Journal of Risk
ISSN:
1465-1211 (print)
1755-2842 (online)
Editor-in-chief: Farid AitSahlia
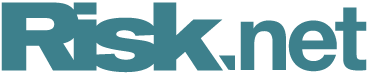
Need to know
- We propose a kernel-based estimator of spectral risk measure.
- The proposed estimator is strongly consistent and asymptotically normal.
- We study the finite sample performance through simulations and carried-out a backtesting exercise.
- Based on our simulation study we estimate the exponential SRM of four future indices namely Nikkei 225, Dax, FTSE 100 and Hang Seng and discuss the use of SRM in setting initial margin requirements of clearinghouses.
Abstract
Spectral risk measures (SRMs) belong to the family of coherent risk measures. A natural estimator for the class of SRMs takes the form of L-statistics. Various authors have studied and derived the asymptotic properties of the empirical estimator of SRMs; we propose a kernel-based estimator. We investigate the large-sample properties of general L-statistics based on independent and identically distributed observations and dependent observations and apply them to our estimator. We prove that it is strongly consistent and asymptotically normal. Using Monte Carlo simulation, we compare the finite-sample performance of our proposed kernel estimator with that of several existing estimators for different SRMs and observe that our proposed kernel estimator outperforms all the other estimators. Based on our simulation study, we estimate the exponential SRM for heavily traded futures (that is, the Nikkei 225, Deutscher Aktienindex, Financial Times Stock Exchange 100 and Hang Seng futures). We also discuss the use of SRMs in setting the initial-margin requirements of clearinghouses. Finally, we perform an SRM backtesting exercise.
Copyright Infopro Digital Limited. All rights reserved.
As outlined in our terms and conditions, https://www.infopro-digital.com/terms-and-conditions/subscriptions/ (point 2.4), printing is limited to a single copy.
If you would like to purchase additional rights please email info@risk.net
Copyright Infopro Digital Limited. All rights reserved.
You may share this content using our article tools. As outlined in our terms and conditions, https://www.infopro-digital.com/terms-and-conditions/subscriptions/ (clause 2.4), an Authorised User may only make one copy of the materials for their own personal use. You must also comply with the restrictions in clause 2.5.
If you would like to purchase additional rights please email info@risk.net