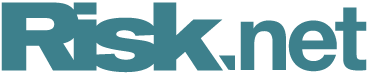
This article was paid for by a contributing third party.More Information.

A call to arms – How machine intelligence can help banks beat financial crime
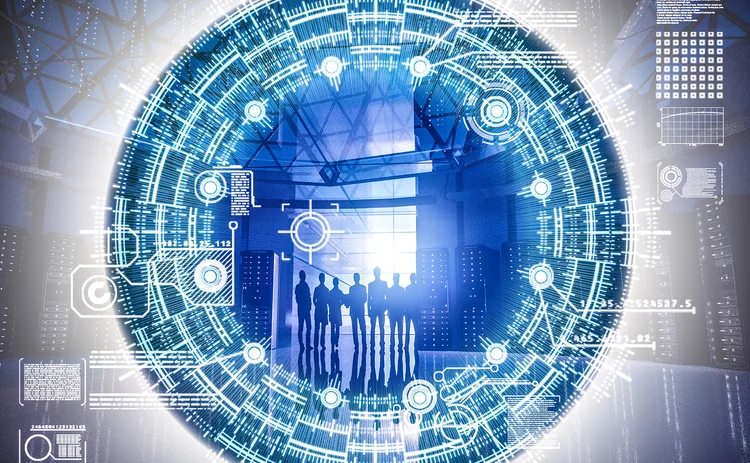
The revolution in artificial intelligence (AI) promises new leads in banks’ fight against dirty money. Alexander Campbell of Risk.net hosted a live online forum, in association with NICE Actimize, to investigate the applications of this emergent technology
The panel
Jim Arndts, Director, Enterprise financial crimes compliance strategy, transformation and governance, US Bank
Michael Schidlow, Head of financial crime compliance and emerging risk audit development, HSBC
Ted Sausen, Director and anti‑money laundering subject matter expert, NICE Actimize
McHenry Kane, Senior vice‑president and director of anti-money laundering, New York Community Bank
Moderator: Alexander Campbell, Editor, Risk.net
What technology evangelists call the fourth industrial revolution is already well under way. Barely a week passes without a new prototype or roll-out of AI, robotic process automation (RPA) or machine learning. For banks, defeating financial crime is a crucial application of this emergent technology.
Its deployment could be a game‑changer for financial institutions. With that in mind, Risk.net convened a panel of financial crime risk, compliance and technology experts from NICE Actimize, HSBC, US Bank and New York Community Bank to discuss the risks and benefits of automation.
Where to start?
The machine-intelligence revolution promises to be fruitful on multiple fronts in the war against dirty money – from screening and prevention to post‑event analytics. Singling out the opportunities and prioritising them over the next few years is a significant challenge for risk, audit and compliance professionals.
Improving the value of alerts and access to relevant data quickly is the top priority for Jim Arndts, director of enterprise financial crimes compliance strategy, transformation and governance at US Bank. Applying AI in this way has great potential to free up human resources and increase productivity.

“Improving our alert production and alert management processes is the biggest opportunity we face in our industry,” said Arndts. “The industry is familiar with producing a large number of alerts: many are high value, many are lower value. With the prototyping and proof-of-concept work we’re doing, we can have big gains towards eliminating the items that we don’t need, looking at how they’re generated, focusing on the higher‑value alerts and getting to the higher‑value cases.”
Michael Schidlow, HSBC’s head of financial crime compliance and emerging risk audit development, highlighted two separate directions from which the bank’s systems and processes could reap the biggest benefits of AI in countering criminals.
“One is certainly in the investigation space,” he said. “Absolutely, we should be able to cut the number of false positives and less useful alerts. At the same time as we’re automating, we should also be able to leverage relevant information more strategically on an automated basis that, until now, we’ve only been using for tactical purposes.”
Schidlow suggested that, by using data much closer to real time, banks could determine whether an apparently innocuous customer is really a hidden beneficial owner behind a seemingly unrelated commercial banking account, which in turn has an undisclosed repeated pattern of suspicious transactions with another customer.
“That level of robust behind‑the‑scenes data culling, which automation makes much more possible than on a manual basis, would be tremendously valuable,” said Schidlow.
He highlighted the risk‑appetite benefits for banks facing a backlog of manual processes: “Do we have to scale back on some of our risk because we’re too busy chasing more and more cases? I think we’re going to start chasing the real cases, and a lot of the effort spent on those false positives can be focused more on the investigations of the true hits that we’re getting.”
Further avenues through which to explore machine learning were added to the mix by Ted Sausen, director and anti‑money laundering (AML) subject matter expert at NICE Actimize. One of these is to look not just at the false-positive rate – though it is high – but also at the false negatives generated by the current generation of legacy systems and manual processes still prevalent within many banks.
“We don’t hear a lot of people talking about the false negatives, or doing below‑the‑line testing,” said Sausen. “That’s hard to deal with and an area I think we’re going to see more of a push in. How do you identify what you don’t know, or what you don’t see? Machine learning is going to benefit anomaly detection and help us find those things that we’ve only just started to be aware of.”
Once banks get a better grasp of those questions, Sausen senses a further opportunity for greater automation within the more refined due-diligence processes.
“We can start to look at all the data attributes of a certain client and compare that against other population bases, and see if that is going to be a riskier client to take on, above and beyond your normal corporate due-diligence activity,” he said. “We’ve got client risk ratings, but there is other data we can collect to make decision‑making more robust before we even take a client into the financial institution.”
Shifting assumptions
The journey towards greater automation needs charting. McHenry Kane, senior vice‑president and director of AML at New York Community Bank, noted the importance of clarity about the differences between implementing RPA and introducing machine learning.
“Machine learning is a bit like a self‑driving car, where we can see what we ultimately want, and there are a lot of different components involved,” said Kane. “With RPA, essentially you program software to do certain things. That is the first step – like adaptive cruise control – to making the throughput for investigators and analysts far more efficient, grabbing the information you need automatically and helping to define it, to go after more specific information.

“When we move onto machine learning, we’re talking about systems that are changing their thresholds and rules on the basis of experience. Those are also defined algorithms in some respects, but they’re self‑correcting and they’re changing their assumptions and their thresholds,” Kane continued.
Kane is interested in what the higher end of the machine-learning revolution could bring to improving model validation, but he forecasts problems on the road towards automising AML work as the industry knows it.
“Model validation requires that any change to the rules, the thresholds and the assumptions underlying the rules requires a lot of consideration and documentation. I think that’s where we’re going to see a lot of development with more machine learning,” said Kane.
Regulators would likely want to keep close tabs on such developments. “In the US, regulators have exhibited willingness to go along with this to a certain degree, but I’m also aware that, like self-driving cars, no regulator wants to end up with an accident on their hands,” Kane added.
Trusting machine learning to continually change underlying assumptions and adjust fact patterns for suspicious activity reports (SARs) could be a cause for concern for authorities if AI is deemed to be a ‘black-box’ problem and a barrier to transparency.
Sausen said he has spoken with several regulators to gauge the levels of their approval of machine learning playing a progressively greater role. “The majority of regulators suggest that, as long as the financial institution is comfortable with the model or machine they have put in place and can explain why they’re comfortable with it, that makes them much more comfortable,” he said. “They’ll have to become more accepting of it, but I think that’s going to be a partnership.”
The financial sector will also need to be comfortable with its risks within the relationship. “It’s certainly giving the industry more buy‑in,” said Kane. “The question is: do you always know where the underlying assumptions and rule changes and thresholds are adapting to? Is it above- or below-the-line testing assumptions that are being changed on the fly; and, therefore, are they fairly tested before adjustments are made?”
Some banks are already experimenting in parallel with their existing systems, Kane suggested, producing positive results. “There’s always that nagging question of what’s excluded and whether you’ve made a mistake,” he said. “That’s partly down to the way the rules are written; there’s a penalty every time you miss an SAR, but there’s not necessarily a penalty if you overinvestigate.”
The large volumes of data being used and produced by analytics – growing increasingly larger with the scale that automation can bring to analysis – should help provide the evidence to document and validate changes to models.
“That data helps you manage this process, be able to point to that particular point in the audit trail where something was changed, and demonstrate why it was changed,” said Arndts. “The industry is producing data that would help tell your story [better] than we have been able to in the past.”
Sausen noted that models, while reflecting “best judgement” and heavily based on historical data, should also be open to continual change. In that sense, the shifting assumptions of AI‑driven data analysis should help with that evolution and provide more robust data as evidence to support change.
“Your business activity changes and criminal activity changes,” he said. “Machine learning will help you validate that your model is doing what it should be doing, or tell you that your model has problems as new activities are introduced. Keeping it fresh will help us in the future.”
Defining what is classed as a model among a financial institution’s myriad systems and processes could become a more important question once many of them are powered by AI, Schidlow emphasised.
“It’s important from an audit perspective because it brings about a lot of governance‑related issues to clearly indicate what systems, services and processes are in fact models that are AI‑enabled or AI‑enhanced, and document those as models,” he said.
Regulators will be acutely interested in the quality of data fuelling the machines, Sausen warned. More does not necessarily mean better, particularly if it lacks proper screening to vouch for its validity or accuracy.
“The one thing we have to watch out for is not to just go out there and grab all the data we can; we also need to review the data,” said Sausen. “I met with a regulator last week, and one of the questions they asked was how you prove you went out there and looked at all that data. One aspect is proving we did something with it and the other is, if you don’t do anything with it, what if there is something glaring there that’s important but you missed it? There’s a lot more data out there, and we can be more efficient, but we don’t want to overdo it.”
Made to measure
According to a poll conducted during the discussion, around two‑thirds of online listeners to the forum said they were either already using or considering using AI to help predict complexity in the risks they face. The remainder had yet to engage.
The panel was unsurprised by this. Multinationals and smaller institutions may roll out automation at different speeds or levels with different capabilities, and for tactical or strategic purposes, Schidlow suggested.

“I dislike using the phrase ‘one size doesn’t fit all’, but in this case it’s true,” he said. “One bank may be dabbling with teradata mining, and they may have just started talking about how to leverage analytics, and what they can be used for.
“A peer institution may have tactical and strategic teams in place, and then another institution of smaller size or level of complexity may not have any of this because the nature of their services doesn’t necessitate it.”
Arndts agrees that institutions will follow different routes for RPA and machine learning. “We’ve learned some lessons from the early 2000s when people started slamming in AML systems without being exactly sure what they were doing. Let’s prove the concepts before everyone dives into the deep end. You’re not going to have a simple adoption rate, just as you’re not going to have a person or a team within every bank that is skilled, from an AML perspective, to develop this,” he said.
Without the right expertise and training in place, AI could prove a wasteful experiment. “Even the greatest and most sophisticated machine is just a shiny paperweight if nobody knows how to use it correctly,” said Kane.
Some firms will therefore want to bring in more outside expertise than others, depending on their own circumstances, Schidlow noted. “Not every bank will need to be as tech‑savvy to necessitate developing in‑house AI. There are opportunities to bring in outside expertise for particular projects on a needs‑based assessment. You simply don’t need to develop your own Harrier jump jet as a sophisticated piece of equipment, depending on the task,” he said.
Moving too fast can carry its own risks. Failure to understand or document changes designed to bring efficiencies could have the opposite effect by leading to trouble with audits as well as with regulators, creating additional work that might have been prevented.
“It’s dangerous to jump in all at once,” said Sausen. “This is a building process in proving it out, and you have to make sure you know what you’re doing before you go all in.”
Change management is shifting fundamentally, Schidlow argued, from a waterfall to more agile processes. He noted that internal audits would be rightly concerned by sudden or poorly planned changes.
“Audits would be rightly concerned with the change management process,” said Schidlow. “You don’t want to see a wholesale unplug, reboot and start‑up. Like any other model, regardless of its sophistication, you want each deployment or iterative change to be tested prior to deployment. You want to ensure it will deliver the output that you anticipate with strong governance, and by a measured and well‑documented change management process.”
No-brainer
Costs related to people represent the number one expense within Kane’s department. Having a supercomputer take seconds to perform what would take a team of humans days or weeks is an obvious boon of handing over onerous tasks to RPA. It should free up staff from monotonous work, reducing the likelihood of the boring nature of such tasks leading to avoidable errors.
“Nobody wants to do work that seems remedial and soul‑sucking,” said Kane. “If you’re looking at false positive after false positive there’s a risk that, when you come across something you ought to investigate further, you dismiss it because that’s what you’re habituated to do.”

Many firms have resources dedicated to the onerous tasks of examining data quality, Schidlow noted, focusing on ensuring that data-quality dimensions conform, are consistent, appropriate, accurate and complete. With technology, these resources can be better deployed.
“Let’s say, as an example, we’ve done a batch analysis of our trade receivables group and, of 1,000 transactions, about 450 had data completeness issues because they all had the same or a similar missing field. By using automation rather than manual processes to know that these transactions did not meet this data-quality dimension requirement, you’d be able to identify the root cause,” said Schidlow.
“If you’ve expedited what would have been a 30-, 60- or 90‑day audit in a matter of moments, that allows you to triage where a specialised team of data auditors can focus. That’s not to say those issues are minute or irrelevant, but there are bigger fish to fry. By automating those data-completeness processes, you’ve freed up human resources to work on something with even more relevance, significance or urgency.”
Sending in a robot to automatically file or structure an SAR or to undertake the data-quality dimensions of auditing frees up people for more profound work. That can lead not just to greater organisational efficiency for the bank, but also to higher levels of job satisfaction for its employees.
“I want people free to use their minds as much as possible,” said Kane. “We work in an interesting industry. People deal with sexy‑sounding risks of crime – narcotics, sanctions, money laundering and terrorist financing. While those things are terrible, it is meaningful for people in the banking world to go out and say ‘We’re finding these things and we’re making a difference.’”
Watch the full webinar proceedings
The panellists were speaking in a personal capacity. The views expressed by the panel do not necessarily reflect or represent the views of their respective institutions.
Sponsored content
Copyright Infopro Digital Limited. All rights reserved.
As outlined in our terms and conditions, https://www.infopro-digital.com/terms-and-conditions/subscriptions/ (point 2.4), printing is limited to a single copy.
If you would like to purchase additional rights please email info@risk.net
Copyright Infopro Digital Limited. All rights reserved.
You may share this content using our article tools. As outlined in our terms and conditions, https://www.infopro-digital.com/terms-and-conditions/subscriptions/ (clause 2.4), an Authorised User may only make one copy of the materials for their own personal use. You must also comply with the restrictions in clause 2.5.
If you would like to purchase additional rights please email info@risk.net