Journal of Operational Risk
ISSN:
1744-6740 (print)
1755-2710 (online)
Editor-in-chief: Marcelo Cruz
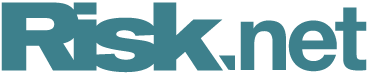
Bayesian operational risk models
Silvia Figini, Lijun Gao and Paolo Giudici
Abstract
ABSTRACT
Operational risk is hard to quantify, due to the presence of heavy tailed loss distributions. Extreme value distributions, used in this context, are very sensitive to the data, and this is a problem in the presence of rare loss data. Self-assessment questionnaires for risk, if properly structured, may provide prior opinions that can be used, in a Bayesian analysis, to better estimate operational risks. In this paper we propose a methodology to frame risk self-assessment data into suitable prior distributions that, updated with the observed loss data, can produce posterior distributions from which accurate operational risk measures, such as value-at-risk (VaR), can be obtained. We test our proposed model on a real database, made up of internal loss data and risk self-assessment questionnaires of an anonymous commercial bank. Our results show that the proposed Bayesian models perform better with respect to classical extreme value models, leading to a smaller quantification of the VaR required to cover unexpected losses.
Copyright Infopro Digital Limited. All rights reserved.
You may share this content using our article tools. Printing this content is for the sole use of the Authorised User (named subscriber), as outlined in our terms and conditions - https://www.infopro-insight.com/terms-conditions/insight-subscriptions/
If you would like to purchase additional rights please email info@risk.net
Copyright Infopro Digital Limited. All rights reserved.
You may share this content using our article tools. Copying this content is for the sole use of the Authorised User (named subscriber), as outlined in our terms and conditions - https://www.infopro-insight.com/terms-conditions/insight-subscriptions/
If you would like to purchase additional rights please email info@risk.net