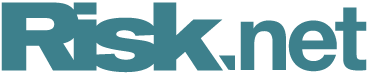
When it comes to correlation, cleaning is a chore that pays
Recent trends in research may help firms obtain reliable correlations from limited data
Correlations are some of the most basic pieces of information that help investors understand market moves and build portfolios. However, they lead to some of the most unwieldy estimation problems faced by portfolio managers today. As with many other issues in quantitative finance, it all comes down to a lack of data.
As the number of assets in a portfolio increases, building reliable correlations becomes difficult. For a large portfolio of 500 assets – for example, one that mirrors the S&P 500 index – one would need many years of data to obtain reliable correlations.
"When you try to estimate very large objects, because the object is very large, it would need super-large data sets, going back to the past centuries to be able to pin down the correlation matrix," says Jean-Philippe Bouchaud, chairman and chief scientist at Capital Fund Management in Paris. "[This] obviously makes no sense at all, because over centuries you would expect a lot of things to change."
Modelling using a limited amount of data means one ends up with a huge amount of noise in the estimated correlation matrix, which can lead to massive estimation errors. Portfolio managers have been attempting to tackle the problem for decades, and have tried to solve it through tweaks to the empirical correlation matrix built from observable data. However, that has resulted in both computational challenges and errors in the numbers produced.
Research on 'cleaning' correlation matrices has been picking up in recent times, with many papers published on the topic in the last five years. Most recently, Bouchaud, along with co-authors Joel Bun, a PhD student at Université Paris-Saclay at the Léonard de Vinci Pôle Universitaire, and Marc Potters, co-chief executive and head of research at Capital Fund Management, analysed a number of correlation matrix cleaning techniques and recommended the one they believe performs the best: a technique proposed by quants Olivier Ledoit and Sandrine Péché in 2011.
Its working involves what the authors call a "mathematical miracle", which starts with the empirical correlation matrix of the portfolio and aims to get as close as possible to the true correlation matrix – that is, one without the errors and which truly represents the relationship between assets.
Any correlation matrix can be decomposed into characteristic entities called Eigen values and Eigen vectors. Assuming one wouldn't know the direction of the true Eigen vectors – that is, by keeping them the same as the ones from the empirical correlation matrix – one can still tweak the Eigen values. In their 2011 paper, Ledoit and Péché calculated a formula that gives the overlap between the Eigen vectors of the empirical matrix and the true correlation matrix. This, in turn, gives the Eigen values of the true correlation matrix.
"The miracle comes from the fact that with this explicit formula, in the end, you are able to get a clean formula for the Eigen values which does not require you to know what you try to measure – that is, the true correlation matrix," explains Bouchaud. "As an intermediate step, you think you need this unknown correlation matrix to get the formula, but the miracle is that it cancels out because we are working with very large objects with large matrices. So there is this extension of the law of very large numbers."
Bouchaud and his co-authors tested the performance of their own extension of the Ledoit-Péché method, which accounts for a wider range of Eigen values than the original, against four other commonly used methods. That includes one of the more simple and commonly-used methods – the shrinkage technique proposed by Ledoit and Michael Wolf in 2003, which tries to pull the extreme coefficients in the matrix towards more central values. Even this method was found to be outperformed by the Ledoit-Péché extension, which gave the lowest realised risk among all five methods considered.
Using this method means that, as a rule of thumb, one would require only twice the number of data points as there are assets to get a reasonably good correlation matrix, Bouchaud argues.
The work raises the question of why it has taken so long for the industry to fix something as straightforward and fundamental as building a reliable correlation matrix out of limited asset returns data. Due to the challenges involved, others have settled for filling in missing returns using certain assumptions or data from time points that don't really match for different assets. The resulting correlation matrices are considered invalid because they do not satisfy an important property called positive semi-definiteness.
"The issue is we don't have deep theory behind these things in terms of what we should do when we are violating some of the basic assumptions in building these portfolios," says Eliott Noma, a managing director at Garrett Asset Management in New York.
By analysing existing methods for cleaning correlations and their drawbacks, Bouchaud and his co-authors are helping to bring this fundamental issue back into focus. That could bring lasting benefits for portfolio managers.
Only users who have a paid subscription or are part of a corporate subscription are able to print or copy content.
To access these options, along with all other subscription benefits, please contact info@risk.net or view our subscription options here: http://subscriptions.risk.net/subscribe
You are currently unable to print this content. Please contact info@risk.net to find out more.
You are currently unable to copy this content. Please contact info@risk.net to find out more.
Copyright Infopro Digital Limited. All rights reserved.
As outlined in our terms and conditions, https://www.infopro-digital.com/terms-and-conditions/subscriptions/ (point 2.4), printing is limited to a single copy.
If you would like to purchase additional rights please email info@risk.net
Copyright Infopro Digital Limited. All rights reserved.
You may share this content using our article tools. As outlined in our terms and conditions, https://www.infopro-digital.com/terms-and-conditions/subscriptions/ (clause 2.4), an Authorised User may only make one copy of the materials for their own personal use. You must also comply with the restrictions in clause 2.5.
If you would like to purchase additional rights please email info@risk.net
More on Risk management
Rising systemic risk demands a new risk management paradigm
Reinsurers need insurance-linked securities to share burden of climate-related catastrophic risk
ECB removes need for governing council to approve CCP facility
New “automatic” facility will require safeguards that are “still being implemented”, bank says
Dodging a steamroller: how the basis trade survived the tariff tantrum
Higher margins, rising yields and stable repo funding helped avert another disruptive blow-up
BoE plans to link system-wide and individual stress tests
Meanwhile, ECB wants to broaden system-wide stress models to include central counterparties
Cyber insurance costs expected to rise as loss ratios worsen
Recent ransomware and tech failure events could feed through into higher premiums this year
The WWR in the tail: a Monte Carlo framework for CCR stress testing
A methodology to compute stressed exposures based on a Gaussian copula and mixture distributions is introduced
Repo clearing rule could raise SOFR volatility – OFR analysts
Analysis of 2022 data finds large divergence in tail rates but no change in median
OCC’s security chief on generative AI with guardrails
Clearing house looks to scale technology across risk and data operations – but safety is still the watchword