
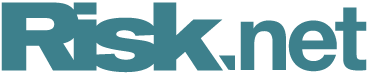
Model risk managers eye benefits of machine learning
Banks are straining to comply with regulator-drafted guidelines introduced to prevent banks suffering losses from decisions based on poorly crafted models. This burden is pushing some firms to explore opportunities afforded by machine learning technologies – though many have reservations
Need to know
- Regulatory initiatives in the US, European Union and UK have turned the spotlight on to banks’ model risk management processes.
- The resulting increased workload on model risk managers is sparking interest in automated processes to help alleviate the burden of certain tasks, such as data cleansing and model validation.
- “If machine learning can help develop a heat map to show where managers should be placing their attention and what models need to be refined, that would help focus their efforts,” says Ed Young at Moody’s Analytics.
- However, consultants and dealers say supervisors are suspicious of the use of ‘black box’ algorithms whose workings banks cannot clearly explain.
- Banks may also struggle to graft machine learning technologies on to legacy systems.
It’s tough being a model risk manager these days. In the US, global investment banks and domestic lenders alike continue to grapple with prudential guidance on model risk management, known as SR 11-7. Meanwhile, their European counterparts recently began welcoming onsite inspectors under the European Central Bank’s Targeted Review of Internal Models (Trim) programme.
UK model teams also have their work cut out. At the end of March, the Bank of England issued a letter to British banks and building societies outlining the model management principles they expect their charges to adhere to.
These regulatory initiatives (see box: New model army) aim to nix the threats posed by unruly models by regimenting the model validation process within banks. But many claim expectations of the model risk management function are outpacing banks’ ability to adapt – with potentially dire consequences.
Floundering amid the wave of new duties assigned to them, model risk managers are understandably seeking a life preserver – and some think they’ve spotted one. Dealers are increasingly exploring the possibilities offered by machine learning (ML) algorithms that can make sense of large, unstructured datasets and police the outputs of primary models.
“I am a big supporter of the use of ML and computational intelligence in model risk management, not only for the development of model benchmarks but also to facilitate the validation process itself,” says Lourenco Miranda, head of model risk management for Americas at Societe Generale in New York. “Humans would never be replaced for the more complex decisions in model risk, but by training a machine to process repetitive parts of validation we can focus our attention on the higher and more complex models responsible for the biggest exposures. It is a great increase in efficacy of the model risk management process.”
Others are less taken by the promise of ML, however: “The short answer is we are not there yet,” says the head of model risk at an international bank. “I think it’s definitely an area to be looked into in the future. But from a practical point of view the risk management platforms of the banks are very heavy. It’s very difficult to change them.”
He’s not alone in his reservations: academics have also warned that ML should not be seen as a silver bullet. Yet so long as the regulatory focus on model risk shows no signs of abating, it seems likely managers will continue to seek new technologies to make their lives easier.
“Anything that could improve the data processing and data cleaning processes would be good, because to tell the truth a good deal of our validation work is on data issues. What I had in mind was to look at whether these solutions can be used in our model environment to replace and automate data treatment and to replace human intervention,” says the head of model validation at a regional European bank.
Reducing the spade work
With resource-strapped model validation teams overloaded, and their in-trays filling up, many are enthused by the potential for machine learning to smooth those parts of the process that are most labour intensive and prone to error.
“The amount of work to do on an ongoing basis to demonstrate to regulators that models are operating properly is overwhelming, and very manually intensive. If machine learning can help develop a heat map to show where managers should be placing their attention and what models need to be refined, that would help focus their efforts,” says Ed Young, senior director in capital planning and stress testing at Moody’s Analytics in New York.
ML algorithms allow computer programs to make decisions and predictions from unseen data inputs. Two principal subsets exist: ‘supervised learning’ algorithms, which are taught through example datasets to map certain inputs to outputs, and ‘unsupervised learning’ algorithms, which are presented with datasets and left to discover patterns on their own, without human guidance.
By training a machine to process repetitive parts of validation we can focus our attention on the higher and more complex models responsible for the biggest exposures
Lourenco Miranda, Societe Generale
French dealer Natixis is one firm getting to grips with the possibilities of unsupervised learning algorithms in model validation. For the past six months, its equity derivatives business has utilised this type of ML to detect anomalous projections generated by its stress-testing models. Every night, these models produce over 3 million computations to inform regulatory, internal capital allocations and limit monitoring. A small fraction of these are incorrect, knocked out of the normal distribution of results by a quirk of the computation cycle or faulty data inputs.
“This ML algorithm helps us to determine which results are suspicious, so that we can analyse them and automatically replay the computation in case it was caused by a transient error. All results are scanned and evaluated by the ML regardless of the final use of the projections, whether for regulatory or trading purposes,” says José Luu, head of IT derivatives and pricing at Natixis in Paris.
This use of ML hands validators a valuable tool for the ongoing monitoring of their stress-testing models, as it can help determine whether they are performing within acceptable tolerances or drifting from their original purpose.
Nomura is another dealer that has been using a form of machine learning as part of its model risk management function, specifically to police model use – something it has been doing for the past six years.
Slava Obraztsov, global head of model validation at Nomura in London, says: “We record model restrictions in a machine-readable format to support their automated monitoring. What happens is we validate a model and impose restrictions on what products it can be used for. The monitoring is run across all trading portfolios on a periodic basis to check that no position has been booked on a model in breach of its restrictions. This is to ensure that products are not booked on models that may not properly capture some product features and dynamics.”
Other dealers have been exploring and implementing ML in relation to operational risk and anti-money laundering (AML) modelling, says Shaheen Dil, New York-based managing director at consultancy Protiviti.

“The reason is that these are the two areas of risk where the datasets are enormous. In the case of operational risk there are no standard acceptable models that have been in place for a long time, so banks have had to build their own from scratch. For AML, many banks are purchasing vendor models, but these are by and large ‘black boxes’ to the banks,” says Dil.
Valid arguments
Validation appears to be the area with the most to gain from embracing ML, as it comprises a number of tasks that could benefit from automation. SR 11-7 pushes banks to conduct periodic reviews “at least annually” of all models to ensure they are working as intended, covering everything from their “conceptual soundness” – essentially their design and construction – to their sensitivity to small changes in data inputs.
Ongoing monitoring is also expected: internal and external data should be checked and re-checked, computer code subjected to “rigorous quality and change control procedures”, reports generated from model outputs reviewed, and the models themselves benchmarked to estimates from internal “challenger models” or third-party calculation engines. All this must also be documented in sufficient detail such that an independent third party – an auditor, for instance – could make sense of it.
The Trim also advocates an annual validation cycle at a similar level of granularity. Right now, this is beyond the capabilities of some banks.
“We do not comply completely; we do not review all the models every year,” says the head of model validation at the regional European bank. “We don’t have the means. I am not afraid about the utility of our models, but the ECB expects us to have a formal, standardised process and right now our function is decentralised and not globally co-ordinated.”
Data quality is a particular focus of the Trim. For example, in the context of the internal ratings-based approach for credit risk capital, the ECB expects input data for these models to be subject to periodic cleansing analyses, as well as benchmarked against external up-to-date credit data sources.
People look at model risk management as a cost. I always remind people: look at the London Whale which cost JP Morgan $6 billion
Head of model risk at an international bank
Dealers say ML algorithms can monitor and identify patterns in data faster and more efficiently than hard-coded programs and identify missing inputs that, if located, could upgrade a model’s performance.
“If you go to the banking book, we have got a lot of products that have different patterns, different structures. We currently use econometric models for the prediction of the data. ML classification can be used and then the production of the model can be done correctly. The projection of the risk by ML techniques is also much more accurate and robust,” says Mostafa Mostafavi, London-based vice-president of risk and quantitative analysis at Credit Suisse.
Take the example of validating an op risk model that measures losses from fraud. An ML algorithm could examine all the inputs that go into predicting fraud losses and identify missing pieces of information that, if added to the statistical model, could improve its performance, suggests Dallas-based Chris Siddons, senior director of regulatory and compliance software at LexisNexis Risk Solutions.
ML could also be harnessed for model benchmarking purposes. “Many larger banks need to build challenger models to test the primary models for accuracy and robustness. ML algorithms can function as challenger models or for checking specific aspects of the primary models’ predictive power,” says Marco Vettori, a partner at McKinsey in Milan.
The consultancy is also tipping ML to advance into the building of primary models themselves – something at least one dealer is already getting to grips with: “We use ML to build primary models, including our CCAR [Comprehensive Capital Analysis and Review] models,” says the head of risk analytics at a second international bank. “ML is used to cluster and segment data to construct each model as well as for model calibration. This year these technologies have been much more heavily used in house.”
Fear of the unknown
Yet plenty stands in the way of a full-scale march of the machines. First, certain banks are nervy about the attitude regulators will take towards these complex technologies. Second, banks themselves may be struggling to understand the biases implicit in these ML models and substantiate them to their own satisfaction.
As these are learning algorithms, it’s hard for a model risk manager to prove how an ML technology reaches its conclusions. If something’s hard to prove, it’s hard to document – something essential to the model risk management process.
“Regulators require banks to explain why a decision was made and ML doesn’t allow for that. There are some efforts to tag ML outputs with explanations, but it’s not a natural part of the process,” says Ranko Mosic, a Toronto-based big data consultant who has worked with Bank of America and State Street, among others.
Yet Credit Suisse’s Mostafavi believes regulators aren’t as scared of ML as these consultants suggest. “Because they are not simple, people think they are not transparent, but I think they are good tools. This is a growing area; ML software will be used frequently in the future,” he says.
Grafting ML technologies on to legacy systems is no walk in the park for the dealers themselves – another reason wholesale adoption does not yet appear to be on the cards.
“A problem in large corporations is the sheer complexity of ML. Firms have enough trouble with their existing processes – extract, transform, load, solving data silo problems, and modelling. With ML it’s not as simple as rolling out a new packaged or in-house built data system. Some firms don’t know where to start,” says Mosic.
Nonetheless, investing in better model risk management is undoubtedly worth the expense when considering the alternatives, many point out.
“People look at model risk management as a cost. I always remind people: look at the losses the banks had in 2008, look at the London Whale which cost JP Morgan $6 billion. When people have that kind of loss, model control which may cost $30–50 million a year becomes small,” says the head of model risk at the first international bank.
New model army
The challenges facing banks’ model risk managers are stiff. The Federal Reserve’s SR 11-7 has become the gold standard for model risk management since its unveiling in 2011 – but despite being in effect for six years, few banks are adhering to it to the letter. Dealers have previously reported that the guidance impelled a threefold increase in the number of models requiring validation and a vast expansion of staff assigned to the model risk function.
Dealers say foreign regulators have effectively cribbed the Fed’s guidelines for their own supervisory standards, extending SR 11-7’s reach far beyond US shores. For instance, the principles set out in the Bank of England’s recently issued letter to British banks and building societies on model governance represent “a concise and mature representation of the SR 11-7 text”, according to the head of model risk at one international bank.
The themes addressed by the European Central Bank’s Targeted Review of Internal Models (Trim) programme are also “very similar” to SR 11-7, says Konstantina Armata, head of global model validation and governance at Deutsche Bank in London.
“These are big exercises; we are talking about weeks and weeks of examinations and hundreds of requests. It’s a big process for us,” she says.
SR 11-7 sets out compliance requirements across four broad categories of model risk management: development and implementation, use, validation, and governance. To manage these simultaneously, most banks have consolidated their model risk management functions under one roof.
Other models we are leaving to one side, such as our economic capital models, as we really need to focus on where there is most outside pressure from regulators
Head of model validation at a regional European bank
This organisational overhaul was only the first step on the journey to compliance, however. Dealers may have made progress building model inventories, assigning model owners and setting up independent validation processes, but there is still much work to be done getting the models themselves up to scratch – and verifying their effectiveness.
“In the case of the biggest challenges for SR 11-7, I would say that model preparedness is number one. Despite the guidance being now six years old, there is still a lot to be done in terms of preparedness: model documentation, internal control testing and documentation of the results, continued monitoring of the impact of model limitations, and so forth,” says Lourenco Miranda, head of model risk management for Americas at Societe Generale.
As for the Trim, the ECB’s expectations appear set to dwarf the capabilities of smaller dealers.
“We have to put in place a series of frameworks that we do not have yet but that regulators clearly expect the banks to put in place,” the head of model validation at a regional European bank says. “With all the requirements and work that has to be done, I don’t do anything outside the scope of internal capital models and valuation models. Other models we are leaving to one side, such as our economic capital models, as we really need to focus on where there is most outside pressure from regulators.”
Only users who have a paid subscription or are part of a corporate subscription are able to print or copy content.
To access these options, along with all other subscription benefits, please contact info@risk.net or view our subscription options here: http://subscriptions.risk.net/subscribe
You are currently unable to print this content. Please contact info@risk.net to find out more.
You are currently unable to copy this content. Please contact info@risk.net to find out more.
Copyright Infopro Digital Limited. All rights reserved.
As outlined in our terms and conditions, https://www.infopro-digital.com/terms-and-conditions/subscriptions/ (point 2.4), printing is limited to a single copy.
If you would like to purchase additional rights please email info@risk.net
Copyright Infopro Digital Limited. All rights reserved.
You may share this content using our article tools. As outlined in our terms and conditions, https://www.infopro-digital.com/terms-and-conditions/subscriptions/ (clause 2.4), an Authorised User may only make one copy of the materials for their own personal use. You must also comply with the restrictions in clause 2.5.
If you would like to purchase additional rights please email info@risk.net
More on Risk management
Diversification of LDI liquidity buffers sparks debate
Funds using credit assets to top up collateral waterfall, but some risk managers are sceptical
Transforming stress-testing with AI
Firms can update their stress-testing capability by harnessing automated scenario generation, says fintech advocate
Basel stops short on wrong-way risk
New guidelines a step in right direction, but experts warn they won’t prevent another Archegos
On resilience risk, banks prepare to let the bad times roll
Lenders bolster first-line teams and upskill boards as compliance with new rules bites
Complex EU active account reporting could drive trades out of UK
Draft Emir rules might not force large volumes to move to EU, but will make compliance difficult
Strategies for navigating market volatility in the post-US election landscape
This article examines the key themes of a recent webinar, sponsored by S&P Global Market Intelligence, on market volatility following the US election, including inflation risks, commodities, geopolitical uncertainty, ESG considerations and the role of…
Risk.net’s top 10 investment risks for 2025
Fresh concerns this year include a trade war, a stock market crash and growing social discord
For banks, change risk is inevitable; managing it, optional
Regional bank survey shows steady growth of dedicated change risk functions and adoption of leading indicators