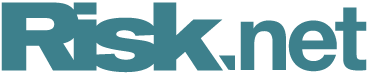
Rogue traders versus value-at-risk and expected shortfall
VAR and ES are ineffective to deter rogue trading
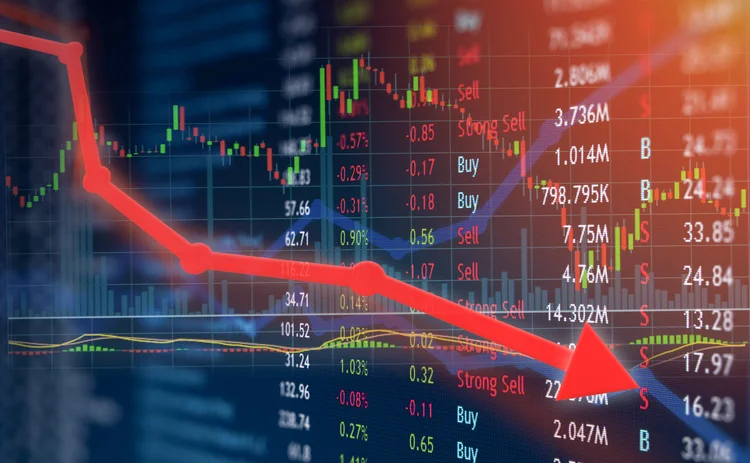
John Armstrong and Damiano Brigo show that, in a Black-Scholes market, value-at-risk and expected shortfall are irrelevant in limiting traders’ excessive tail risk-seeking behaviour, as modelled by Kahneman and Tversky’s S-shaped utility. The authors argue that to have effective constraints one can introduce a risk limit based on a second, but concave, utility function
In this article, we aim to analyse how classic risk measures such as value-at-risk and expected shortfall (ES) fare in limiting excessive tail risk-seeking behaviour in market players. We will model excessive tail risk-seeking behaviour using Kahneman and Tversky’s S-shaped utility (Kahneman & Tversky 1979). For example, S-shaped utility is naturally suited to modelling the behaviour of a trader who cares only about their pay packet and not about the overall loss of the bank, once the latter defaults. A traditional concave utility function would fail to reflect the limited liability of traders.
We point out, via a payoff optimisation approach, that a trader may optimise expected S-shaped utility in the presence of budget constraints regardless of VAR or ES constraints, in the sense that the optimal expected S-shaped utility without VAR or ES constraints will be exactly the same as that with VAR or ES. VAR and ES are thus ineffective in limiting rogue traders. This is particularly important when we consider ES was officially endorsed and suggested as a risk measure by the Basel Committee in 2012 and 2013, partly for its ‘coherent risk measure’ properties (Artzner et al 1999; Acerbi & Tasche 2002).
We then hint at a solution to the problem: it will be enough to introduce a risk limit based on a second utility function, a traditional concave utility, for the expected concave utility constraint to be effective in curbing excessive tail risk-seeking behaviour. Indeed, for this concave utility-based risk constraint the optimum will not be the same as in the case without constraint. We do not prove the result in this article, instead referring the reader to Armstrong & Brigo (2017). The proof given there relies on the ideas used by Hardy and Littlewood to prove their inequality on symmetric decreasing rearrangements (Hardy et al 1952).
In this article, we limit our analysis mostly to the Black-Scholes-Merton case (Black & Scholes 1973; Merton 1973), since this is a benchmark model for derivatives valuation and allows us to state our case without excessive mathematical infrastructure and within a familiar setting. However, our result is much more general, and the general theory relies on a result that is similar to the theory of rearrangements behind the Hardy-Littlewood inequality (see Armstrong & Brigo 2017). This is not needed or used here, however, as in this article we use more direct techniques that allow us to avoid such complicated machinery. The result is thus more immediate. The general result follows the same line of research as earlier contributions to behavioural finance, prospect theory and portfolio choice, which we will now review.
Expected utility maximisation under risk measure and budget constraints was considered in Basak & Shapiro (2001), but only under standard utility assumptions, and not an S-shaped utility in particular. In that paper, it is shown that a market player forced by a VAR constraint to reduce portfolio losses in some states would finance these reduced losses by increasing portfolio losses in costly states where the terminal state price density is large. As such states already have the lowest terminal portfolio value for the unconstrained problem, the VAR constraint ends up fattening the left tail of the terminal portfolio distribution. This leads to an increased probability of extreme losses. In Cuoco et al (2008), it is shown that VAR constraints play a better role when, as is done in practice, the portfolio VAR is re-evaluated dynamically by incorporating available conditioning information. Again, this is done under standard utility, and S-shaped utility is not considered.
Prospect theory has been studied in relation to risk measures and portfolio choice in a series of papers (Jin & Zhou 2008; Zhou 2010; He & Zhou 2011; He et al 2015). These tackle problems similar but not equivalent to the problem we consider here. Jin & Zhou (2008); He & Zhou (2011), for example, do not study risk constraint in optimising S-shaped utilities (with distortions), while in He et al (2015) the problem closest to our own is a problem in which expected returns are optimised but expected utility is not. Still, these papers find connections with rearrangements, use law-invariant portfolio optimisation and employ techniques and proofs that deal with and solve a wide range of behavioural finance problems that are similar to those in this article and in our more general paper (Armstrong & Brigo 2017).
The Black-Scholes market
We introduce briefly the Black-Scholes model (Black & Scholes 1973; Merton 1973) for a market with a single risky asset and a bank account. We consider a probability space with a right-continuous filtration . In the given economy, two securities are traded continuously from time until time . The first security, the cash account or bank account, is locally risk-free and its price evolves according to:
(1) |
where is a non-negative number. Usually, the risk-free rate is an adapted process but, for simplicity, in this context we assume it is a positive deterministic constant.
As for the second security, given the -Wiener process , consider the following stochastic differential equation for the price of such a security, typically a stock:
(2) |
with initial condition , and where and are positive constants. Equation (2) has a unique (strong) solution, given by:
(3) |
We can write the probability law of easily by recalling that is normally distributed with mean zero and variance . Let us call the cumulative distribution function (CDF) of under the measure . We have a lognormal distribution for :
where is the CDF of the standard normal distribution.
Further, in the basic Black-Scholes model there are no transaction costs; short selling is allowed without penalty or restrictions, and borrowing and lending occur at the risk-free rate , with no credit or default risk or funding costs.
The full development of valuation in the presence of counterparty credit risk, liquidity risk, funding and capital costs has been addressed in the literature in recent years: see, for example, Brigo et al (2017), a work that tries to stay as close as possible to Black-Scholes while including credit, repurchase agreement and funding effects.
Hence, even if the Black-Scholes model neglects important aspects of valuation, we work under its assumptions because we are interested in S-shaped utility and ES limits in benchmark models. We expect our results to hold in extensions of the basic model, particularly in cases where the resulting valuation approach is very similar to the basic Black-Scholes setting, as in Brigo et al (2017).
Simple claims and market price of risk
We consider a simple contingent claim. This is a contract guaranteeing a payoff of the form payable at maturity . If we assume the market is arbitrage-free and complete, the unique no-arbitrage price of our simple claim at time is the expected value:
where the expected value is taken under a probability measure , the risk-neutral measure, equivalent to . Under this measure :
where is a standard Brownian motion under . It is immediately obvious the ratio is a -martingale, and this is why is sometimes referred to as the martingale measure. We can write the Radon-Nikodym derivative connecting the two equivalent measures as:
In the particular version of the Black-Scholes setting we are working with, this formula can also be written as:
The Radon-Nikodym derivative becomes an explicit function of the underlying . This makes both the Radon-Nikodym derivative and the payoff functions of the same variable and renders the analysis of their interaction explicit.
The constant is called the market price of risk or a particular version of the Sharpe ratio. We have:
(4) |
We assume , ie, . This means a trader will be interested in investing in stock , since its expected return will exceed the risk-free rate in the market.
Standardisation to a uniform risky asset
In our subsequent utility analysis, we will rescale the risky asset’s probability distribution to be uniform. In the Black-Scholes context, this means that instead of expressing simple claims as functions of , we will express them as functions of . Indeed, we know that has a standard uniform distribution. This allows us to write the price of the claim as:
where:
Given our expressions above for , and our assumption on , it is clear is decreasing. Further, a simple limit calculation based on the fact is essentially a power to the shows:
S-shaped utility and tail risk-seeking behaviour
It is observed in Kahneman & Tversky (1979) that individuals appear to have preferences governed by an S-shaped utility function, . This is increasing, strictly convex on the left, strictly concave on the right, non-differentiable at the origin and asymmetrical: negative events are considered worse than positive events, which are considered good.
A typical S-shaped utility function is shown in figure 1.
Figure 1: Typical example of an S-shaped utility curve.
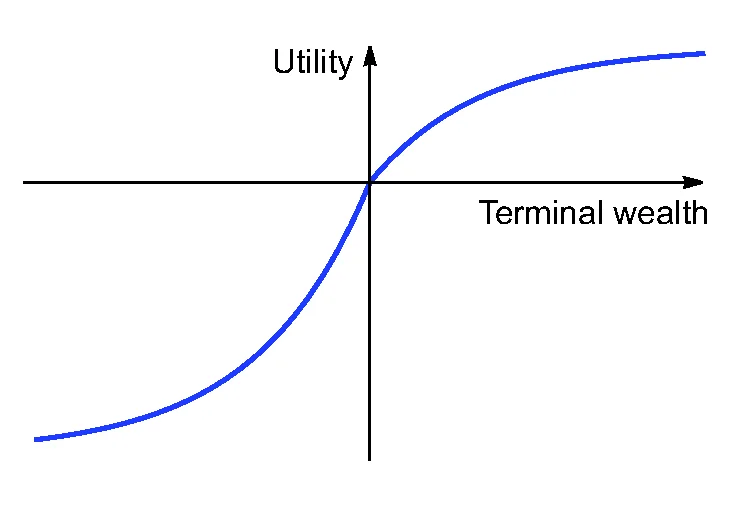
Whether the cause of S-shaped utility functions is the irrationality or limited liability of a market player, there is certainly good evidence they are a useful tool for modelling real-world behaviour. A regulator or risk manager could certainly consider them as a possibility.
As not all of the characteristics of S-shaped utility functions are important to us, we adopt a slightly more general definition. Suppose we have a candidate utility function as an increasing function , which represents the utility of holding the wealth . Inspired by the definition of S-shaped utility in Kahneman & Tversky (1979), we assume the following estimates hold:
for some positive constants . We call such a utility function risk seeking in the left tail and risk averse in the right tail.
In this work, we finally define formally an S-shaped utility curve as an increasing function that is negative for , positive for , concave for , risk seeking in the left tail and risk averse in the right tail.
Utility maximisation under budget and expected shortfall constraints
We are now interested in a utility maximisation problem in the Black-Scholes market. The trader or investor wishes to optimise over all simple claims to find the claim that gives them the maximum utility:
(5) |
under constraints:
(6) | ||||||
(7) |
where denotes the expected shortfall of over the horizon at confidence level . In this formulation of the problem, we implicitly assume the additional constraint that the expectation and ES are both well defined and finite. We can reformulate the above problem after uniform rescaling :
(8) |
under constraints:
(9) | ||||||
(10) |
The ES representation in this last formulation comes from Acerbi & Tasche (2002), (3.3). Again, we implicitly assume the latter two integrals exist and are finite.
We will now show that, under the assumption (recall that depends on ), the ES constraint is not relevant in that the maximum attained under the constraint is the unconstrained . Thus, for S-shaped utility functions that are risk seeking in the tail, as we expect from traders, the ES constraint is ineffective in curbing excessive risk taking.
First, we could consider all constant functions and optimise on those. If we denote by the function constantly equal to , the optimisation problem is:
(under constraints) | (11) | |||||
(budget) | (12) | |||||
(ES constraint) | (13) |
So, we know that if is the optimal utility in the full problem, we have as our lower bound the result for the ‘constant ’ problem:
As it turns out, it is enough to move to the next simplest possible function , namely a two-step piecewise constant function, to obtain a much sharper result.
Theorem 1.
(Irrelevance of ES constraint in S-shaped utility maximisation in a Black-Scholes market) Consider a Black-Scholes market in which the bank account price and the risky asset (stock) price follow the differential equations:
(14) |
where is a standard Brownian motion under the measure in a probability space , and is a positive deterministic constant modelling the volatility. Assume , and assume we are given an S-shaped utility function that is risk seeking in the tail; namely, there are constants and such that:
(15) |
Let be the supremum over claims defined by:
under constraints:
Then .
Proof.
We will use non-decreasing piecewise constant functions taking only two values. Given three constants , with and , define:
We will omit the arguments and for brevity in this proof. Consider the expected utility for this function:
(16) |
Let us now write the two constraints in the optimisation problem: the budget constraint and the ES constraint. The budget constraint reads:
or | |||
The ES constraint reads:
or | |||
Putting both constraints together, we obtain:
In this constrained interval, we now pick a special point, the initial point, thus assuming the ES constraint is met as an equality:
We need to check this constraint is consistent with our assumption that . This holds as long as is chosen to be sufficiently large and positive, namely . We denote the related function as:
and the related expected utility (16) specialises to:
(17) |
The budget constraint for becomes:
(18) |
We can obtain a lower limit to the right-hand side of the budget constraint (18) by noting:
If we now impose for a sufficiently large positive constant , then the right-hand side becomes negative and we can estimate it further with:
where the first estimate follows from being decreasing, so if we take for a positive , we conclude:
The range for will depend on . However, we can estimate the left-hand side of the budget constraint (18) from above by noting:
and this can be made sufficiently negative, say less than , by choosing a sufficiently large :
where we are further assuming . Thus, we may write:
(19) |
as long as we make sure the central inequality:
(20) |
holds. Since the denominator tends to as tends to , it suffices, again, to choose a small enough .
We conclude that both the budget and ES constraints are met if we require and satisfy:
(21) |
with the lower bound satisfying:
with constants large enough and small enough, and with ranges determined by the other constants . We assume these hold from now on.
We may now invoke inequality (15) for the utility function to deduce:
as long as with chosen to be sufficiently small. We can use this to estimate the expected utility in (17) as follows:
Given that , we can conclude that for the first term on the right-hand side tends to zero, and we are left with:
with the budget and ES constraints satisfied implicitly via (21) when taking the limit.
It follows that our general utility optimisation problem has the solution when optimising in the subclass of admissible functions . It also follows that the optimum on a larger class will yield an optimal expected utility larger than for all possible . However, it is obvious the optimal expected utility will be bounded from above by the supremum of the utility function. This then proves the claim that the optimal expected utility will be equal to the supremum of the utility function.
Figure 2: Payoff used in the proof of the main theorem.
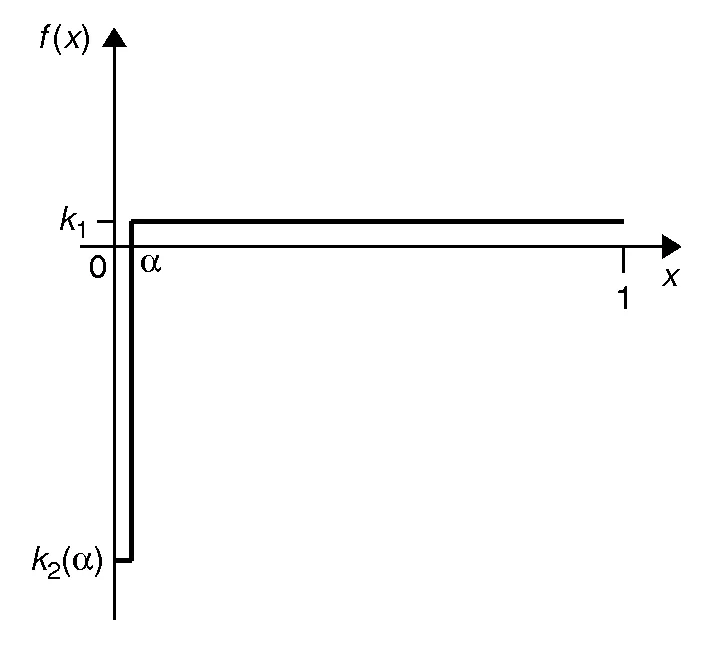
It may be interesting to check what the limiting function looks like for small . Recall that:
Fixing , for very small the first constant becomes negative and very large in absolute value, but on a very small interval ; the second constant, however, is equal to on a large interval . We thus have a digital option with an extremely negative constant payoff in a small range of the rescaled uniform underlying, , and with a much smaller positive payoff in the remaining range . This is illustrated in figure 2.
An effective risk constraint based on a second concave utility
The focus of this short article is the negative result above. However, we would like to hint at a possible solution for the ineffectiveness of the VAR and ES constraints; this is developed fully in Armstrong & Brigo (2017).
Above, we have seen a result related to the following fact: an investor with S-shaped utility function , with plus a budget constraint, and who is subject only to ES (or VAR) constraints for risk can find a sequence of portfolios satisfying these constraints that has an expected -utility tending to infinity. This implies VAR or ES constraints cannot limit excessive tail risk-seeking behaviour.
If the risk constraint is based instead on a second utility function of the type:11We use the term ‘utility function’ for somewhat informally here, as what is important for our result is the functional form of the limit set and not whether it has been derived from any specific individual’s utility function. One might loosely think of as the regulator’s or risk manager’s utility function, although in practice the regulator or risk manager should choose any risk limits to reflect the risk preferences of whoever bears the risk. We have not attempted to consider how they should do this. We are simply assuming one of the limits set has the given functional form.
with , where the constraint requires the payoff to be in the set:
for a negative loss level , then any sequence of portfolios whose expected S-shaped utility tends to will have expected tending to , and thus will not be acceptable for the risk constraints. In this sense, a classic concave utility adopted by the risk manager or regulator can be used more effectively than VAR or ES in limiting excessive tail risk-seeking behaviour in the presence of limited liability/S-shaped utility investors.
John Armstrong is a senior lecturer at King’s College London. Professor Damiano Brigo heads the mathematical finance group at Imperial College London and is part of the stochastic analysis group.
Email: john.1.armstrong@kcl.ac.uk,
damiano.brigo@imperial.ac.uk.
References
- Acerbi C and D Tasche, 2002
On the coherence of expected shortfall
Journal of Banking and Finance 26, pages 1487–1503 - Armstrong J and D Brigo, 2017
Optimizing S-shaped utility and implications for risk management
Report 1711.00443, arxiv.org - Artzner P, F Delbaen, J-M Eber and D Heath, 1999
Coherent measures of risk
Mathematical Finance 9(3), pages 203–228 - Basak S and A Shapiro, 2001
Value-at-risk based risk management: optimal policies and asset prices
Review of Financial Studies 14, pages 371–405 - Black F and M Scholes, 1973
The pricing of options and corporate liabilities
Journal of Political Economy 81(3), pages 637–654 - Brigo D, C Buescu and M Rutkowski, 2017
Funding, repo and credit inclusive valuation as modified option pricing
Operations Research Letters 45(6), pages 665–670 - Cuoco D, H He and S Issaenko, 2008
Optimal dynamic trading strategies with risk limits
Operations Research 56, pages 358–368 - Hardy GH, JE Littlewood and G Pólya, 1952
Inequalities
Cambridge University Press - He X and X Zhou, 2011
Portfolio choice via quantiles
Mathematical Finance 21, pages 203–231 - He X, X Jin and X Zhou, 2015
Dynamic portfolio choice when risk is measured by weighted VaR
Mathematics of Operations Research 40, pages 773–796 - Jin H and X Zhou, 2008
Behavioral portfolio selection in continuous time
Mathematical Finance 18, pages 385–426 - Kahneman D and A Tversky, 1979
Prospect theory: an analysis of decision under risk
Econometrica: Journal of the Econometric Society 47(2), pages 263–292 - Merton RC, 1973
Theory of rational option pricing
Bell Journal of Economics and Management Science 4(1), pages 141–183 - Zhou X, 2010
Mathematicalising behavioural finance
Proceedings of the International Congress of Mathematicians, Hyderabad, India
Only users who have a paid subscription or are part of a corporate subscription are able to print or copy content.
To access these options, along with all other subscription benefits, please contact info@risk.net or view our subscription options here: http://subscriptions.risk.net/subscribe
You are currently unable to print this content. Please contact info@risk.net to find out more.
You are currently unable to copy this content. Please contact info@risk.net to find out more.
Copyright Infopro Digital Limited. All rights reserved.
As outlined in our terms and conditions, https://www.infopro-digital.com/terms-and-conditions/subscriptions/ (point 2.4), printing is limited to a single copy.
If you would like to purchase additional rights please email info@risk.net
Copyright Infopro Digital Limited. All rights reserved.
You may share this content using our article tools. As outlined in our terms and conditions, https://www.infopro-digital.com/terms-and-conditions/subscriptions/ (clause 2.4), an Authorised User may only make one copy of the materials for their own personal use. You must also comply with the restrictions in clause 2.5.
If you would like to purchase additional rights please email info@risk.net
More on Risk management
BofA sets its sights on US synthetic risk transfer market
New trading initiative has already notched at least three transactions
Op risk data: At Trafigura, a $1 billion miss in Mongolia
Also: Insurance cartels, Santander settlement and TSB’s “woeful” customer treatment. Data by ORX News
Cyber risk can be modelled like credit risk, says Richmond Fed
US supervisors may begin to use historical datasets to assess risk at banks and system-wide
The changing shape of risk
S&P Global Market Intelligence’s head of credit and risk solutions reveals how firms are adjusting their strategies and capabilities to embrace a more holistic view of risk
To liquidity and beyond: new funding strategies for UK pensions and insurance
Prompted by policy shifts and macro events, pension funds and insurance firms are seeking alternative solutions around funding and liquidity
More cleared repo sponsors join Eurex ahead of cross-margining
End of TLTROs for banks and pension fund search for liquidity management tools drives uptake
Reimagining model risk management: new tools and approaches for a new era
A collaborative report by Chartis and Evalueserve on how the use of automation can combat the growing complexity of managing model risk due to regulation and market volatility
What Goldman’s appeal victory means for Fed stress tests
Decision could embolden more banks to appeal, analysts say. But others believe result is one-off