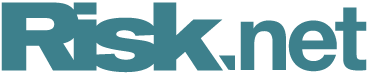
This article was paid for by a contributing third party.More Information.

Staying afloat in the data deluge

Oracle was named winner of the risk data repository and data management, and enterprise-wide operational risk management categories in the 2016 Risk Technology Rankings. Ambreesh Khanna, group vice-president and general manager financial services analytical applications, explains how regulatory deadlines, infrastructure redesigns and the sheer volume of risk data are making new demands and applying new pressure to banks and their technology systems.
What are the major challenges facing banks today in managing risk data for regulatory compliance and business activities?
Ambreesh Khanna: Banks are continuing to evolve their risk data management processes and are now looking at ways to monetise the data. However, they continue to face challenges in streamlining their risk data aggregation process. Three key challenges being faced are:
- Risk data exists in different source systems and in multiple formats: sourcing it into a common repository in a consistent manner is one of the largest challenges for banks.
- The second major challenge concerns data quality. There is inconsistency in availability of data across product types and lines of business, and often source data is incorrectly captured. This leads to data governance and reconciliation issues. Banks have been working on this problem and are now starting to get a grip on it.
- The third challenge relates to consistency in results data across multiple analytical streams. Banks use multiple technology solutions, each of which may vary in terms of metadata or calculations. This leads to inconsistency in results, which becomes a major challenge given the increasing cross-functional nature of regulatory reporting.
Can banks rely on legacy systems and systems upgrades to meet the challenges, or do they need a new approach?

Ambreesh Khanna: The sheer volume of risk data required for regulatory and internal risk management has increased dramatically over the past decade. Banks now need granular account-level data across banking and trading books for most of their risk models, for regulatory and even for statutory reporting. Added to that, regulators are asking banks to maintain historical records going back many years with full traceability and auditability. Most legacy systems have not been designed to handle such high volumes and neither have they been designed to meet the data governance needs of today.
Therefore, systems upgrades of legacy solutions are of limited use and end up highly expensive and inefficient in the long run. Banks need to use this opportunity given by the regulatory focus on risk data to deploy cutting-edge solutions that are built with deep risk domain expertise, have strong data governance capabilities and are able to handle large volumes. In addition, banks must focus on the ability of their data management solutions to leverage new technologies such as blockchain and machine learning to maximise the value derived from their data.
How should banks design their data management architecture to most effectively meet the current challenges?
Ambreesh Khanna: Financial institutions need to focus on four key principles in designing their data management architecture:
- A common data foundation across risk and finance designed to hold granular data with traceability to source systems.
- Strong data governance that includes data quality and reconciliation capabilities, and data access controls.
- Flexibility in being able to evolve quickly in sync with newer regulatory and business requirements.
- The ability to leverage cutting-edge technologies such as machine learning that enable the organisation to derive business benefits from the data.
What are the key technologies that will support effective risk data management?
Ambreesh Khanna: Computations for risk management – value-at-risk calculations, valuations, price simulations, backtesting, capital allocation optimisation and default modelling – have all traditionally relied on expensive grid computing infrastructure backed by high-performance relational data stores/marts. With the growing adoption of open-source in-memory general-purpose processing clusters such as Apache Spark and Apache Flink, many of the expensive proprietary grids may be replaced with general-purpose big data clusters, which have the added advantage of data local processing. Risk and finance data that has been aggregated by the institution in a data lake can now be readily used for computational processing right in the data lake. Moreover, these open-source grids also now support massively parallel matrix computations and convex optimisation routines, further enabling efficient computation of risk measures.
Given that IT budgets are tightly constrained these days, what steps can banks take to ensure that risk data management is optimised and cost-effective?
Ambreesh Khanna: Banks need to look beyond cost and process efficiencies and start to focus on monetising the data. Easy access to reconciled clean data can help lines of business in improving their risk models, which, in turn, can improve margins through better capital allocations and risk-based pricing.
Sponsored content
Copyright Infopro Digital Limited. All rights reserved.
As outlined in our terms and conditions, https://www.infopro-digital.com/terms-and-conditions/subscriptions/ (point 2.4), printing is limited to a single copy.
If you would like to purchase additional rights please email info@risk.net
Copyright Infopro Digital Limited. All rights reserved.
You may share this content using our article tools. As outlined in our terms and conditions, https://www.infopro-digital.com/terms-and-conditions/subscriptions/ (clause 2.4), an Authorised User may only make one copy of the materials for their own personal use. You must also comply with the restrictions in clause 2.5.
If you would like to purchase additional rights please email info@risk.net