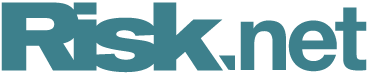
Neural network learns ‘universal model’ for stock-price moves
Relationships between order flow and price “are stable through time and across stocks and sectors”

Academics have used machine learning to create a “universal” model for predicting short-term stock-price changes – disproving common assumptions among dealers, hedge funds and high-frequency traders about how such models should be built.
In a recent study, Rama Cont, a professor at Imperial College London, and Justin Sirignano, assistant professor at the University of Illinois at Urbana-Champaign, used a neural network trained on two years of intraday data from Nasdaq’s limit order book to
Only users who have a paid subscription or are part of a corporate subscription are able to print or copy content.
To access these options, along with all other subscription benefits, please contact info@risk.net or view our subscription options here: http://subscriptions.risk.net/subscribe
You are currently unable to print this content. Please contact info@risk.net to find out more.
You are currently unable to copy this content. Please contact info@risk.net to find out more.
Copyright Infopro Digital Limited. All rights reserved.
As outlined in our terms and conditions, https://www.infopro-digital.com/terms-and-conditions/subscriptions/ (point 2.4), printing is limited to a single copy.
If you would like to purchase additional rights please email info@risk.net
Copyright Infopro Digital Limited. All rights reserved.
You may share this content using our article tools. As outlined in our terms and conditions, https://www.infopro-digital.com/terms-and-conditions/subscriptions/ (clause 2.4), an Authorised User may only make one copy of the materials for their own personal use. You must also comply with the restrictions in clause 2.5.
If you would like to purchase additional rights please email info@risk.net
More on Asset management
Execs can game sentiment engines, but can they fool LLMs?
Quants are firing up large language models to cut through corporate blather
Pension schemes prep facilities to ‘repo’ fund units
Schroders, State Street and Cardano plan new way to shore up pension portfolios against repeat of 2022 gilt crisis
Fears of runaway risk on offshore reinsurance
Life insurers catch the eye of UK regulator for pension buyout financing trick
Hot topic: SEC climate disclosure rule divides industry
Proposal likely to flounder on First Amendment concerns, lawyers believe
‘Brace, brace’: quants say soft landing is unlikely
Investors should prepare for sticky inflation and volatile asset prices as central banks grapple with turning rates cycle
Trend following struggles to return to vogue
Macro outlook for trend appears to be favourable, but 2023’s performance flop gives would-be investors pause for thought
Start-up bond platform OpenYield prepares to launch
Start-up aims to give retail brokers the same electronic liquidity used by the professionals
Can machine learning help predict recessions? Not really
Artificial intelligence models stumble on noisy data and lack of interpretability