have enhanced and streamlined pricing, order-taking, documentation and post-trade analytics; it’s a very smooth set-up
Vikesh Kotecha, UBS
Remarkably, the volume of trades now automated even includes some that rely on bespoke contractual elements. Eliminating manual input on these trades not only means better risk management, but also quicker quote processing. The dividends, in terms of more effective price hedging and establishing the optimum market share for different trades, are already apparent, especially in Japan.
“This has really helped in Japan where quicker processing has contributed to a 50% increase in structured flow volumes year-to-date,” says Takayuki Suzuki, head of Japan equity derivatives sales at UBS in Tokyo. “It is not unusual for Japanese banks to ask for specialised quotes, which include a specific strike or barrier. Elsewhere, quotes tend to be generated manually, but, of course, end-clients want the quotes as soon as possible.”
Investment in automation and machine learning is also helping UBS manage the explosive growth volumes it has seen this year in listed warrants and callable bull bear contracts (CBBCss) in Hong Kong. Improvements have been made to risk recycling through the introduction of trader-assisted decision-support systems for hedging and pricing, and the automation of delta hedging.
As of the end of June 2018, UBS was number one for CBBCs with a market share of 19.45% in turnover. On CBBCs and listed warrants overall, UBS was number two with a market share of 15.7% in turnover among all 12 issuers. The number of warrants and CBBCs issued by UBS also grew by more than 150% year-on-year and the number of underlyings covered increased by 43% to 167 in 2017. “The feedback we’ve had from them has been very positive,” says Kotecha.
Managing autocallable risks
UBS has also bolstered the risk management of its growing autocall books this year through the application of quantitative analysis techniques, and growing risk transfer for flow trades through covariance swaps and knock-in correlation swaps with institutional clients.
A stochastic local volatility model developed by UBS quants is now being used for the risk management of autocallable products. While most banks have similar models, they tend to use it only for valuation adjustments, not for risk management as it is now being applied at UBS.
Developing a better understanding of the interplay between index spot prices and volatility in range-bound markets was the key objective for using the model as a risk management tool.
In a market sell-off the correlation between the spot price of a particular equity index and volatility would normally be negative – if the spot falls, then volatility rises. This dynamic can reverse, however, if index had previously remained range bound for a prolonged period.
A positive correlation between spot and volatility has materialised on occasion in the past, with the Nikkei 225 index in 2012 and, more recently, on the Eurostoxx 50 index in 2015. In both instances dealers suffered big hedging losses. Kotecha says the use of a stochastic local volatility model means UBS can now properly account for unusual spot and volatility correlations in range-bound markets in the risk management of its autocallable books.
“For example, the dynamics in play in 2012, that is, a range-bound Nikkei and the spot-vol correlation turning positive, would be completely captured now. We can see what the dynamics will be two months down the line, and so have better insight into how we should be hedging,” says Kotecha.
Since Asian indexes often exhibit large vol-of-vol regimes, UBS’s quants also built advanced moment-matching techniques into the model to account for explosive market scenarios. The model also considers the correlation impacts often embedded into multi-asset books in order to help the exotics desks avoid over or under hedging.
Calibration technology
For dual-underlying conditional volatility and variance swaps, UBS quants have designed calibration techniques that bolster the management of cross-asset spot-vol, vol-of-vol and skew and smile correlation risks. With the resulting calibration methodology, UBS can better determine the appropriate instruments for hedging autocall flows, says Kotecha.
“The quant team has devised a calibration methodology, which has bolstered the management of correlation risk and helped hedge risk on spot-vol and vol-of-vol,” says Kotecha, who also notes that correlation and volatility hedges gained traction after the February sell-off while dual corridor trades on ESX5 and SPX allowed dealers to reduce vega risk.
In the flow derivatives space covariance and knock-in (KI) correlation swaps are being used to hedge volatility correlations between assets generated by autocall flows.
Otherwise known as geometric dispersion trades, covariance swaps pay investors the mean realised variance between two variables, such as Nikkei-SPX, HSCEI-SPX and HSCEI-SX5E. The trades offer dealers the means to buy back correlation skew with a replicable payoff, and provide funds with both a yield pick-up and the opportunity to sell correlation at a premium.
UBS says correlation and volatility hedges gained traction with clients after the February selloff in global stocks. As volatility returned to the markets, UBS looked for ways to recycle its risk inventory more systematically. Large dual-corridor trades were seen on Eurostoxx 50 versus S&P 500 in order to hedge the dynamics of Korean autocallables. This allowed dealers to reduce their vega risk as Eurostoxx 50 outperformed the S&P 500, and implied volatility on Europe’s blue-chip index began trading below that of the US stock index.
“We have improved our risk management via the application of quantitative analysis and risk transfer through co-variance and KI correlation swaps with institutional clients,” says Kotecha.
Together with the advances made in trade automation, the enhancements in risk transfer have allowed the bank to grow its structured products business. Kotecha says the growth in equity-linked structured products is clearly a product of the improvements that automation and quantitative analysis has brought to the team’s understanding of risk dynamics.
“Overall, we now have more market share with better automation and less operational risk,” he adds. “We have better risk management tools, a much better understanding of spot-vol dynamics in the market, and enhanced hedging techniques.”
Only users who have a paid subscription or are part of a corporate subscription are able to print or copy content.
To access these options, along with all other subscription benefits, please contact info@risk.net or view our subscription options here: http://subscriptions.risk.net/subscribe
You are currently unable to print this content. Please contact info@risk.net to find out more.
You are currently unable to copy this content. Please contact info@risk.net to find out more.
Copyright Infopro Digital Limited. All rights reserved.
As outlined in our terms and conditions, https://www.infopro-digital.com/terms-and-conditions/subscriptions/ (point 2.4), printing is limited to a single copy.
If you would like to purchase additional rights please email info@risk.net
Copyright Infopro Digital Limited. All rights reserved.
You may share this content using our article tools. As outlined in our terms and conditions, https://www.infopro-digital.com/terms-and-conditions/subscriptions/ (clause 2.4), an Authorised User may only make one copy of the materials for their own personal use. You must also comply with the restrictions in clause 2.5.
If you would like to purchase additional rights please email info@risk.net
More on Awards
Clearing house of the year: LCH
Risk Awards 2025: LCH outshines rivals in its commitment to innovation and co-operation with clearing members
Best use of machine learning/AI: CompatibL
CompatibL won Best use of machine learning/AI at the 2025 Risk Markets Technology Awards for its use of LLMs for automated trade entry, redefining speed and reliability in what-if analytics
Markets Technology Awards 2025 winners’ review
Vendors jockeying for position in this year’s MTAs, as banks and regulators take aim at counterparty blind spots
Equity derivatives house of the year: Bank of America
Risk Awards 2025: Bank gains plaudits – and profits – with enhanced product range, including new variants of short-vol structures and equity dispersion
Law firm of the year: Linklaters
Risk Awards 2025: Law firm’s work helped buttress markets for credit derivatives, clearing and digital assets
Derivatives house of the year: UBS
Risk Awards 2025: Mega-merger expected to add $1 billion to markets revenues, via 30 integration projects
Interest rate derivatives house of the year: JP Morgan
Risk Awards 2025: Steepener hedges and Spire novations helped clients navigate shifting rates regime
Currency derivatives house of the year: UBS
Risk Awards 2025: Access to wealth management client base helped Swiss bank to recycle volatility and provide accurate pricing for a range of FX structures